Thus far, we have used statistical methods to reach conclusions by seeing how compatible the observations were with the null hypothesis that the treatment had no effect. When the data were unlikely to occur if this null hypothesis was true, we rejected it and concluded that the treatment had an effect. We used a test statistic (F, t, z, or χ2) to quantify the difference between the actual observations and those we would expect if the null hypothesis of no effect were true. We concluded that the treatment had an effect if the value of this test statistic was bigger than 95% of the values that would occur if the treatment had no effect. When this is so, it is common for medical investigators to report a statistically significant effect. On the other hand, when the test statistic is not big enough to reject the hypothesis of no treatment effect, investigators often report no statistically significant difference and then discuss their results as if they had proven that the treatment had no effect. All they really did was fail to demonstrate that it did have an effect. The distinction between positively demonstrating that a treatment had no effect and failing to demonstrate that it did have an effect is subtle but very important, especially in the light of the small numbers of subjects included in most clinical studies.*
As already mentioned in our discussion of the t test, the ability to detect a treatment effect with a given level of confidence depends on the size of the treatment effect, the variability within the population, and the size of the samples used in the study. Just as bigger samples make it more likely that you will be able to detect an effect, smaller sample sizes make it harder. In practical terms, this fact means that studies of therapies that involve only a few subjects and fail to reject the null hypothesis of no treatment effect may arrive at this result because the statistical procedures lacked the power to detect the effect because of a too small sample size, even though the treatment did have an effect. Conversely, considerations of the power of a test permit you to compute the sample size needed to detect a treatment effect of given size that you believe is present.
* This problem is particularly encountered in small clinical studies in which there are no “failures” in the treatment group. This situation often leads to overly optimistic assessments of therapeutic efficacy. See Hanley JA, Lippman-Hand A. If nothing goes wrong, is everything all right? Interpreting zero numerators. JAMA. 1983;249:1743–1745.
Now, we make a radical departure from everything that has preceded: we assume that the treatment does have an effect.
Figure 6-1 shows the same population of people we studied in Figure 4-3 except that this time the drug given to increase daily urine production works. It increases the average urine production for members of this population from 1200 to 1400 mL/day. Figure 6-1A shows the distribution of daily urine production for all 200 members of the population in the control (placebo) group, and Figure 6-1B shows the distribution of urine production for all 200 members of the population in the diuretic group.
Figure 6-1.

Daily urine production in a population of 200 people while they are taking a placebo and while they are taking an effective diuretic that increases urine production by 200 mL/day on the average. Panels A and B show the specific individuals selected at random for study. Panel C shows the results as they would appear to the investigator. t = 2.447 for these observations. Since the critical value of t for P < .05 with 2(10 − 1) = 18 degrees of freedom is 2.101, the investigator would probably report that the diuretic was effective.
More precisely, the population of people taking the placebo consist of a normally distributed population with mean μpla = 1200 mL/day and the population of people taking the drug consist of a normally distributed population with a mean of μdr = 1400 mL/day. Both populations have the same standard deviation, σ = 200 mL/day.
Of course, an investigator cannot observe all members of the population, so he or she selects two groups of 10 people at random, gives one group the diuretic and the other a placebo, and measures their daily urine production. Figure 6-1C shows what the investigator would see. The people receiving a placebo produce an average of 1180 mL/day, and those receiving the drug produce an average of 1400 mL/day. The standard deviations of these two samples are 144 and 245 mL/day, respectively. The pooled estimate of the population variance is
which exceeds 2.101, the value that defines the most extreme 5% of possible values of the t test statistic when the two samples are drawn from the same population. (There are ν = ndr + npla − 2 = 10 + 10 − 2 = 18 degrees of freedom.) The investigator would conclude that the observations are not consistent with the assumption that two samples came from the same population and report that the drug increased urine production. And he or she would be right.
Of course, there is nothing special about the two random samples of people selected for the experiment. Figure 6-2 shows two more groups of people selected at random to test the drug, together with the results as they would appear to the investigator. In this case, the mean urine production is 1216 mL/day for the people given the placebo and 1368 mL/day for the people taking the drug. The standard deviations of urine production in the two samples are 97 and 263 mL/day, respectively, so the pooled estimate of the variance is 1/2(972 + 2632) = 1982. The value of t associated with these observations is
which is less than 2.101. Had the investigator selected these two groups of people for testing, he or she would not have obtained a value of t large enough to reject the hypothesis that the drug had no effect and probably reported “no significant difference.” If the investigator went on to conclude that the drug had no effect, he or she would be wrong.
Figure 6-2.

There is nothing special about the two random samples shown in Figure 6-1. This illustration shows another random sample of two groups of 10 people each selected at random to test the diuretic (A and B) and the results as they would appear to the investigator (C). The value of t associated with these observations is only 1.71, not great enough to reject the hypothesis of no drug effect with P < 0.05, that is, α = 0.05. If the investigator reported the drug had no effect, he or she would be wrong.
Notice that this is a different type of error from that discussed in Chapters 3, 4, 5. In the earlier chapters, we were concerned with rejecting the hypothesis of no effect when it was true. Now we are concerned with not rejecting it when it is not true. This situation is called a Type II error or β error.
Just as we could repeat this experiment more than 1027 times when the drug had no effect to obtain the distribution of possible values of t (compare with the discussion of Fig. 4-4), we can do the same thing when the drug does have an effect. Figure 6-3 shows the results of 200 such experiments; 111 out of the resulting values of t fall at or above 2.101, the value we used to define a “big” t. Put another way, if we wish to keep the P value at or below 5%, there is a 111/200 = 56% chance of concluding that the diuretic increases urine output when average urine output actually increases by 200 mL/day. We say the power of the test is .56. The power quantifies the chance of detecting a real difference of a given size.
Figure 6-3.

(A) The distribution of values of the t test statistic computed from 200 experiments that consisted of drawing two samples of size 10 each from a single population; this is the distribution we would expect if the diuretic had no effect on urine production is centered on zero. (compare with Fig. 4-4A.) (B) The distribution of t values from 200 experiments in which the drug increased average urine production by 200 mL/day. t = 2.1 defines the most extreme 5% of the possible values of t when the drug has no effect; 111 of the 200 values of t we would expect to observe from our data fall above this point when the drug increases urine production by 200 mL/day. Therefore, there is a 56% chance that we will conclude that the drug actually increases urine production from our experiment.
Alternatively, we could concentrate on the 89 of the 200 experiments that produced t values below 2.101, in which case we would fail to reject the hypothesis that the treatment had no effect and be wrong. Thus, there is an 89/200 = 44% = .44 chance of continuing to accept the hypothesis of no effect when the drug really increased urine production by 200 mL/day on the average.
Now we have isolated the two different ways the random-sampling process can lead to erroneous conclusions. These two types of errors are analogous to the false-positive and false-negative results one obtains from diagnostic tests. Before this chapter we concentrated on controlling the likelihood of making a false-positive error, that is, concluding that a treatment has an effect when it really does not. In keeping with tradition, we have generally sought to keep the chances of making such an error below 5%; of course, we could arbitrarily select any cutoff value we wanted at which to declare the test statistic “big.” Statisticians denote the maximum acceptable risk of this error by α, the Greek letter alpha. If we reject the hypothesis of no effect whenever P <.05, α = 0.05 or 5%. If we actually obtain data that lead us to reject the null hypothesis of no effect when the null hypothesis of no effect is true, statisticians say that we have made a Type I error. All this logic is relatively straightforward because we have specified how much we believe the treatment affects the variable of interest, that is, not at all.
What about the other side of the coin, the chance of making a false-negative conclusion and not reporting an effect when one exists? Statisticians denote the chance of erroneously accepting the hypothesis of no effect by β, the Greek letter beta. The chance of detecting a true positive, that is, reporting a statistically significant difference when the treatment really produces an effect, is 1 − β. The power of the test that we discussed earlier is equal to 1 − β. For example, if a test has power equal to .56, there is a 56% chance of actually reporting a statistically significant effect when one is really present. Table 6-1 summarizes these definitions.
Actual Situation | ||
---|---|---|
Conclude From Observations | Treatment Has an Effect | Treatment Has No Effect |
Treatment has an effect | True positive | False positive |
Correct conclusion 1 — β | Type I error α | |
Treatment has no effect | False negative | True negative |
Type II error β | Correct conclusion 1 − α |
So far we have developed procedures for estimating and controlling the Type I, or α, error. Now we turn our attention to keeping the Type II, or β, error as small as possible. In other words, we want the power to be as high as possible. In theory, this problem is not very different from the one we already solved with one important exception. Since the treatment has an effect, the size of this effect influences how easy it is to detect. Large effects are easier to detect than small ones. To estimate the power of a test, you need to specify how small an effect is worth detecting.
Just as with false positives and false negatives in diagnostic testing, the Type I and Type II errors are intertwined. As you require stronger evidence before reporting that a treatment has an effect, that is, make α smaller, you also increase the chance of missing a true effect, that is, make β bigger or power smaller. The only way to reduce both α and β simultaneously is to increase the sample size, because with a larger sample you can be more confident in your decision, whatever it is.
- The risk of error you will tolerate when rejecting the hypothesis of no treatment effect.
- The size of the difference you wish to detect relative to the amount of variability in the populations.
- The sample size.
Figure 6-3 showed the complementary nature of the maximum size of the Type I error α and the power of the test. The acceptable risk of erroneously rejecting the hypothesis of no effect, α, determines the critical value of the test statistic above which you will report that the treatment had an effect, P < α. (We have usually taken α = 0.05.) This critical value is defined from the distribution of the test statistic for all possible experiments with a specific sample size given that the treatment had no effect. The power is the proportion of possible values of the test statistic that fall above this cutoff value given that the treatment had a specified effect (here a 200 mL/day increase in urine production). Changing α, or the P value required to reject the hypothesis of no difference, moves this cutoff point, affecting the power of the test.
Figure 6-4 illustrates this point further. Figure 6-4A essentially reproduces Figure 6-3 except that it depicts the distribution of t values for all 1027 possible experiments involving two groups of 10 people as a continuous distribution. The top part, copied from Figure 4-4D, shows the distribution of possible t values (with ν = 10 + 10 − 2 = 18 degrees of freedom) that would occur if the drug did not affect urine production. Suppose we require P < .05 before we are willing to assert that the observations were unlikely to have arisen from random sampling rather than the effect of the drug. According to the table of critical values of the t distribution (see Table 4-1), for ν = 18 degrees of freedom, 2.101 is the (two-tail) critical value that defines the most extreme 5% of possible values of the t test statistic if the null hypothesis of no effect of the diuretic on urine production is true. In other words, when we make α = 0.05, in which case −2.101 and +2.101 delimit the most extreme 5% of all possible t values we would expect to observe if the diuretic did not affect urine production.
Figure 6-4.

(A) The top panel shows the distribution of the t test statistic that would occur if the null hypothesis was true and the diuretic did not affect urine production. The distribution is centered on 0 (because the diuretic has no effect on urine production) and, from Table 4-1, t = +2.101 (and −2.101) define the (two-tail) 5% most extreme values of the t test statistic that would be expected to occur by chance if the drug had no effect. The second panel shows the actual distribution of the t test statistic that occurs when the diuretic increases urine output by 200 mL/day; the distribution of t values is shifted to the right, so the distribution is now centered on 2.236. The critical value of 2.101 is −.135 below 2.236, the center of this shifted distribution. From Table 6-2, .56 of the possible t values fall in the one-tail above −.135, so we conclude that the power of a t test to detect a 200 mL/day increase in urine production is 56%. (The power also includes the portion of the t distribution in the lower tail below −2.101, but because this area is so small we will ignore it.) (B) If we require more evidence before rejecting the null hypothesis of no difference by reducing α to 0.01, the critical value of t that must be exceeded to reject the null hypothesis increases to 2.878 (and −2.878). Since the effect of the diuretic is unchanged, the actual distribution of t remains centered on 2.236; the critical value of 2.878 is .642 above 2.236, the center of the actual t distribution. From Table 6-2, .27 of the possible t values fall in the tail above .642, so the power of the test drops to 27%.
Probability of Larger Value (Upper Tail) | ||||||||||
---|---|---|---|---|---|---|---|---|---|---|
.995 | .99 | .98 | .975 | .95 | .90 | .85 | .80 | .70 | .60 | |
Probability of Smaller Value (Lower Tail) | ||||||||||
ν | .005 | .01 | .02 | .025 | .05 | .10 | .15 | .20 | .30 | .40 |
2 | −9.925 | −6.965 | −4.849 | −4.303 | −2.920 | −1.886 | −1.386 | −1.061 | −0.617 | −0.289 |
4 | −4.604 | −3.747 | −2.999 | −2.776 | −2.132 | −1.533 | −1.190 | −0.941 | −0.569 | −0.271 |
6 | −3.707 | −3.143 | −2.612 | −2.447 | −1.943 | −1.440 | −1.134 | −0.906 | −0.553 | −0.265 |
8 | −3.355 | −2.896 | −2.449 | −2.306 | −1.860 | −1.397 | −1.108 | −0.889 | −0.546 | −0.262 |
10 | −3.169 | −2.764 | −2.359 | −2.228 | −1.812 | −1.372 | −1.093 | −0.879 | −0.542 | −0.260 |
12 | −3.055 | −2.681 | −2.303 | −2.179 | −1.782 | −1.356 | −1.083 | −0.873 | −0.539 | −0.259 |
14 | −2.977 | −2.624 | −2.264 | −2.145 | −1.761 | −1.345 | −1.076 | −0.868 | −0.537 | −0.258 |
16 | −2.921 | −2.583 | −2.235 | −2.120 | −1.746 | −1.337 | −1.071 | −0.865 | −0.535 | −0.258 |
18 | −2.878 | −2.552 | −2.214 | −2.101 | −1.734 | −1.330 | −1.067 | −0.862 | −0.534 | −0.257 |
20 | −2.845 | −2.528 | −2.197 | −2.086 | −1.725 | −1.325 | −1.064 | −0.860 | −0.533 | −0.257 |
25 | −2.787 | −2.485 | −2.167 | −2.060 | −1.708 | −1.316 | −1.058 | −0.856 | −0.531 | −0.256 |
30 | −2.750 | −2.457 | −2.147 | −2.042 | −1.697 | −1.310 | −1.055 | −0.854 | −0.530 | −0.256 |
35 | −2.724 | −2.438 | −2.133 | −2.030 | −1.690 | −1.306 | −1.052 | −0.852 | −0.529 | −0.255 |
40 | −2.704 | −2.423 | −2.123 | −2.021 | −1.684 | −1.303 | −1.050 | −0.851 | −0.529 | −0.255 |
60 | −2.660 | −2.390 | −2.099 | −2.000 | −1.671 | −1.296 | −1.045 | −0.848 | −0.527 | −0.254 |
120 | −2.617 | −2.358 | −2.076 | −1.980 | −1.658 | −1.289 | −1.041 | −0.845 | −0.526 | −0.254 |
∞ | −2.576 | −2.326 | −2.054 | −1.960 | −1.645 | −1.282 | −1.036 | −0.842 | −0.524 | −0.253 |
Normal | −2.576 | −2.326 | −2.054 | −1.960 | −1.645 | −1.282 | −1.036 | −0.842 | −0.524 | −0.253 |
Probability of Larger Value (Upper Tail) | ||||||||||
.50 | .40 | .30 | .20 | .15 | .10 | .05 | .025 | .02 | .01 | .005 |
Probability of Smaller Value (Lower Tail) | ||||||||||
.50 | .60 | .70 | .80 | .85 | .90 | .95 | .975 | .98 | .99 | .995 |
0 | 0.289 | 0.617 | 1.061 | 1.386 | 1.886 | 2.920 | 4.303 | 4.849 | 6.965 | 9.925 |
0 | 0.271 | 0.569 | 0.941 | 1.190 | 1.533 | 2.132 | 2.776 | 2.999 | 3.747 | 4.604 |
0 | 0.265 | 0.553 | 0.906 | 1.134 | 1.440 | 1.943 | 2.447 | 2.612 | 3.143 | 3.707 |
0 | 0.262 | 0.546 | 0.889 | 1.108 | 1.397 | 1.860 | 2.306 | 2.449 | 2.896 | 3.355 |
0 | 0.260 | 0.542 | 0.879 | 1.093 | 1.372 | 1.812 | 2.228 | 2.359 | 2.764 | 3.169 |
0 | 0.259 | 0.539 | 0.873 | 1.083 | 1.356 | 1.782 | 2.179 | 2.303 | 2.681 | 3.055 |
0 | 0.258 | 0.537 | 0.868 | 1.076 | 1.345 | 1.761 | 2.145 | 2.264 | 2.624 | 2.977 |
0 | 0.258 | 0.535 | 0.865 | 1.071 | 1.337 | 1.746 | 2.120 | 2.235 | 2.583 | 2.921 |
0 | 0.257 | 0.534 | 0.862 | 1.067 | 1.330 | 1.734 | 2.101 | 2.214 | 2.552 | 2.878 |
0 | 0.257 | 0.533 | 0.860 | 1.064 | 1.325 | 1.725 | 2.086 | 2.197 | 2.528 | 2.845 |
0 | 0.256 | 0.531 | 0.856 | 1.058 | 1.316 | 1.708 | 2.060 | 2.167 | 2.485 | 2.787 |
0 | 0.256 | 0.530 | 0.854 | 1.055 | 1.310 | 1.697 | 2.042 | 2.147 | 2.457 | 2.750 |
0 | 0.255 | 0.529 | 0.852 | 1.052 | 1.306 | 1.690 | 2.030 | 2.133 | 2.438 | 2.724 |
0 | 0.255 | 0.529 | 0.851 | 1.050 | 1.303 | 1.684 | 2.021 | 2.123 | 2.423 | 2.704 |
0 | 0.254 | 0.527 | 0.848 | 1.045 | 1.296 | 1.671 | 2.000 | 2.099 | 2.390 | 2.660 |
0 | 0.254 | 0.526 | 0.845 | 1.041 | 1.289 | 1.658 | 1.980 | 2.076 | 2.358 | 2.617 |
0 | 0.253 | 0.524 | 0.842 | 1.036 | 1.282 | 1.645 | 1.960 | 2.054 | 2.326 | 2.576 |
0 | 0.253 | 0.524 | 0.842 | 1.036 | 1.282 | 1.645 | 1.960 | 2.054 | 2.326 | 2.576 |
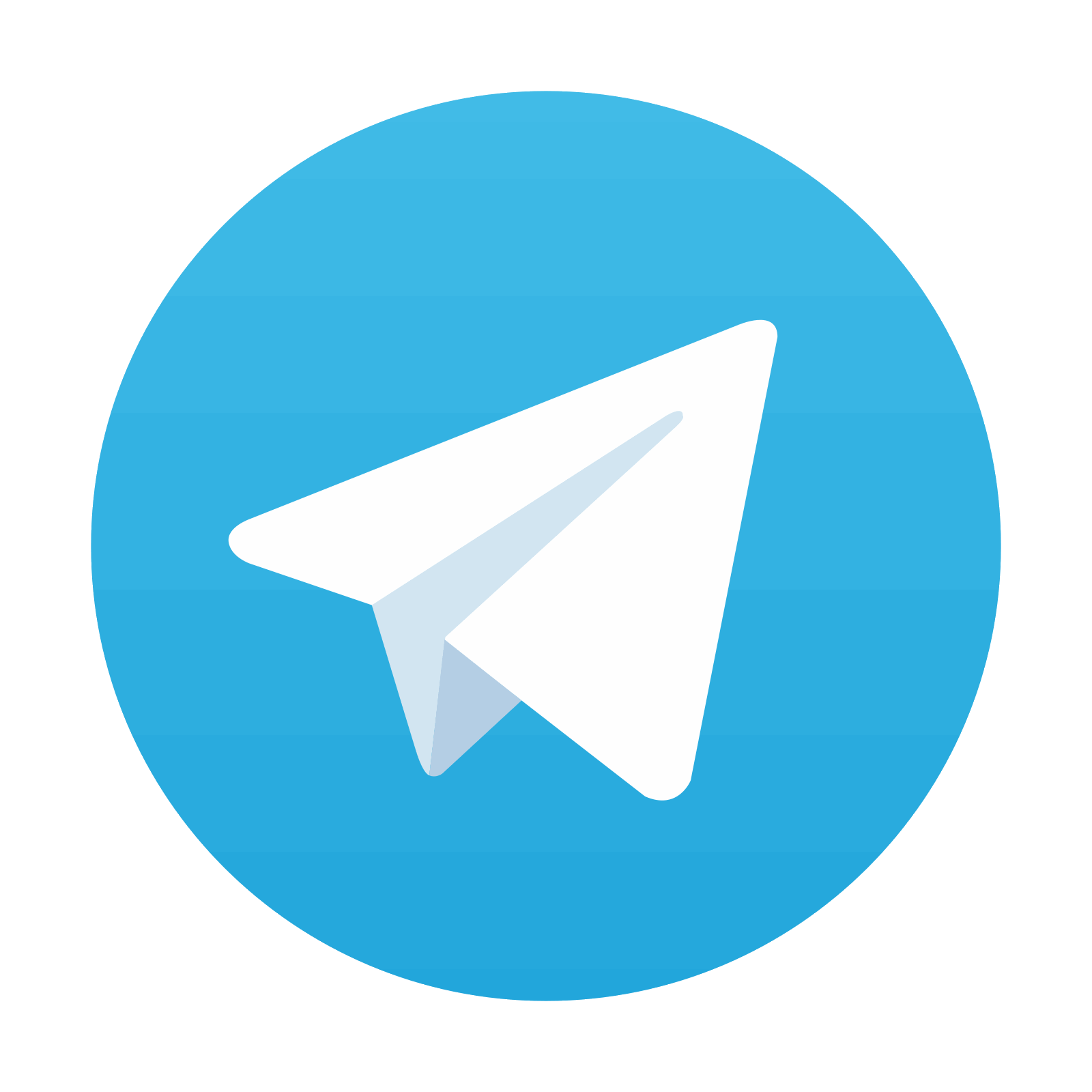
Stay updated, free articles. Join our Telegram channel

Full access? Get Clinical Tree
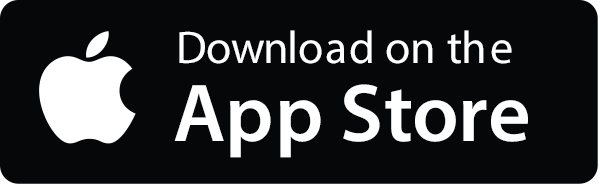

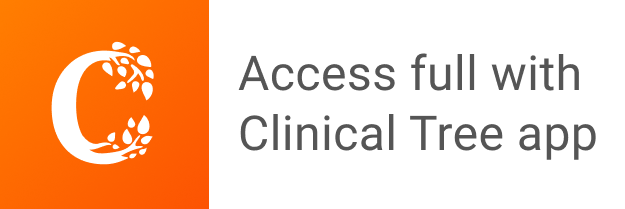