As we have just seen in Chapter 3, many investigations require comparing only two groups. In addition, as the last example in Chapter 3 illustrated, when there are more than two groups, analysis of variance only allows you to conclude that the data are not consistent with the hypothesis that all the samples were drawn from a single population. It does not help you decide which one or ones are most likely to differ from the others. To answer these questions, we now develop a procedure that is specifically designed to test for differences in two groups: the t test or Student’s t test. While we will develop the t test from scratch, we will eventually show that it is just a different way of doing an analysis of variance. In particular, we will see that F = t2 when there are two groups.
The t test is the most common statistical procedure in the medical literature; you can expect it to appear in more than half the papers you read in the general medical literature. In addition to being used to compare two group means, it is widely applied incorrectly to compare multiple groups, by doing all the pairwise comparisons, for example, by comparing more than one intervention with a control condition or the state of a patient at different times following an intervention. As we will see, this incorrect use increases the chances of rejecting the null hypothesis of no effect above the nominal level, say 5%, used to select the cutoff value for a “big” value of the test statistic t. In practical terms, this boils down to increasing the chances of reporting that some therapy had an effect when the evidence does not support this conclusion.
Suppose we wish to test a new drug that may be an effective diuretic. We assemble a group of 10 people and divide them at random into two groups, a control group that receives a placebo and a treatment group that receives the drug; then we measure their urine production for 24 hours. Figure 4-1A shows the resulting data. The average urine production of the group receiving the diuretic is 240 mL higher than that of the group receiving the placebo. Simply looking at Figure 4-1A, however, does not provide very convincing evidence that this difference is due to anything more than random sampling.
Figure 4-1.

(A) Results of a study in which five people were treated with a placebo and five people were treated with a drug thought to increase daily urine production. On the average, the five people who received the drug produced more urine than the placebo group. Are these data convincing evidence that the drug is an effective diuretic? (B) Results of a similar study with 20 people in each treatment group. The means and standard deviations associated with the two groups are similar to the results in panel A. Are these data convincing evidence that the drug is an effective diuretic? If you changed your mind, why did you do it?
Nevertheless, we pursue the problem and give the placebo or drug to another 30 people to obtain the results shown in Figure 4-1B. The mean responses of the two groups of people as well as the standard deviations are almost identical to those observed in the smaller samples shown in Figure 4-1A. Even so, most observers are more confident in claiming that the diuretic increased average urine output from the data in Figure 4-1B than the data in Figure 4-1A, even though the samples in each case are good representatives of the underlying population. Why?
As the sample size increases, most observers become more confident in their estimates of the population means so they can begin to discern a difference between the people taking the placebo or the drug. Recall that the standard error of the mean quantifies the uncertainty of the estimate of the true population mean based on a sample. Furthermore, as the sample size increases, the standard error of the mean decreases according to
where n is the sample size and σ is the standard deviation of the population from which the sample was drawn. As the sample size increases the uncertainty in the estimate of the difference of the means between the people who received placebo and the patients who received the drug decreases relative to the difference of the means. As a result, we become more confident that the drug actually has an effect. More precisely, we become less confident in the hypothesis that the drug had no effect, in which case the two samples of patients could be considered two samples drawn from a single population.
When this ratio is small we will conclude that the data are compatible with the hypothesis that both samples were drawn from a single population. When this ratio is large we will conclude that it is unlikely that the samples were drawn from a single population and assert that the treatment (e.g., the diuretic) produced an effect.
This logic, while differing in emphasis from that used to develop the analysis of variance, is essentially the same. In both cases, we are comparing the relative magnitude of the differences in the sample means with the amount of variability that would be expected from looking within the samples.
To compute the t ratio we need to know two things: the difference of the sample means and the standard error of this difference. Computing the difference of the sample means is easy; we simply subtract. Computing an estimate for the standard error of this difference is a bit more involved. We begin with a slightly more general problem, that of finding the standard deviation of the difference of two numbers drawn at random from the same population.
Figure 4-2A shows a population with 200 members. The mean is 0, and the standard deviation is 1. Now, suppose we draw two samples at random and compute their difference. Figure 4-2B shows this result for the two members indicated by solid circles in Figure 4-2A. Drawing five more pairs of samples (indicated by different symbols in Fig. 4-2A) and computing their differences yields the corresponding shaded points in Figure 4-2B. Note that there seems to be more variability in the differences of the samples than in the samples themselves. Figure 4-2C shows the results of Figure 4-2B, together with the results of drawing another 50 pairs of numbers at random and computing their differences. The standard deviation of the population of differences is about 40% larger than the standard deviation of the population from which the samples were drawn.
Figure 4-2.

If one selects pairs of members of the population in panel A at random and computes the difference the population of differences, shown in panel B, has a wider variance than the original population. Panel C shows another 100 values for differences of pairs of members selected at random from the population in A to make this point again.
In fact, it is possible to demonstrate mathematically that the variance of the difference (or sum) of two variables selected at random equals the sum of the variances of the two populations from which the samples were drawn. In other words, if X is drawn from a population with standard deviation σx and Y is drawn from a population with standard deviation σy, the distribution of all possible values of X − Y (or X + Y) will have variance
This result should seem reasonable to you because when you select pairs of values that are on opposite (the same) sides of the population mean and compute their difference (sum), the result will be even farther from the mean. Returning to the example in Figure 4-2, we can observe that both the first and second numbers were drawn from the same population whose variance was 1 and so the variance of the difference should be
Since the standard deviation is the square root of the variance, the standard deviation of the population of differences will be times the standard deviation of the original population, or about 40% bigger, confirming our earlier subjective impression.*
When we wish to estimate the variance in the difference or sum of members of two populations based on the observations, we simply replace the population variances σ2 in the equation above with the estimates of the variances computed from our samples:
The standard error of the mean is just the standard deviation of the population of all possible sample means of samples of size n, and so we can find the standard error of the difference of two means using the equation above. Specifically,
* The fact that the sum of randomly selected variables has a variance equal to the sum of the variances of the individual numbers explains why the standard error of the mean equals the standard deviation divided by . Suppose we draw n numbers at random from a population with standard deviation s. The mean of these numbers will be
Alternatively, we can write t in terms of the sample standard deviations rather than the standard errors of the mean:
If the hypothesis that the two samples were drawn from the same population is true, the variances and
computed from the two samples are both estimates of the same population variance σ 2. Therefore, we replace the two different estimates of the population variance in the equation above with a single estimate, s2, that is obtained by averaging these two separate estimates:
This is called the pooled-variance estimate since it is obtained by pooling the two estimates of the population variance to obtain a single estimate. The t test statistic based on the pooled-variance estimate is
The specific value of t one obtains from any two samples depends not only on whether or not there actually is a difference in the means of the populations from which the samples were drawn but also on which specific individuals happened to be selected for the samples. Thus, as for F, there will be a range of possible values that t can have, even when both samples are drawn from the same population. Since the means computed from the two samples will generally be close to the mean of the population from which they were drawn, the value of t will tend to be small when the two samples are drawn from the same population. Therefore, we will use the same procedure to test hypotheses with t as we did with F in Chapter 3. Specifically, we will compute t from the data then reject the assertion that the two samples were drawn from the same population if the resulting value of t is “big.”
Let us return to the problem of assessing the value of the diuretic we were discussing earlier. Suppose the entire population of interest contains 200 people. In addition, we will assume that the diuretic had no effect, so that the two groups of people being studied can be considered to represent two samples drawn from a single population. Figure 4-3A shows this population, together with two samples of 10 people each selected at random for study. The people who received the placebo are shown as dark circles, and the people who received the diuretic are shown as lighter circles. The lower part of Figure 4-3A shows the data as they would appear to the investigator together with the mean and standard deviations computed from each of the two samples. Looking at these data certainly does not suggest that the diuretic had any effect. The value of t associated with these samples is −0.2.
Figure 4-3.


A population of 200 individuals and two groups selected at random for study of a drug designed to increase urine production but which is totally ineffective. The people shown as dark circles received the placebo and those with the lighter circles received the drug. An investigator would not see the entire population but just the information as reflected in the lower part of panel A; nevertheless, the two samples show very little difference and it is unlikely that one would have concluded that the drug had an effect on urine production. Of course, there is nothing special about the two random samples shown in panel A, and an investigator could just as well have selected the two groups of people in panel B for study. There is more difference between these two groups than the two shown in panel A and there is a chance that the investigator would think that this difference is due to the drug’s effect on urine production rather than simple random sampling. Panel C shows yet another pair of random samples the investigator might have drawn for the study.
Of course, there is nothing special about these two samples and we could just as well have selected two different groups of people to study. Figure 4-3B shows another collection of people that could have been selected at random to receive the placebo (dark circles) or diuretic (light circles). Not surprisingly, these two samples differ from each other as well as the samples selected in Figure 4-3A. Given only the data in the lower part of Figure 4-3B we might think that the diuretic increases urine production. The t value associated with these data is −2.1. Figure 4-3C shows yet another pair of samples. They differ from each other and the other samples considered in Figure 4-3A and 4-3B. The samples in Figure 4-3C yield a value of 0 for t.
We could continue this process for quite a long time since there are more than 1027 different pairs of samples of 10 people each that we could draw from the population of 200 individuals shown in Figure 4-3A. We can compute a value of t for each of these 1027 different pairs of samples. Figure 4-4 shows the values of t associated with 200 different pairs of random samples of 10 people each drawn from the original population, including the three specific pairs of samples shown in Figure 4-3. The distribution of possible t values is symmetrical about t = 0 because it does not matter which of the two samples we subtract from the other. As predicted, most of the resulting values of t are close to zero; t rarely is below about −2 or above +2.
Figure 4-4.

The results of 200 studies like that described in Figure 4-3; the three specific studies from Figure 4-3 are indicated in panel A. Note that most values of the t statistic cluster around 0, but it is possible for some values of t to be quite large, exceeding 1.5 or 2. Panel B shows that there are only 10 chances in 200 of t exceeding 2.1 in magnitude if the two samples were drawn from the same population. If one continues examining all possible samples drawn from the population and our pairs of samples drawn from the same population, one obtains a distribution of all possible t values which becomes the smooth curve in panel C. In this case, one defines the critical value of t by saying that it is unlikely that this value of t statistic was observed under the hypothesis that the drug had no effect by taking the 5% most extreme error areas under the tails of distribution and selecting the t value corresponding to the beginning of this region. Panel D shows that if one required a more stringent criterion for rejecting the hypothesis for no difference by requiring that t be in the most extreme 1% of all possible values, the cutoff value of t is 2.878.
Figure 4-4 allows us to determine what a “big” t is. Figure 4-4B shows that t will be less than −2.1 or greater than +2.1 10 out of 200, or 5% of the time. In other words, there is only a 5% chance of getting a value of t more extreme than −2.1 or +2.1 when the two samples are drawn from the same population. Just as with the F distribution, the number of possible t values rapidly increases beyond 1027 as the population size grows, and the distribution of possible t values approaches a smooth curve. Figure 4-4C shows the result of this limiting process. We define the cutoff values for t that are large enough to be called “big” on the basis of the total area in the two tails. Figure 4-4C shows that only 5% of the possible values of t will lie beyond −2.1 or +2.1 when the two samples are drawn from a single population. When the data are associated with a value of t beyond this range, it is customary to conclude that the data are inconsistent with the null hypothesis of no difference between the two samples and report that there was a difference in treatment.
The extreme values of t that lead us to reject the hypothesis of no difference lie in both tails of the distribution. Therefore, the approach we are taking is sometimes called a two-tailed t test. Occasionally, people use a one-tailed t test, and there are indeed cases where this is appropriate. One should be suspicious of such one-tailed tests, however, because the cutoff value for calling t “big” for a given value of P is smaller. In reality, people are almost always looking for a difference between the control and treatment groups so a two-tailed test is appropriate. This book always assumes a two-tailed test.
Note that the data in Figure 4-3B are associated with a t value of −2.1, which we have decided to consider “big.” If all we had were the data shown in Figure 4-4B, we would conclude that the observations were inconsistent with the hypothesis that the diuretic had no effect and report that it increased urine production, and even though we did the statistical analysis correctly, our conclusion about the drug would be wrong.
Reporting P< .05 means that if the treatment had no effect, there is less than a 5% chance of getting a value of t from the data as far or farther from 0 as the critical value for t to be called “big.” It does not mean it is impossible to get such a large value of t when the treatment has no effect. We could, of course, be more conservative and say that we will reject the hypothesis of no difference between the populations from which the samples were drawn if t is in the most extreme 1% of possible values. Figure 4-4D shows that this would require t to be beyond −2.88 or +2.88 in this case, so we would not erroneously conclude that the drug had an effect on urine output in any of the specific examples shown in Figure 4-3. In the long run, however, we will make such errors about 1% of the time. The price of this conservatism is decreasing the chances of concluding that there is a difference when one really exists. Chapter 6 discusses this trade-off in more detail.
The critical values of t, like F, have been tabulated and depend not only on the level of confidence with which one rejects the hypothesis of no difference—the P value—but also on the sample size. As with the F distribution, this dependence on sample size enters the table as the degrees of freedom, ν, which is equal to 2(n − 1) for this t test, where n is the size of each sample. As the sample size increases the value of t needed to reject the hypothesis of no difference decreases. In other words, as sample size increases it becomes possible to detect smaller differences with a given level of confidence. Reflecting on Figure 4-1 should convince you that this is reasonable.
It is easy to generalize the t test to handle problems in which there are different numbers of members in the two samples being studied. Recall that t is defined by
in which and
are the standard errors of the means of the two samples. If the first sample is of size n1 and the second sample contains n2 members,
in which s1 and s2 are the standard deviations of the two samples. Use these definitions to rewrite the definition of t in terms of the sample standard deviations
This is the definition of t for comparing two samples of unequal size. There are ν = n1 + n2 − 2 degrees of freedom.
The study by Fejes and colleagues about the relationship of cell phone use and rapid sperm motility we discussed in Chapter 3 had two observational groups, 61 men who used cell phones less than 15 minutes/day and 61 men who used cell phones more than 60 minutes/day, so we can analyze their data using a t test, as well as an analysis of variance. From Figure 3-7, the mean percentage of rapidly mobile sperm was 49% for the low use group and 41% for the high use group. The standard deviations were 21% and 22%, respectively. Because the sample sizes are equal,*
with ν = 2(n − 1) = 2(61 − 1) = 120 degrees of freedom. Table 4-1 shows that the magnitude of t should only exceed 1.980 only 5% of the time by chance when the null hypothesis is true, in this case, that cell phone exposure does not affect rapid sperm motility (P < .05). Since the magnitude of t associated with the data exceeds 1.980, we reject the null hypothesis and conclude that cell phone use is associated with rapid sperm motility.
Probability of Greater Value (P) | |||||||||
---|---|---|---|---|---|---|---|---|---|
ν | 0.50 | 0.20 | 0.10 | 0.05 | 0.02 | 0.01 | 0.005 | 0.002 | 0.001 |
1 | 1.000 | 3.078 | 6.314 | 12.706 | 31.821 | 63.657 | 127.321 | 318.309 | 636.619 |
2 | 0.816 | 1.886 | 2.920 | 4.303 | 6.965 | 9.925 | 14.089 | 22.327 | 31.599 |
3 | 0.765 | 1.638 | 2.353 | 3.182 | 4.541 | 5.841 | 7.453 | 10.215 | 12.924 |
4 | 0.741 | 1.533 | 2.132 | 2.776 | 3.747 | 4.604 | 5.598 | 7.173 | 8.610 |
5 | 0.727 | 1.476 | 2.015 | 2.571 | 3.365 | 4.032 | 4.773 | 5.893 | 6.869 |
6 | 0.718 | 1.440 | 1.943 | 2.447 | 3.143 | 3.707 | 4.317 | 5.208 | 5.959 |
7 | 0.711 | 1.415 | 1.895 | 2.365 | 2.998 | 3.449 | 4.029 | 4.785 | 5.408 |
8 | 0.706 | 1.397 | 1.860 | 2.306 | 2.896 | 3.355 | 3.833 | 4.501 | 5.041 |
9 | 0.703 | 1.383 | 1.833 | 2.262 | 2.821 | 3.250 | 3.690 | 4.297 | 4.781 |
10 | 0.700 | 1.372 | 1.812 | 2.228 | 2.764 | 3.169 | 3.581 | 4.144 | 4.587 |
11 | 0.697 | 1.363 | 1.796 | 2.201 | 2.718 | 3.106 | 3.497 | 4.025 | 4.437 |
12 | 0.695 | 1.356 | 1.782 | 2.179 | 2.681 | 3.055 | 3.428 | 3.930 | 4.318 |
13 | 0.694 | 1.350 | 1.771 | 2.160 | 2.650 | 3.012 | 3.372 | 3.852 | 4.221 |
14 | 0.692 | 1.345 | 1.761 | 2.145 | 2.624 | 2.977 | 3.326 | 3.787 | 4.140 |
15 | 0.691 | 1.341 | 1.753 | 2.131 | 2.602 | 2.947 | 3.286 | 3.733 | 4.073 |
16 | 0.690 | 1.337 | 1.746 | 2.120 | 2.583 | 2.921 | 3.252 | 3.686 | 4.015 |
17 | 0.689 | 1.333 | 1.740 | 2.110 | 2.567 | 2.898 | 3.222 | 3.646 | 3.965 |
18 | 0.688 | 1.330 | 1.734 | 2.101 | 2.552 | 2.878 | 3.197 | 3.610 | 3.922 |
19 | 0.688 | 1.328 | 1.729 | 2.093 | 2.539 | 2.861 | 3.174 | 3.579 | 3.883 |
20 | 0.687 | 1.325 | 1.725 | 2.086 | 2.528 | 2.845 | 3.153 | 3.552 | 3.850 |
21 | 0.686 | 1.323 | 1.721 | 2.080 | 2.518 | 2.831 | 3.135 | 3.527 | 3.819 |
22 | 0.686 | 1.321 | 1.717 | 2.074 | 2.508 | 2.819 | 3.119 | 3.505 | 3.792 |
23 | 0.685 | 1.319 | 1.714 | 2.069 | 2.500 | 2.807 | 3.104 | 3.485 | 3.768 |
24 | 0.685 | 1.318 | 1.711 | 2.064 | 2.492 | 2.797 | 3.091 | 3.467 | 3.745 |
25 | 0.684 | 1.316 | 1.708 | 2.060 | 2.485 | 2.787 | 3.078 | 3.450 | 3.725 |
26 | 0.684 | 1.315 | 1.706 | 2.056 | 2.479 | 2.779 | 3.067 | 3.435 | 3.707 |
27 | 0.684 | 1.314 | 1.703 | 2.052 | 2.473 | 2.771 | 3.057 | 3.421 | 3.690 |
28 | 0.683 | 1.313 | 1.701 | 2.048 | 2.467 | 2.763 | 3.047 | 3.408 | 3.674 |
29 | 0.683 | 1.311 | 1.699 | 2.045 | 2.462 | 2.756 | 3.038 | 3.396 | 3.659 |
30 | 0.683 | 1.310 | 1.697 | 2.042 | 2.457 | 2.750 | 3.030 | 3.385 | 3.646 |
31 | 0.682 | 1.309 | 1.696 | 2.040 | 2.453 | 2.744 | 3.022 | 3.375 | 3.633 |
32 | 0.682 | 1.309 | 1.694 | 2.037 | 2.449 | 2.738 | 3.015 | 3.365 | 3.622 |
33 | 0.682 | 1.308 | 1.692 | 2.035 | 2.445 | 2.733 | 3.008 | 3.356 | 3.611 |
34 | 0.682 | 1.307 | 1.691 | 2.032 | 2.441 | 2.728 | 3.002 | 3.348 | 3.601 |
35 | 0.682 | 1.306 | 1.690 | 2.030 | 2.438 | 2.724 | 2.996 | 3.340 | 3.591 |
36 | 0.681 | 1.306 | 1.688 | 2.028 | 2.434 | 2.719 | 2.990 | 3.333 | 3.582 |
37 | 0.681 | 1.305 | 1.687 | 2.026 | 2.431 | 2.715 | 2.985 | 3.326 | 3.574 |
38 | 0.681 | 1.304 | 1.686 | 2.024 | 2.429 | 2.712 | 2.980 | 3.319 | 3.566 |
39 | 0.681 | 1.304 | 1.685 | 2.023 | 2.426 | 2.708 | 2.976 | 3.313 | 3.558 |
40 | 0.681 | 1.303 | 1.684 | 2.021 | 2.423 | 2.704 | 2.971 | 3.307 | 3.551 |
42 | 0.680 | 1.302 | 1.682 | 2.018 | 2.418 | 2.698 | 2.963 | 3.296 | 3.538 |
44 | 0.680 | 1.301 | 1.680 | 2.015 | 2.414 | 2.692 | 2.956 | 3.286 | 3.526 |
46 | 0.680 | 1.300 | 1.679 | 2.013 | 2.410 | 2.687 | 2.949 | 3.277 | 3.515 |
48 | 0.680 | 1.299 | 1.677 | 2.011 | 2.407 | 2.682 | 2.943 | 3.269 | 3.505 |
50 | 0.679 | 1.299 | 1.676 | 2.009 | 2.403 | 2.678 | 2.937 | 2.261 | 3.496 |
52 | 0.679 | 1.298 | 1.675 | 2.007 | 2.400 | 2.674 | 2.932 | 3.255 | 3.488 |
54 | 0.679 | 1.297 | 1.674 | 2.005 | 2.397 | 2.670 | 2.927 | 3.248 | 3.480 |
56 | 0.679 | 1.297 | 1.673 | 2.003 | 2.395 | 2.667 | 2.923 | 3.242 | 3.473 |
58 | 0.679 | 1.296 | 1.672 | 2.002 | 2.392 | 2.663 | 2.918 | 3.237 | 3.466 |
60 | 0.679 | 1.296 | 1.671 | 2.000 | 2.390 | 2.660 | 2.915 | 3.232 | 3.460 |
62 | 0.678 | 1.295 | 1.670 | 1.999 | 2.388 | 2.657 | 2.911 | 3.227 | 3.454 |
64 | 0.678 | 1.295 | 1.669 | 1.998 | 2.386 | 2.655 | 2.908 | 3.223 | 3.449 |
66 | 0.678 | 1.295 | 1.668 | 1.997 | 2.384 | 2.652 | 2.904 | 3.218 | 3.444 |
68 | 0.678 | 1.294 | 1.668 | 1.995 | 2.382 | 2.650 | 2.902 | 3.214 | 3.439 |
70 | 0.678 | 1.294 | 1.667 | 1.994 | 2.381 | 2.648 | 2.899 | 3.211 | 3.435 |
72 | 0.678 | 1.293 | 1.666 | 1.993 | 2.379 | 2.646 | 2.896 | 3.207 | 3.431 |
74 | 0.678 | 1.293 | 1.666 | 1.993 | 2.378 | 2.644 | 2.894 | 3.204 | 3.427 |
76 | 0.678 | 1.293 | 1.665 | 1.992 | 2.376 | 2.642 | 2.891 | 3.201 | 3.423 |
78 | 0.678 | 1.292 | 1.665 | 1.991 | 2.375 | 2.640 | 2.889 | 3.198 | 3.420 |
80 | 0.678 | 1.292 | 1.664 | 1.990 | 2.374 | 2.639 | 2.887 | 3.195 | 3.416 |
90 | 0.677 | 1.291 | 1.662 | 1.987 | 2.368 | 2.632 | 2.878 | 3.183 | 3.402 |
100 | 0.677 | 1.290 | 1.660 | 1.984 | 2.364 | 2.626 | 2.871 | 3.174 | 3.390 |
120 | 0.677 | 1.289 | 1.658 | 1.980 | 2.358 | 2.617 | 2.860 | 3.160 | 3.373 |
140 | 0.676 | 1.288 | 1.656 | 1.977 | 2.353 | 2.611 | 2.852 | 3.149 | 3.361 |
160 | 0.676 | 1.287 | 1.654 | 1.975 | 2.350 | 2.607 | 2.846 | 3.142 | 3.352 |
180 | 0.676 | 1.286 | 1.653 | 1.973 | 2.347 | 2.603 | 2.842 | 3.136 | 3.345 |
200 | 0.676 | 1.286 | 1.653 | 1.972 | 2.345 | 2.601 | 2.839 | 3.131 | 3.340 |
∞ | 0.6745 | 1.2816 | 1.6449 | 1.9600 | 2.3263 | 2.5758 | 2.8070 | 3.0902 | 3.2905 |
Normal | 0.6745 | 1.2816 | 1.6449 | 1.9600 | 2.3263 | 2.5758 | 2.8070 | 3.0902 | 3.2905 |
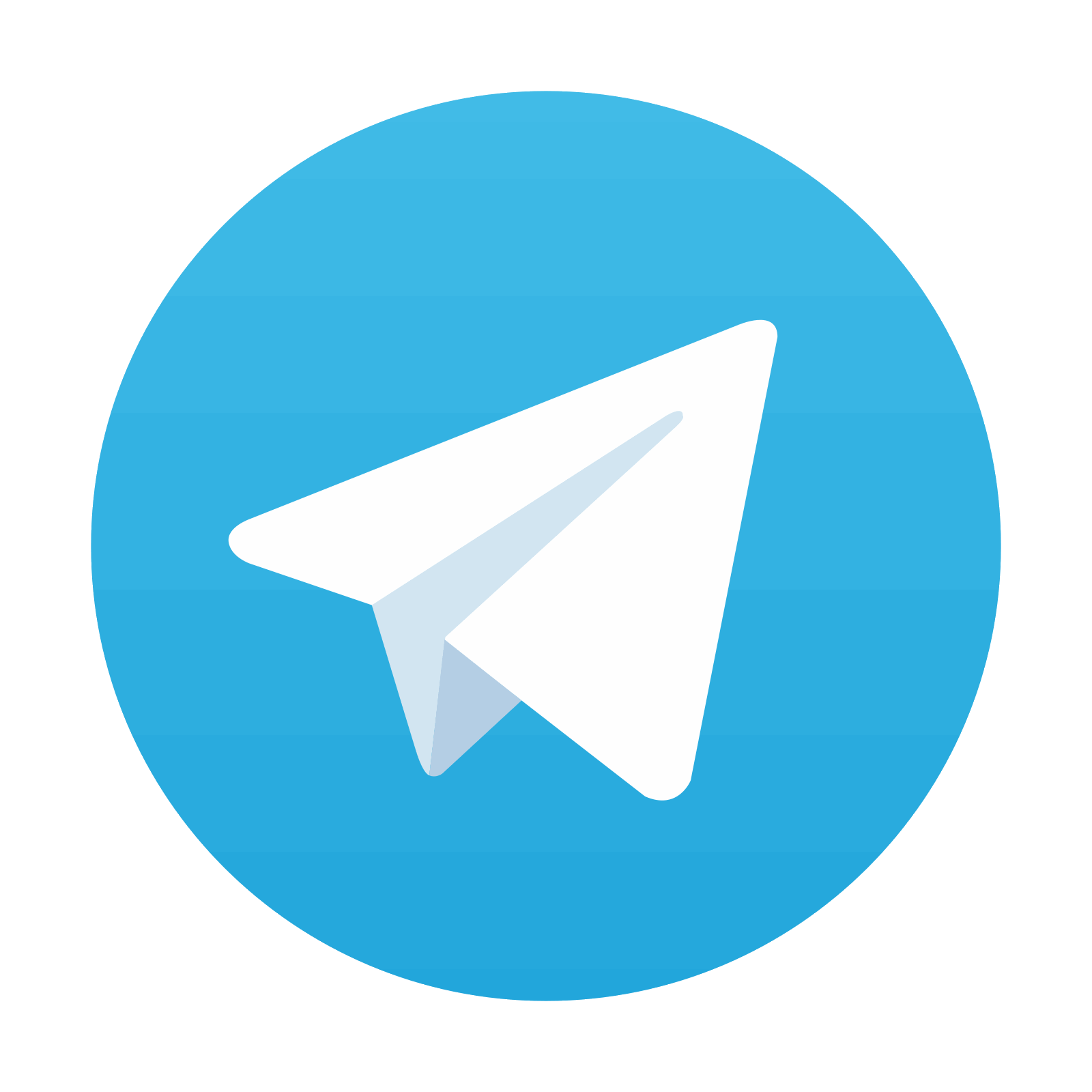
Stay updated, free articles. Join our Telegram channel

Full access? Get Clinical Tree
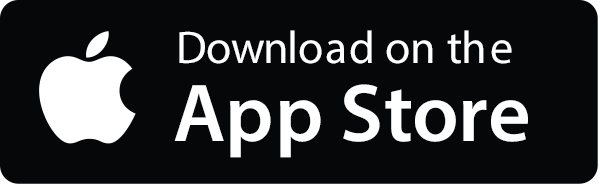

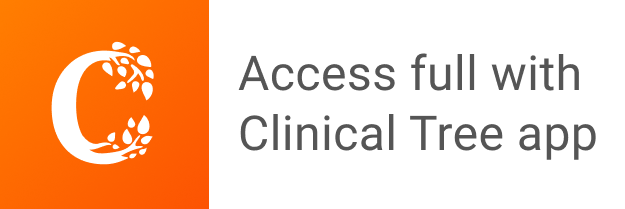