Analysis of variance, including the t tests, is widely used to test the hypothesis that one or more treatments had no effect on the mean of some observed variable. All forms of analysis of variance, including the t tests, are based on the assumption that the observations are drawn from normally distributed populations in which the variances are the same even if the treatments change the mean responses. These assumptions are often satisfied well enough to make analysis of variance an extremely useful statistical procedure. On the other hand, experiments often yield data that are not compatible with these assumptions. In addition, there are often problems in which the observations are measured on an ordinal scale rather than an interval scale and may not be amenable to an analysis of variance. This chapter develops analogs to the t tests and analysis of variance based on ranks of the observations rather than the observations themselves. This approach uses information about the relative sizes of the observations without assuming anything about the specific nature of the population they were drawn from.
We will begin with the nonparametric analog to the unpaired and paired t tests, the Mann-Whitney rank-sum test, and Wilcoxon signed-rank test. Then we will present the analogs of one-way analysis of variance, the Kruskal-Wallis analysis of variance based on ranks, and the Friedman repeated measures analysis of variance based on ranks.
As already noted, analysis of variance is called a parametric statistical method because it is based on estimates of the two population parameters, the mean and standard deviation (or variance), that completely define a normal distribution. Given the assumption that the samples are drawn from normally distributed populations, one can compute the distributions of the F or t test statistics that will occur in all possible experiments of a given size when the treatments have no effect. The critical values that define a value of F or t can then be obtained from that distribution. When the assumptions of parametric statistical methods are satisfied, they are the most powerful tests available.
If the populations the observations were drawn from are not normally distributed (or are not reasonably compatible with other assumptions of a parametric method, such as equal variances in all the treatment groups), parametric methods become quite unreliable because the mean and standard deviation, the key elements of parametric statistics, no longer completely describe the population. In fact, when the population substantially deviates from normality, interpreting the mean and standard deviation in terms of a normal distribution can produce a very misleading picture.
For example, recall our discussion of the distribution of heights of the entire population of Jupiter. The mean height of all Jovians is 37.6 cm in Figure 2-3A and the standard deviation is 4.5 cm. Rather than being equally distributed about the mean, the population is skewed toward taller heights. Specifically, the heights of Jovians range from 31 to 52 cm, with most heights around 35 cm. Figure 2-3B shows what the population of heights would have been if, instead of being skewed toward taller heights, they had been normally distributed with the same mean and standard deviation as the actual population (in Figure 2-3A). The heights would have ranged from 26 to 49 cm, with most heights around 37 to 38 cm. Simply looking at Figure 2-3 should convince you that envisioning a population on the basis of the mean and standard deviation can be quite misleading if the population does not, at least approximately, follow the normal distribution.
The same thing is true of statistical tests that are based on the normal distribution. When the population the samples were drawn from does not at least approximately follow the normal distribution, these tests can be quite misleading. In such cases, it is possible to use the ranks of the observations rather than the observations themselves to compute statistics that can be used to test hypotheses. By using ranks rather than the actual measurements it is possible to retain much of the information about the relative size of responses without making any assumptions about how the population the samples were drawn from is distributed. Since these tests are not based on the parameters of the underlying population, they are called nonparametric or distribution-free methods.* All the methods we will discuss require only that the distributions under the different treatments have similar shapes, but there is no restriction on what those shapes are.†
When the observations are drawn from normally distributed populations, the nonparametric methods in this chapter are about 95% as powerful as the analogous parametric methods. As a result, power for these tests can be estimated by computing the power of the analogous parametric test. When the observations drawn from populations that are not normally distributed, nonparametric methods are not only more reliable but also more powerful than parametric methods.
Unfortunately, you can never observe the entire population. So how can you tell whether the assumptions such as normality are met, to permit using the parametric tests such as analysis of variance? The simplest approach is to plot the observations and look at them. Do they seem compatible with the assumptions that they were drawn from normally distributed populations with roughly the same variances, that is, within a factor of 2 to 3 of each other? If so, you are probably safe in using parametric methods. If, on the other hand, the observations are heavily skewed (suggesting a population such as the Jovians in Fig. 2-3A) or appear to have more than one peak, you probably will want to use a nonparametric method. When the standard deviation is about the same size or larger than the mean and the variable can take on only positive values, this is an indication that the distribution is skewed. (A normally distributed variable would have to take on negative values.) In practice, these simple rules of thumb are often all you will need.
There are two ways to make this procedure more objective. The first is to plot the observations as a normal probability plot. A normal probability plot has a distorted vertical scale that makes normally distributed observations plot as a straight line (just as exponential functions plot as a straight line on a semilogarithmic graph). Examining how straight the line is will show how compatible the observations are with a normal distribution. One can also construct a χ2 statistic to test how closely the observed data agree with those expected if the population is normally distributed with the same mean and standard deviation. Since in practice simply looking at the data is generally adequate, we will not discuss these approaches in detail.‡
Unfortunately, none of these methods is especially convincing one way or the other for the small sample sizes common in biomedical research, and your choice of approach (i.e., parametric versus nonparametric) often has to be based more on judgment and preference than hard evidence.
One informal approach is to do the analysis with both the applicable parametric and nonparametric methods, then compare the results. If the data are from a normal population, then the parametric method should be more sensitive (and so provide a lower P value), whereas if there is substantial nonnormality then the nonparametric method should be more sensitive (and so provide the lower P value). If the data are only slightly nonnormal, the two approaches should give similar results.
Things basically come down to the following difference of opinion: Some people think that in the absence of evidence that the data were not drawn from a normally distributed population, one should use parametric tests because they are more powerful and more widely used. These people say that you should use a nonparametric test only when there is positive evidence that the populations under study are not normally distributed. Others point out that the nonparametric methods discussed in this chapter are 95% as powerful as parametric methods when the data are from normally distributed populations and more reliable when the data are not from normally distributed populations. They also believe that investigators should assume as little as possible when analyzing their data. They therefore recommend that nonparametric methods be used except when there is positive evidence that parametric methods are suitable. At the moment, there is no definitive answer stating which attitude is preferable. And there probably never will be such an answer.
* The methods in this chapter are obviously not the first nonparametric methods we have encountered. The χ2 for analysis of nominal data in contingency tables in Chapter 5, the Spearman rank correlation to analyze ordinal data in Chapter 8, and McNemar’s test in Chapter 9 are three widely used nonparametric methods.
† They also require that the distributions be continuous (so that ties are impossible) to derive the mathematical forms of the sampling distributions used to define the critical values of the various test statistics. In practice, however, the continuity restriction is not important, and the methods can be (and are) applied to observations with tied measurements.
When we developed the analysis of variance, t test, and Pearson product-moment correlation, we began with a specific (normally distributed) population and examined the values of the test statistic associated with all possible samples of a given size that could be selected from that population. The situation is different for methods based on ranks rather than the actual observations. We will replace the actual observations with their ranks, then focus on the population of all possible combinations of ranks. Since all samples have a finite number of members, we can simply list all the different possible ways to rank the members to obtain the distribution of possible values for the test statistic when the treatment has no effect.
To illustrate this process but keep this list relatively short, let us analyze a small experiment in which three people take a placebo and four people take a drug that is thought to be a diuretic. Table 10-1 shows the daily urine production observed in this experiment. Table 10-1 also shows the ranks of all the observations without regard to which experimental group they fall in; the smallest observed urine production is ranked 1 and the largest one is ranked 7. If the drug affected daily urine production, we would expect the rankings in the control group to be lower (or higher, if the drug decreased urine production) than the ranks for the treatment group. We will use the sum of ranks in the smaller group (in this case, the control group) as our test statistic T. The control-group ranks add up to 9.
Is the value of T = 9 sufficiently extreme to justify rejecting the hypothesis that the drug had no effect?
To answer this question, we examine the population of all possible rankings of the seven observations divided into two groups, one with 3 individuals and one with 4, to see how likely we are to get a rank sum as extreme as that associated in Table 10-1. Notice that we are no longer discussing the actual observations but their ranks, so our results will apply to any experiment in which there are two samples, one containing three individuals and the other containing four individuals, regardless of the nature of the underlying populations.
We begin with the hypothesis that the drug did not affect urine production, so that the ranking pattern in Table 10-1 is just due to chance. To estimate the chances of getting this pattern when the two samples were drawn from a single population, we need not engage in any fancy mathematics, we just list all the possible rankings that could have occurred. Table 10-2 shows all 35 different ways the ranks could have been arranged with three people in one group and four in the other. The crosses indicate a person in the placebo group, and the blanks indicate a person in the treatment group. The right-hand column shows the sum of ranks for the people in the smaller (placebo) group for each possible combination of ranks. Figure 10-1 shows the distribution of possible values of our test statistic, the sum of ranks of the smaller group T that can occur when the treatment has no effect. While this distribution looks a little like the t distribution in Figure 4-5, there is a very important difference. Whereas the t distribution is continuous and, in theory, is based on an infinitely large collection of possible values of the t test statistic, Figure 10-1 shows every possible value of the sum-of-ranks test statistic T.
Rank | |||||||
---|---|---|---|---|---|---|---|
1 | 2 | 3 | 4 | 5 | 6 | 7 | Rank Sum T |
X | X | X | 6 | ||||
X | X | X | 7 | ||||
X | X | X | 8 | ||||
X | X | X | 9 | ||||
X | X | X | 10 | ||||
X | X | X | 8 | ||||
X | X | X | 9 | ||||
X | X | X | 10 | ||||
X | X | X | 11 | ||||
X | X | X | 10 | ||||
X | X | X | 11 | ||||
X | X | X | 12 | ||||
X | X | X | 12 | ||||
X | X | X | 13 | ||||
X | X | X | 14 | ||||
X | X | X | 9 | ||||
X | X | X | 10 | ||||
X | X | X | 11 | ||||
X | X | X | 12 | ||||
X | X | X | 11 | ||||
X | X | X | 12 | ||||
X | X | X | 13 | ||||
X | X | X | 13 | ||||
X | X | X | 14 | ||||
X | X | X | 15 | ||||
X | X | X | 12 | ||||
X | X | X | 13 | ||||
X | X | X | 14 | ||||
X | X | X | 14 | ||||
X | X | X | 15 | ||||
X | X | X | 16 | ||||
X | X | X | 15 | ||||
X | X | X | 16 | ||||
X | X | X | 17 | ||||
X | X | X | 18 |
Since there are 35 possible ways to combine the ranks, there is 1 chance in 35 of getting rank sums of 6, 7, 17, or 18; 2 chances in 35 of getting 8 or 16; 3 chances in 35 of getting 9 or 15; 4 chances in 35 of getting 10, 11, 13, or 14; and 5 chances in 35 of getting 12. What are the chances of getting an extreme value of T? There is a 2/35 = .057 = 5.7% chance of obtaining T = 6 or T = 18 when the treatment has no effect. We use these numbers as the critical values to define extreme values of T and reject the hypothesis of no treatment effect. Hence, the value of T = 9 associated with the observations in Table 10-1 is not extreme enough to justify rejecting the hypothesis that the drug has no effect on urine production.
Notice that in this case T = 6 and T = 18 correspond to P = .057. Since T can take on only integer values, P can take on only discrete values. As a result, tables of critical values of T present pairs of values that define the proportion of possible values nearest traditional critical P values, for example, 5% and 1%, but the exact P values defined by these critical values generally do not equal 5% and 1% exactly. Table 10-3 presents these critical values. nS and nB are the number of members in the smaller and larger samples group. The table gives the critical values of T that come nearest defining the most extreme 5% and 1% of all possible values of T that will occur if the treatment has no effect, as well as the exact proportion of possible T values defined by the critical values. For example, Table 10-3 shows that 7 and 23 define the 4.8% most extreme possible values of the rank sum of the smaller of two sample groups T when nS = 3 and nB = 6.
Probability Levels Near | |||||
---|---|---|---|---|---|
.05 | .01 | ||||
nS | nB | Critical Values | P | Critical Values | P |
3 | 4 | 6,18 | .057 | ||
5 | 6,21 | .036 | |||
5 | 7,20 | .071 | |||
6 | 7,23 | .048 | 6,24 | .024 | |
7 | 7,26 | .033 | 6,27 | .017 | |
7 | 8,25 | .067 | |||
8 | 8,28 | .042 | 6,30 | .012 | |
4 | 4 | 11,25 | .057 | 10,26 | .026 |
5 | 11,29 | .032 | 10,30 | .016 | |
5 | 12,28 | .063 | |||
6 | 12,32 | .038 | 10,34 | .010 | |
7 | 13,35 | .042 | 10,38 | .012 | |
8 | 14,38 | .048 | 11,41 | .008 | |
8 | … . | … . | 12,40 | .016 | |
5 | 5 | 17,38 | .032 | 15,40 | .008 |
5 | 18,37 | .056 | 16,39 | .016 | |
6 | 19,41 | .052 | 16,44 | .010 | |
7 | 20,45 | .048 | 17,48 | .010 | |
8 | 21,49 | .045 | 18,52 | .011 | |
6 | 6 | 26,52 | .041 | 23,55 | .009 |
6 | … . | … . | 24,54 | .015 | |
7 | 28,56 | .051 | 24,60 | .008 | |
7 | … . | … . | 25,59 | .014 | |
8 | 29,61 | .043 | 25,65 | .008 | |
8 | 30,60 | .059 | 26,64 | .013 | |
7 | 7 | 37,68 | .053 | 33,72 | .011 |
8 | 39,73 | .054 | 34,78 | .009 | |
8 | 8 | 49,87 | .050 | 44,92 | .010 |
The procedure we just described is the Mann-Whitney rank-sum test.* The procedure for testing the hypothesis that a treatment had no effect with this statistic is:
- Rank all observations according to their magnitude, a rank of 1 being assigned to the smallest observation. Tied observations should be assigned the same rank, equal to the average of the ranks they would have been assigned had there been no tie (i.e., using the same procedure as in computing the Spearman rank correlation coefficient in Chapter 8).
- Compute T, the sum of the ranks in the smaller sample. (If both samples are the same size, you can compute T from either one.)
- Compare the resulting value of T with the distribution of all possible rank sums for experiments with samples of the same size to see whether the pattern of rankings is compatible with the hypothesis that the treatment had no effect.
There are two ways to compare the observed value of T with the critical value defining the most extreme values that would occur if the treatment had no effect. The first approach is to compute the exact distribution of T by listing all the possibilities, as we just did, then tabulate the results in a table such as Table 10-3. For experiments in which the samples are small enough to be included in Table 10-3 this approach gives the exact P value associated with a given set of experimental observations. For larger experiments this exact approach becomes quite tedious because the number of possible rankings gets very large. For example, there are 184,756 different ways to rank two samples of 10 individuals each.
Second, when the large sample contains more than eight members, the distribution of T is very similar to the normal distribution with mean
and compare this statistic with the critical values of the normal distribution that define the, say 5%, most extreme possible values. zT can also be compared with the t distribution with an infinite number of degrees of freedom (Table 4-1) because it equals the normal distribution.
This comparison can be made more accurate by including a continuity correction (analogous to the Yates correction for continuity in Chapter 5) to account for the fact that the normal distribution is continuous whereas the rank sum T must be an integer
* There is an alternative formulation of this test that yields a statistic commonly denoted by U. U is related to T by the formula U = T − nSnB + nS (nS + 1)/2, where ns is the size of the smaller sample (or either sample if both contain the same number of individuals). For a presentation of the U statistic, see Siegel S, Castellan NJ Jr. The Wilcoxon-Mann-Whitney U test. In: Nonparametric Statistics for the Behavioral Sciences, 2nd ed. New York: McGraw-Hill; 1988:sec 6.4. For a detailed derivation and discussion of the Mann-Whitney test as developed here, as well as its relationship to U, see Mosteller F, Rourke R. Ranking methods for two independent samples. Sturdy Statistics: Nonparametrics and Order Statistics. Reading, MA: Addison-Wesley; 1973:chap 3.
* When there are tied measurements, the standard deviation needs to be reduced according to the following formula, which depends on the number of ties.
Diabetic neuropathy is a painful consequence of diabetes mellitus in which peripheral nerves are damaged, probably because of damage diabetes does to the small blood vessels that supply the nerves. The symptoms vary depending on the specific manifestation of the disease, but can include numbness and tingling in the extremities, uncontrollable muscle contractions and burning or electric pain. In an effort to develop better ways to control this pain, Dinesh Selvarjah and colleagues† conducted a prospective randomized double blind placebo controlled trial of a cannabis-based medicine to investigate whether this medicinal would effectively control the pain associated with diabetic neuropathy.
Experimental subjects were recruited from a diabetes clinic and randomly assigned to either receive the cannabis medicinal or a placebo. The experiment is double blind because neither the experimental subjects nor the investigators knew who was receiving the active medicinal. Including the placebo and blinding the experimental subjects was important not only to avoid the placebo effect, but also to avoid biased reporting of pain, which can be subjective. Likewise, the investigators were also blinded to the subjects’ treatments to avoid biasing the recording and analysis of the pain data. The volunteers in the experiment were treated for 12 weeks, then asked to report their level of pain using a standardized questionnaire.
Figure 10-2 shows the raw data for the 29 people randomized to receive the placebo and the 24 people randomized to receive the medicinal. Even a cursory examination of the data shows that the pain responses are not normally distributed. (We discussed the data for placebo in conjunction with Figure 2-11 and Box 2-1.) Because we cannot assume that the underlying populations from which the data were drawn is normally distributed, we compare these two treatment groups using the Mann-Whitney rank-sum test.
Figure 10-2.

The level of pain reported among people with diabetic neuropathy after 12 weeks of taking a placebo or cannabis medicinal. The experimental subjects did not know which treatment they were receiving. Note that the pain distributions are not symmetrically distributed, but are skewed: most values tend to fall below about 30, but a few people experienced severe pain (high scores).
Table 10-4 shows the observed pain scores as well as the ranks of all the pain scores, without regard for which treatment each person received. All 53 people are ranked as a single group with the person with the lowest pain score ranked 1 and the highest ranked 53. In this case, two people in the placebo group are tied for the lowest pain score, 4, so each receives a rank of 1.5, the average of the first and second ranks. Because three people, two in the placebo group and one on the cannibis group, have the next highest pain score of 7, each receives a rank of 4, the average of the third, fourth, and fifth ranks. The person with the highest pain score, 100, who happens to also be in the placebo group, receives a rank of 53.
Placebo | Cannabis Medicinal | ||
---|---|---|---|
Observation | Rank | Observation | Rank |
13 | 16 | 90 | 50 |
8 | 6.5 | 10 | 9.5 |
46 | 39 | 45 | 38 |
61 | 44 | 70 | 45.5 |
28 | 31.5 | 13 | 16 |
7 | 4 | 27 | 30 |
93 | 51 | 11 | 11 |
10 | 9.5 | 70 | 45.5 |
7 | 4 | 14 | 19 |
100 | 53 | 15 | 20 |
4 | 1.5 | 13 | 16 |
16 | 21 | 75 | 47 |
23 | 27 | 50 | 40 |
33 | 35 | 30 | 34 |
18 | 22 | 80 | 48 |
51 | 41 | 40 | 37 |
26 | 29 | 29 | 33 |
19 | 23.5 | 13 | 16 |
20 | 25.5 | 9 | 8 |
54 | 42 | 7 | 4 |
19 | 23.5 | 20 | 25.5 |
37 | 36 | 85 | 49 |
13 | 16 | 55 | 43 |
8 | 6.5 | 94 | 52 |
28 | 31.5 | ||
25 | 28 | T = 737 | |
4 | 1.5 | ||
12 | 12.5 | ||
12 | 12.5 |
The cannabis medicinal group is the smaller sample, so we compute the test statistic T by summing all the ranks in that group, yielding T = 737. The cannabis group has nS = 24 people in it and the larger placebo group, nB = 29, so the mean value of T for all studies of this size is
This value is smaller than 1.960, the value of z that defines the most extreme 5% of the normal distribution (from Table 4-1). Hence, this study does not provide substantial evidence that the cannabis medicinal was any more or less effective than placebo in controlling pain associated with diabetic neuropathy.
Chapter 9 presented the paired t test to analyze experiments in which each experimental subject was observed before and after a single treatment. This test required that the changes accompanying treatment be normally distributed. We now develop an analogous test based on ranks that does not require this assumption. We compute the differences caused by the treatment in each experimental subject, rank these differences according to their magnitude (without regard for sign), then attach the sign of the difference to each rank, and, finally, sum the signed ranks to obtain the test statistic W.
This procedure uses information about the sizes of the differences the treatment produces in each experimental subject as well as its direction. Since it is based on ranks, it does not require making any assumptions about the nature of the population of the differences the treatment produces. As with the Mann-Whitney rank-sum test statistic, we can obtain the distribution of all possible values of the test statistic W by simply listing all the possibilities of the signed-rank sum for experiments of a given size. We finally compare the value of W associated with our observations with the distribution of all possible values of W that can occur in experiments involving the number of individuals in our study. If the observed value of W is “big,” the observations are not compatible with the assumption that treatment had no effect.
Remember that observations are ranked based on the magnitude of the changes without regard for signs, so that the differences that are equal in magnitude but opposite in sign, say −5.32 and +5.32, both have the same rank.
We begin with another hypothetical experiment in which we wish to test a potential diuretic on six people. In contrast to the experiments the last section described, we will observe daily urine production in each person before and after administering the drug. Table 10-5 shows the results of this experiment, together with the change in urine production that followed administering the drug in each person.
Daily Urine Production (mL/d) | |||||
---|---|---|---|---|---|
Person | Before Drug | After Drug | Difference | Rank* of Difference | Signed Rank of Difference |
1 | 1600 | 1490 | −110 | 5 | −5 |
2 | 1850 | 1300 | −550 | 6 | −6 |
3 | 1300 | 1400 | +100 | 4 | +4 |
4 | 1500 | 1410 | −90 | 3 | −3 |
5 | 1400 | 1350 | −50 | 2 | −2 |
6 | 1010 | 1000 | −10 | 1 | −1 |
W = −13 |
Daily urine production fell in five of the six people. Are these data sufficient to justify asserting that the drug was an effective diuretic?
To apply the signed-rank test, we first rank the magnitudes of each observed change, beginning with 1 for the smallest change and ending with 6 for largest change. Next, we attach the sign of the change to each rank (last column of Table 10-5) and compute the sum of the signed ranks W. For this experiment, W = −13.
If the drug has no effect, the ranks associated with positive changes should be similar to the ranks associated with the negative changes and W should be near zero. On the other hand, when the treatment alters the variable being studied, the changes with the larger or smaller ranks will tend to have the same sign and the signed rank sum W will be a big positive or big negative number.
As with all test statistics, we need only draw the line between “small” and “big.” We do this by listing all 64 possible combinations of different ranking patterns, from all negative changes to all positive changes (Table 10-6). There is one chance in 64 of getting any of these patterns by chance. Figure 10-3 shows all 64 of the signed-rank sums listed in Table 10-6.
Rank* | ||||||
---|---|---|---|---|---|---|
1 | 2 | 3 | 4 | 5 | 6 | Sum of Signed Ranks |
− | − | − | − | − | − | −21 |
+ | − | − | − | − | − | −19 |
− | + | − | − | − | − | −17 |
− | − | + | − | − | − | −15 |
− | − | − | + | − | − | −13 |
− | − | − | − | + | − | −11 |
− | − | − | − | − | + | −9 |
+ | + | − | − | − | − | −15 |
+ | − | + | − | − | − | −13 |
+ | − | − | + | − | − | −11 |
+ | − | − | − | + | − | −9 |
+ | − | − | − | − | + | −7 |
− | + | + | − | − | − | −11 |
− | + | − | + | − | − | −9 |
− | + | − | − | + | − | −7 |
− | + | − | − | − | + | −5 |
− | − | + | + | − | − | −7 |
− | − | + | − | + | − | −5 |
− | − | + | − | − | + | −3 |
− | − | − | + | + | − | −3 |
− | − | − | + | − | + | −1 |
− | − | − | − | + | + | 1 |
+ | + | + | − | − | − | −9 |
+ | + | − | + | − | − | −7 |
+ | + | − | − | + | − | −5 |
+ | + | − | − | − | + | −3 |
+ | − | + | + | − | − | −5 |
+ | − | + | − | + | − | −3 |
+ | − | + | − | − | + | −1 |
+ | − | − | + | + | − | −1 |
+ | − | − | + | − | + | 1 |
+ | − | − | − | + | + | 3 |
− | + | + | + | − | − | −3 |
− | + | + | − | + | − | −1 |
− | + | + | − | − | + | 1 |
− | + | − | + | + | − | 1 |
− | + | − | + | − | + | 3 |
− | + | − | − | + | + | 5 |
− | − | + | + | + | − | 3 |
− | − | + | + | − | + | 5 |
− | − | + | − | + | + | 7 |
− | − | − | + | + | + | 9 |
+ | + | + | − | + | − | 1 |
+ | + | + | − | − | + | 3 |
+ | + | − | + | + | − | 3 |
+ | + | − | + | − | + | 5 |
+ | + | − | − | + | + | 7 |
+ | − | + | + | + | − | 5 |
+ | − | + | + | − | + | 7 |
+ | − | + | − | + | + | 9 |
+ | − | − | + | + | + | 11 |
− | + | + | + | + | − | 7 |
− | + | + | + | − | + | 9 |
− | + | + | − | + | + | 11 |
− | + | − | + | + | + | 13 |
− | − | + | + | + | + | 15 |
+ | + | + | + | + | − | 9 |
+ | + | + | + | − | + | 11 |
+ | + | + | − | + | + | 13 |
+ | + | − | + | + | + | 15 |
+ | − | + | + | + | + | 17 |
− | + | + | + | + | + | 19 |
+ | + | + | + | + | + | 21 |
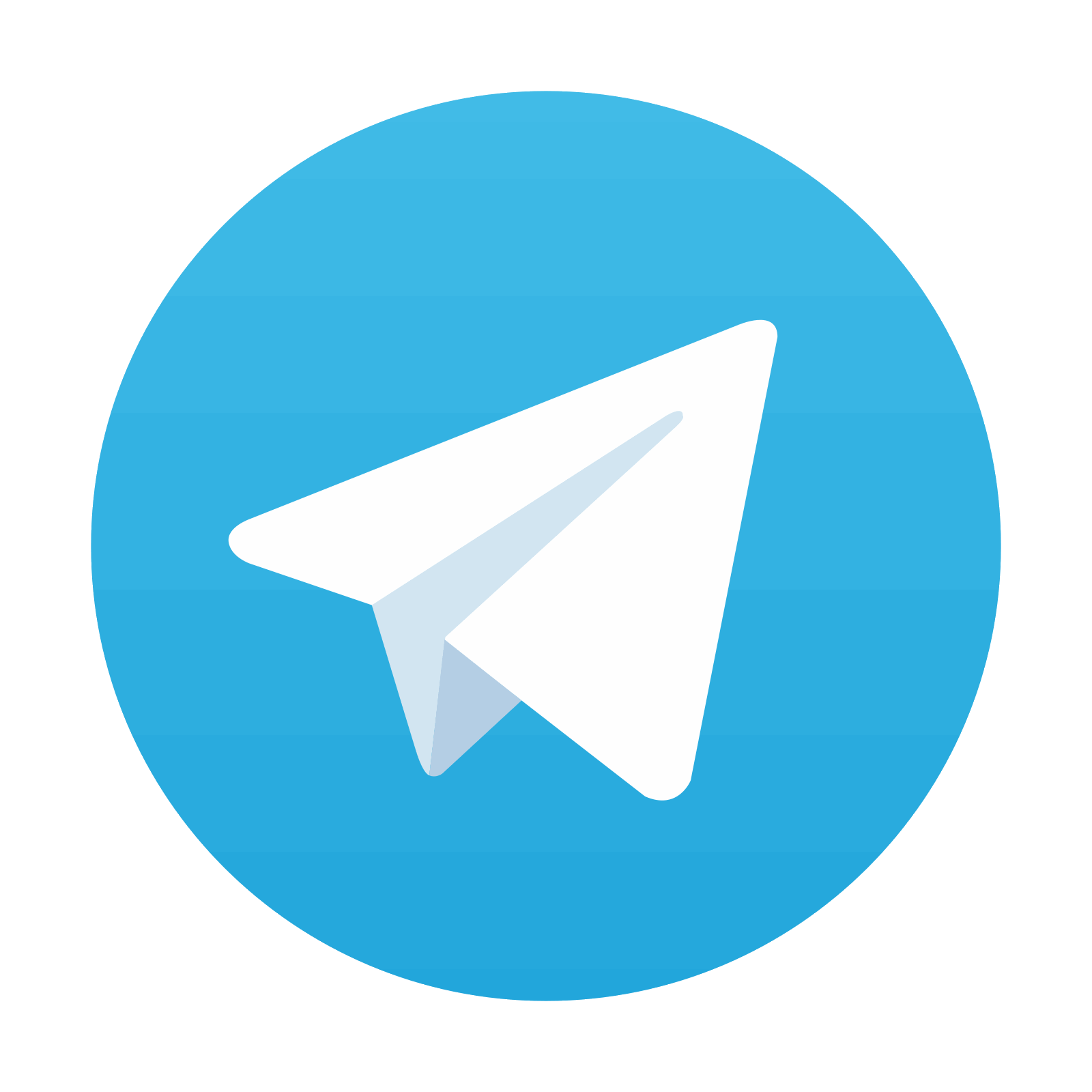
Stay updated, free articles. Join our Telegram channel

Full access? Get Clinical Tree
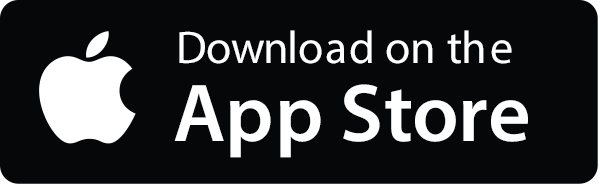

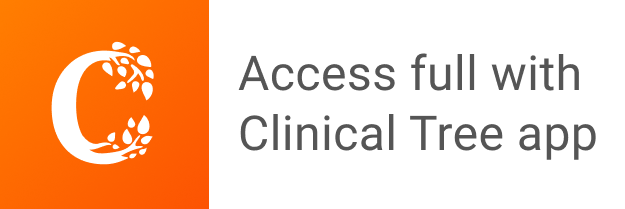