The statistical methods we have been discussing permit you to estimate the certainty of statements and precision of measurements that are common in the biomedical sciences and clinical practice about a population after observing a random sample of its members. To use statistical procedures correctly one needs to use a procedure that is appropriate for the study design and the scale (i.e., interval, nominal, ordinal or survival) used to record the data. All these procedures have, at their base, the assumption that the samples were selected at random from the populations of interest. If the study as conducted does not satisfy this randomization assumption, the resulting P values and confidence intervals are meaningless.
In addition to seeing that the individuals in the sample are selected at random, there is often a question of exactly what actual populations the people in any given study represent. This question is especially important and often difficult to answer when the experimental subjects are patients in academic medical centers, a group of people hardly typical of the population as a whole. Even so, identifying the population in question is the crucial step in deciding the broader applicability of the findings of any study.
Taking all the information we have discussed on cell phones and sperm allows us to confidently conclude that exposure to cell phones adversely affects sperm. We began in Chapter 3 with two human observational studies showing lower sperm motility. The first one* showed a difference between men with lower and higher cell phone use. The second study† improved upon this design by including a true control group of men that did not use cell phones at all as well as including several levels of use and finding a dose–response relationship, with greater reductions in sperm motility associated with increased levels of cell phone use. These two studies, however, were observational, leaving open the possibility that the relationships they elucidated were actually reflecting the effects of some unobserved confounding variable. Concern over confounding variables is especially acute because all the men providing the sperm samples were recruited at fertility clinics, so, even though the investigators tried to screen out men with other reasons for reproductive problems, the possibility remained that something else than exposure to cell phone radiation was causing the reduction in sperm motility.
We increased our confidence that the cell phone radiation was actually affecting sperm when we considered an animal experimental study‡ that showed that rabbits exposed to cell phone radiation had depressed sperm motility. Unlike the earlier two human studies, these results came from an experiment in which the rabbits were randomized to the different treatments and in which the investigators controlled the environment, so we can be much more confident that the results were the result of the cell phone radiation causing the observed changes rather than them being a reflection of some unobserved confounding variable. The issue of interspecies extrapolation, however, remains.
We addressed this issue in Chapter 8 with the experimental study that exposed sperm from normal men to controlled levels of cell phone radiation.§ Because the investigators recruited healthy men as volunteers − not volunteers from men attending a fertility clinic − we can be more confident that the sperm were not behaving abnormally for other reasons. Because the sperm were subjected to controlled irradiation in petri dishes, the experiment avoided the possibility that other aspects of the volunteer men’s behaviors in conjunction with the cell phone use was responsible for the observed effects. The fact that there was a dose-response relationship between the strength of cell phone exposure (measured as specific absorption rate, SAR) and the induction of reactive oxygen species in the sperm, which was, in turn, related to sperm DNA damage, provides a biological mechanism for the changes observed in the original human observational studies. The problem, however, with this experimental study is that sperm in petri dishes may respond differently than sperm in men.
Thus, we are left with the situation in which we have several pieces of evidence on the effects of cell phone exposure on sperm, all of which provide some information, but none of which is definitive and above criticism. The first two studies are realistic because the data come from real people using cell phones in real situations, but they are observational and the fact that the men being studied were attending a fertility clinic could introduce unknown confounding variables. The rabbit study was an experiment, but rabbits are not people. The study of sperm in petri dishes was also an experiment and the sperm were from normal volunteers, but the sperm were irradiated in petri dishes, not people.
The important thing to do is to consider the evidence as a whole. Do all the studies generally point in the same direction? Are they consistent with each other? Do the experimental studies, which almost always are conducted in artificial environments, elucidate the biological mechanisms that explain the observational studies which, while conducted in more realistic environments, suffer from the limitation that they are observational? Conversely, do the observational studies provide results consistent with one would expect based on the biology elucidated in the experiments?
The more of these questions that you can answer “yes,” the more confident that you can be in concluding that the exposure (or treatment) causes the outcome. In this case, we can be very confident that cell phones are causing abnormal sperm behavior**
* Fejes I, Závacki Z, Szöllősi J, Koloszár S, Daru J, Kovács L, Pál A. Is there a relationship between cell phone use and semen quality? Arch Androl. 2005;51:385–393.
† Agarwal A, Deepinder F, Sharma RK, Ranga G, Li J. Effect of cell phone usage on semen analysis in men attending infertility clinic: an observational study. Fertil Steril. 2008;89:124–128.
‡ Salama N, Kishimoto T, Kanayama H. Effects of exposure to a mobile phone on testicular function and structure in adult rabbit. Int J Androl. 2010;33:88–94.
§ De Iuliis GN, Newey RJ, King BV, Aitken RJ. Mobil phone radiation induces reactive oxygen species production and DNA damage in human spermatoza in vitro. PLoS One. 2010;4(7):e6446. doi:10.1371/journal.pone.0006446.
We have reached the end of our discussion of different statistical tests and procedures. It is by no means exhaustive, for there are many other approaches to problems and many kinds of experiments we have not even discussed. Nevertheless, we have developed a powerful set of tools and laid the groundwork for the statistical methods needed to analyze more complex experiments. Table 12-1 shows that it is easy to place all these statistical hypothesis testing procedures this book presents into context by considering two things: the type of experiment or observational study used to collect the data and the scale of measurement.
Study Design | |||||
---|---|---|---|---|---|
Scale of Measurement | Two Treatment Groups Consisting of Different Individuals | Three or More Treatment Groups Consisting of Different Individuals | Before and after a Single Treatment in the Same Individuals | Multiple Treatments in the Same Individuals | Association between Two Variables |
Interval (and drawn from normally distributed populations*) | Unpaired t test (Chapter 4) | Analysis of variance (Chapter 3) | Paired t test (Chapter 9) | Repeated measures analysis of variance (Chapter 9) | Linear regression, Pearson product-moment correlation, or Bland-Altman analysis (Chapter 8) |
Nominal | Chi-square analysis of contingency table (Chapter 5) | Chi-square analysis of contingency table (Chapter 5) | McNemar’s test (Chapter 9) | Cochrane Q† | Relative risk or odds ratio (Chapter 5) |
Ordinal* | Mann-Whitney rank-sum test (Chapter 10) | Kruskal-Wallis test (Chapter 10) | Wilcoxon signed-rank test (Chapter 10) | Friedman test (Chapter 10) | Spearman rank correlation (Chapter 8) |
Survival time | Log rank test or Gehan’s test (Chapter 11) |
To determine which test to use, one needs to consider the study design. Were the treatments applied to the same or different individuals? How many treatments were there? Was the study designed to define a tendency for two variables to increase or decrease together?
How the response is measured is also important. Were the data measured on an interval scale? If so, are you satisfied that the underlying population is normally distributed? Do the variances within the treatment groups or about a regression line appear equal? When the observations do not appear to satisfy these requirements—or if you do not wish to assume that they do—you lose little power by using nonparametric methods based on ranks. Finally, if the response is measured on a nominal scale in which the observations are simply categorized, one can analyze the results using contingency tables. If the nominal dependent variable is a survival time or the data are censored, use survival analysis.
Table 12-1 comes close to summarizing the lessons of this book, but there are three important things that it excludes. First, as Chapter 6 discussed, it is important to consider the power of a test when determining whether or not the failure to reject the null hypothesis of no treatment effect is likely to be because the treatment really has no effect or because the sample size was too small for the test to detect the treatment effect. Second, Chapter 7 discussed the importance of quantifying the size of the treatment effect (with confidence intervals) in addition to the certainty with which you can reject the hypothesis that the treatment had no effect (the P value). Third, one must consider how the samples were selected and whether or not there are biases that invalidate the results of any statistical procedure, however elegant or sophisticated.
It is through these more subtle aspects of the study design that authors (and the sponsors that fund the authors) can manipulate the outcomes of a research paper. Even with correct statistical calculations, an underpowered study will not detect complications in a clinical trial of a new therapy or diseases caused by an environmental toxin such as tobacco smoke or cell phone exposure.* Establishing an inappropriate comparison group can make a test drug look better or worse. When designing or assessing a research, it is important to consider these potential biases, as well as who sponsored the work and the investigators’ relationship with the sponsor.†
* See, for example, Tsang R, Colley L, Lynd LD. Inadequate statistical power to detect clinically significant differences in adverse event rates in randomized clinical trials. J Clin Epidemiol. 2009;62:609–616; Bero LA, Barnes DB. Why review articles on the health effects of passive smoking reach different conclusions. JAMA. 1998;279(19):1566–70; Huss A, Egger M, Huwiler-Müntener K, Röösli M. Source of funding and results of studies of health effects of mobile phone use: systematic review of experimental studies. Environ Health Perspect. 2007;115:1–4.
As already noted, all the statistical procedures assume that the observations represent a sample drawn at random from a larger population. What, precisely, does “drawn at random” mean? It means that any specific member of the population is as likely as any other member to be selected for study and, further, that in an experiment any given individual is as likely to be selected for one sample group as the other (i.e., control or treatment). The only way to achieve randomization is to use an objective procedure, such as a table of random numbers or a random number generator, to select subjects for a sample or treatment group. When other criteria are used that permit the investigator (or participant) to influence which treatment a given individual receives, one can no longer conclude that observed differences are due to the treatment rather than biases introduced by the process of selecting individuals for an observational study or assigning different individuals to different groups in an experimental study. When the randomization assumption is not satisfied, the logic underlying the distributions of the test statistics (F, t, χ2 , z, r, rs , T, W, H, or used to quantify whether the observed differences between the different treatment groups are due to chance as opposed to the treatment fails and the resulting P values (i.e., estimates that the observed differences are due to chance) are meaningless.
To reach meaningful conclusions about the efficacy of some treatment, one must compare the results obtained in the individuals who receive the treatment with an appropriate control group that is identical to the treatment group in all respects except the treatment. Clinical studies often fail to include adequate controls. This omission generally biases the study in favor of the treatment.
Despite the fact that questions of proper randomization and control are really distinct statistical questions, in practice these two areas are so closely related that we will discuss them together by considering two classic examples.
People with coronary artery disease develop chest pain (angina pectoris) when they exercise because the narrowed arteries cannot deliver enough blood to carry oxygen and nutrients to the heart muscle and remove waste products fast enough. Relying on some anatomical studies and clinical reports during the 1930s, some surgeons suggested that tying off (ligating) the mammary arteries would force blood into the arteries that supplied the heart and increase the amount of blood available to it. By comparison with major operations that require splitting the chest open, the procedure to ligate the internal mammary arteries is quite simple. The arteries are near the skin, and the entire procedure can be done under local anesthesia.
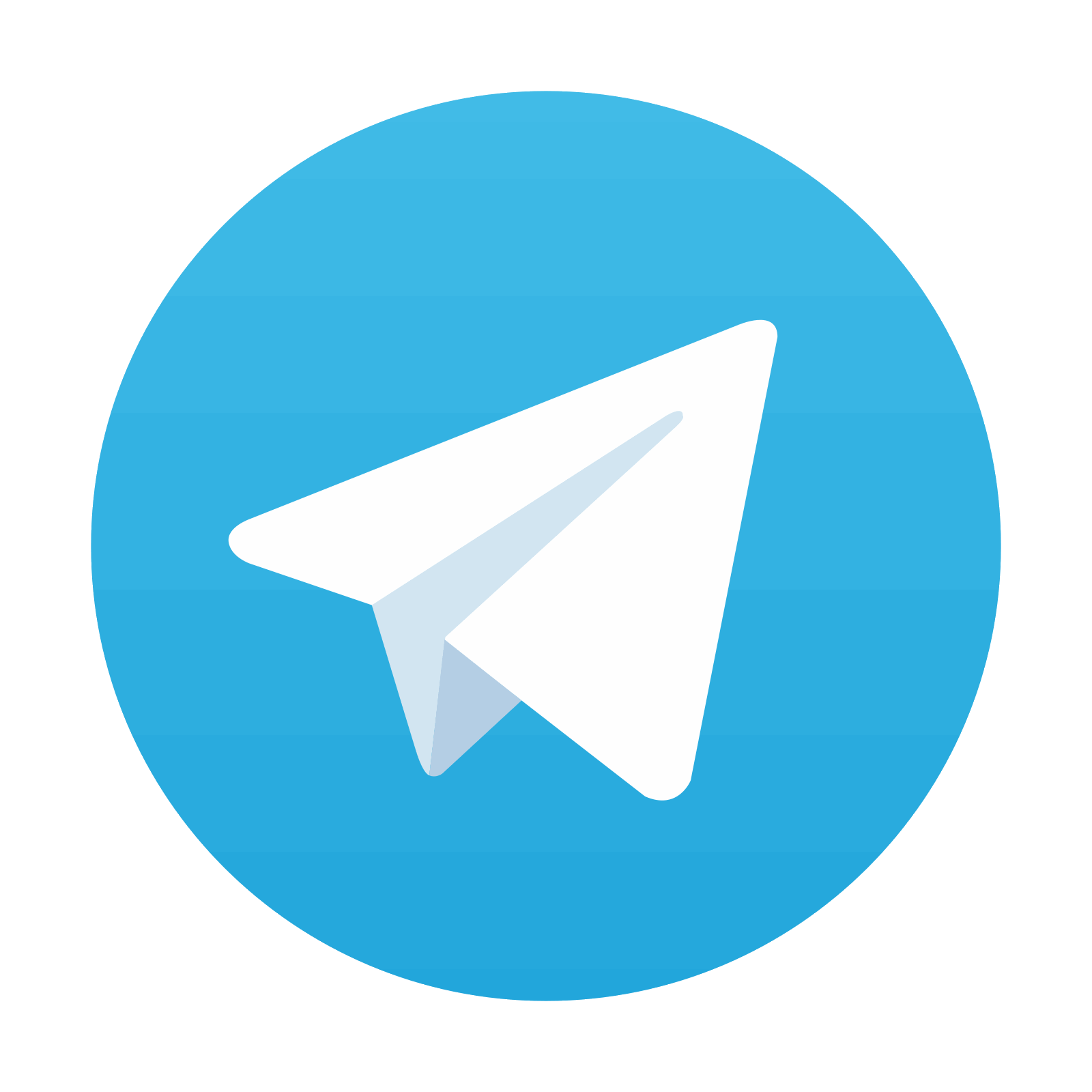
Stay updated, free articles. Join our Telegram channel

Full access? Get Clinical Tree
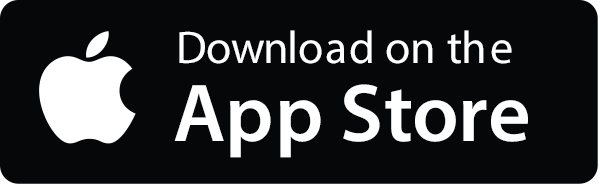
