Abstract
While preoperative testing can provide clinicians with important information into a patient’s suitability for a procedure, there are instances where the tests can inaccurately deliver information depending on the tests themselves. Every test has a unique profile of statistical characteristics (i.e., specificity, accuracy) that may limit its significance and application. When analyzing test results, anesthesiologists must consider the results themselves in the face of the clinical situation. Without correlating the test results to the clinical picture, false-positive and false-negative results can lead to confusion and inappropriate patient care. Knowing the limitations of individual tests can assist in their interpretation, but judiciously ordering tests in appropriate situations can minimize the number of unnecessary tests that can potentially lead to erroneous information.
Keywords
accuracy, false-negative, false-positive, likelihood ratio, predictive value, preoperative test results, sensitivity, specificity
Case Synopsis
A 25-year-old male athlete presents for repair of the anterior cruciate ligament of his knee. He is currently not on any medications. He has a negative personal and family history of abnormal clotting or bleeding or easy bruising. Multiple tests, including prothrombin time and partial thromboplastin time (PTT), are ordered. The PTT results are reported as above normal. The surgery is postponed, and an extensive hematology workup is performed. The final report concludes “normal variant, no coagulation defect.”
Problem Analysis
Definition
A test is useful only to the extent that clinicians (and patients) can understand the implications of a positive or negative result. Few, if any, tests always correctly identify the presence or absence of disease in all patients. Clinicians can decide what to do with a “positive” or “negative” test result only when they have a clear knowledge of the test’s characteristics and its statistical predictive value when applied to a specific patient population. Such “medical decision analysis” directly affects the clinical care of patients.
Some tests provide a qualitative binary positive or negative result. Most test results, however, are quantitative and define a range of “normal” values around a central mean. Therefore members of any population will have an “abnormal” test result but do not actually have a disease. This means that a test result may be misleading owing to natural variability in the general population.
The test can give an incorrect result due to (1) inaccuracy, (2) imprecision, or (3) incorrect performance. The accuracy of a test is the difference between the mean value of test results and the true result, as measured by a gold-standard test. The precision of a test is the reproducibility of results between instruments or persons performing the test. An incorrectly performed test may completely invalidate any result.
Accuracy
Test accuracy can be described in several ways. The sensitivity of a test measures the proportion of individuals who have a disease and are correctly identified as being positive for that disease, based on the test. Specificity measures the proportion of individuals who do not have a disease and are identified as being disease free, based on the test. False-positive results are more likely with tests that have a high sensitivity, low specificity, or both. Sensitivity and specificity are characteristics of the test and do not change with the prevalence (frequency) of disease in the population. In other words, a test’s sensitivity and specificity do not affect the probability of a patient having a disease.
Predictive Value
The predictive value of tests, in contrast, depends on the prevalence of a disease in a population of patients. The predictive value of a positive test indicates the proportion of those with a positive test who actually have the disease. Often, the predictive value of tests is expressed as the probability, or odds, that a condition is present.
Likelihood ratios express the amount that the odds change when the results of the test are available ( Table 20.1 ). In this respect, an important concept is Bayes’ theorem, which “relates the probability of an item (e.g., a patient) being a member of a particular group (e.g., clinical class), given the presence of an attribute (e.g., an abnormal test result), to the probability of known group members having the attribute and the probability of obtaining a group member when picking at random an item from the universe of items.” It allows the calculation of changes in the probability of disease as new information (e.g., test results) becomes available. The “posttest” probability of having the disease in question can be estimated with the Fagan nomogram, as shown in Fig. 20.1 . A web-interactive Fagan nomogram can be accessed at http://araw.mede.uic.edu/cgi-bin/testcalc.pl . There are also several desktop computer as well as mobile apps available for smartphones and tablets to calculate Bayesian analysis. A “two-step” Fagan nomogram, which simplifies estimation by adding lines for diagnostic sensitivity and specificity, can be accessed at http://www.adelaide.edu.au/vetsci/research/pub_pop/2step-nomogram/ .
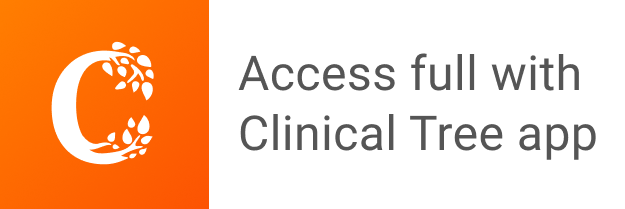