CHAPTER 3 INJURY SEVERITY SCORING: ITS DEFINITION AND PRACTICAL APPLICATION
The urge to prognosticate following trauma is as old as the practice of medicine. This is not surprising, because injured patients and their families wish to know if death is likely, and physicians have long had a natural concern not only for their patients’ welfare but for their own reputations. Today there is a growing interest in tailoring patient referral and physician compensation based on outcomes, outcomes that are often measured against patients’ likelihood of survival. Despite this enduring interest the actual measurement of human trauma began only 50 years ago when DeHaven’s investigations1 into light plane crashes led him to attempt the objective measurement of human injury. Although we have progressed far beyond DeHaven’s original efforts, injury measurement and outcome prediction are still in their infancy, and we are only beginning to explore how such prognostication might actually be employed.
In this chapter, we examine the problems inherent in injury measurement and outcome prediction, and then recount briefly the history of injury scoring, culminating in a description of the current de facto standards: the Injury Severity Score (ISS),2 the Revised Trauma Score (RTS),3 and their synergistic combination with age and injury mechanism into the Trauma and Injury Severity Score (TRISS).4 We will then go on to examine the shortcomings of these methodologies and discuss two newer scoring approaches, the Anatomic Profile (AP) and the ICD-9 Injury Scoring System (ICISS), that have been proposed as remedies. Finally, we will speculate on how good prediction can be and to what uses injury severity scoring should be put given these constraints. We will find that the techniques of injury scoring and outcome prediction have little place in the clinical arena and have been oversold as means to measure quality. They remain valuable as research tools, however.
INJURY DESCRIPTION AND SCORING: CONCEPTUAL BACKGROUND
Injury scoring is a process that reduces the myriad complexities of a clinical situation to a single number. In this process information is necessarily lost. What is gained is a simplification that facilitates data manipulation and makes objective prediction possible. The expectation that prediction will be improved by scoring systems is unfounded, however, since when ICU scoring systems have been compared to clinical acumen, the clinicians usually perform better.4,5
Clinical trauma research is made difficult by the seemingly infinite number of possible anatomic injures, and this is the first problem we must confront. Injury description can be thought of as the process of subdividing the continuous landscape of human injury into individual, well-defined injuries. Fortunately for this process, the human body tends to fail structurally in consistent ways. Le Fort6 discovered that the human face usually fractures in only three patterns despite a wide variety of traumas, and this phenomenon is true for many other parts of the body. The common use of eponyms to describe apparently complex orthopedic injuries underscores the frequency with which bones fracture in predictable ways. Nevertheless, the total number of possible injuries is large. The Abbreviated Injury Scale is now in its fifth edition (AIS 2005) and includes descriptions of more than 2000 injuries (increased from 1395 in AIS 1998). The International Classification of Diseases, Ninth Revision (ICD-9) also devotes almost 2000 codes to traumatic injuries. Moreover, most specialists could expand by several-fold the number of possible injuries. However, a scoring system detailed enough to satisfy all specialists would be so demanding in practice that it would be impractical for nonspecialists. Injury dictionaries thus represent an unavoidable compromise between clinical detail and pragmatic application.
TESTING A TEST: STATISTICAL MEASURES OF PREDICTIVE ACCURACY AND POWER
A final property of a good scoring system is that it is well calibrated, that is, reliable. In other words, a predictive scoring system that is well calibrated should perform consistently throughout its entire range, with 50% of patients with a 0.5 predicted mortality actually dying, and 10% or patients with a 0.1 predicted mortality actually dying. Although this is a convenient property for a scoring system to have, it is not a measure of the actual predictive power of the underlying model and predictor variables. In particular, a well-calibrated model does not have to produce more accurate predictions of outcome than a poorly calibrated model. Calibration is best thought of as a measure of how well a model fits the data, rather than how well a model actually predicts outcome. As an example of the malleability of calibration, Figure 2A and B displays the calibration of a single ICD-9 Injury Severity Score (ICISS) (discussed later), first as the raw score and then as a simple mathematical transformation of the raw score. Although the addition of a constant and a fraction of the score squared add no information and does not change the discriminatory power based on ROC, the transformed score presented in Figure 2B is dramatically better calibrated. Calibration is commonly evaluated using the Hosmer Lemeshow (HL) statistic. This statistic is calculated by first dividing the data set into 10 equal deciles (by count or value) and then comparing the predicted number of survivors in each decile to the actual number of survivors. The result is evaluated as a chi-square test. A high (p>0.05) value implies that the model is well calibrated, that is, it is accurate. Unfortunately, the HL statistic is sensitive to the size of the data set, with very large data sets uniformly being declared “poorly calibrated.” Additionally, the creators of the HL statistic have noted that its actual value may depend on the arbitrary groupings used in its calculation,7 and this further diminishes the HL statistic’s appeal as a general measure of reliability.
The success of a model in predicting mortality is thus measured in terms of its ability to discriminate survivors from nonsurvivors (ROC statistic) and its calibration (HL statistic). In practice, however, we often wish to compare two or more models rather than simply examine the performance of a single model. The procedure for model selection is a sophisticated statistical enterprise that has not yet been widely applied to trauma outcome models. One promising avenue is an information theoretic approach in which competing models are evaluated based on their estimated distance from the true (but unknown) model in terms of information loss. While it might seem impossible to compare distances to an unknown correct model, such comparisons can be accomplished by using the Akaike information criterion (AIC)8 and related refinements.
MEASURING ANATOMIC INJURY
Both the AIS dictionary and the ISS score have enjoyed considerable popularity over the past 30 years. The fifth revision of the AIS9 has recently been published, and now includes over 2000 individual injury descriptors. Each injury in this dictionary is assigned a severity from 1 (slight) to 6 (unsurvivable), as well as a mapping to the Functional Capacity Index (a quality-of-life measure).10 The ISS has enjoyed even greater success—it is virtually the only summary measure of trauma in clinical or research use, and has not been modified in the 30 years since its invention.
Despite their past success, both the AIS dictionary and the ISS score have substantial shortcomings. The problems with AIS are twofold. First, the severities for each of the 2000 injuries are consensus derived from committees of experts and not simple measurements. Although this approach was necessary before large databases of injuries and outcomes were available, it is now possible to accurately measure the severity of injuries on the basis of actual outcomes. Such calculations are not trivial, however, because patients typically have more than a single injury, and untangling the effects of individual injuries is a difficult mathematical exercise. Using measured severities for injuries would correct the inconsistent perceptions of severity of injury in various body regions first observed by Beverland and Rutherford11 and later confirmed by Copes et al.12 A second difficulty is that AIS scoring is expensive, and therefore is done only in hospitals with a zealous commitment to trauma. As a result, the experiences of most non-trauma center hospitals are excluded from academic discourse, thus making accurate demographic trauma data difficult to obtain.
The ISS has several undesirable features that result from its weak conceptual underpinnings. First, because it depends on the AIS dictionary and severity scores, the ISS is heir to all the difficulties outlined previously. But the ISS is also intrinsically flawed in several ways. By design, the ISS allows a maximum of three injuries to contribute to the final score, but the actual number is often fewer. Moreover, because the ISS allows only one injury per body region to be scored, the scored injuries are often not even the three most severe injuries. By considering less severe injuries, ignoring more severe injuries, and ignoring many injuries altogether, the ISS loses considerable information. Baker herself proposed a modification of the ISS, the new ISS (NISS13), which was computed from the three worst injuries, regardless of the body region in which they occurred. Unfortunately, the NISS did not improve substantially upon the discrimination of ISS.
The consequences of these idiosyncrasies for the ISS are severe, as an examination of the actual mortality for each of 44 ISS scores in a large data set (691,973 trauma patients contributed to the National Trauma Data Bank [NTDB]14) demonstrates. Mortality does not increase smoothly with increasing ISS, and, more troublingly, for many pairs of ISS scores, the higher score is actually associated with a lower mortality (Figure 1A). Some of these disparities are striking: patients with ISS scores of 27 are four times less likely to die than patients with ISS scores of 25. This anomaly occurs because the injury subscore combinations that result in an ISS of 25 (5,0,0 and 4,3,0) are, on average, more likely to be fatal than the injury subscore combinations that result in and ISS of 27 (5,1,1 and 3,3,3). (Kilogo et al.15 note that 25% of ISS scores can actually be the result of two different subscore combinations, and that these subscore combinations usually have mortalities that differ by over 20%.)
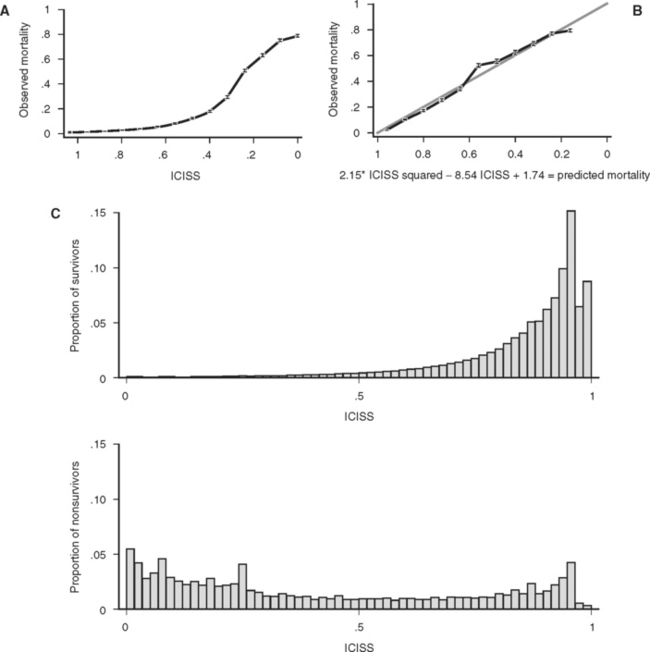
Figure 1 (A) Survival as a function of ICD-9 Injury Scoring System (ICISS) score (691,973 patients from the National Trauma Data Bank [NTDB]). (B) Survival as a function of ICISS score mathematically transformed by the addition of an ICISS2 term (a “calibration curve”). Note that although this transformation does not add information (or change the discrimination [receiver operation characteristic value]) of the model, it does substantially improve the calibration of the model (691,973 patients from the NTDB). (C) ICISS scores presented as paired histograms of survivors (above) and nonsurvivors (691,973 patients from the NTDB).
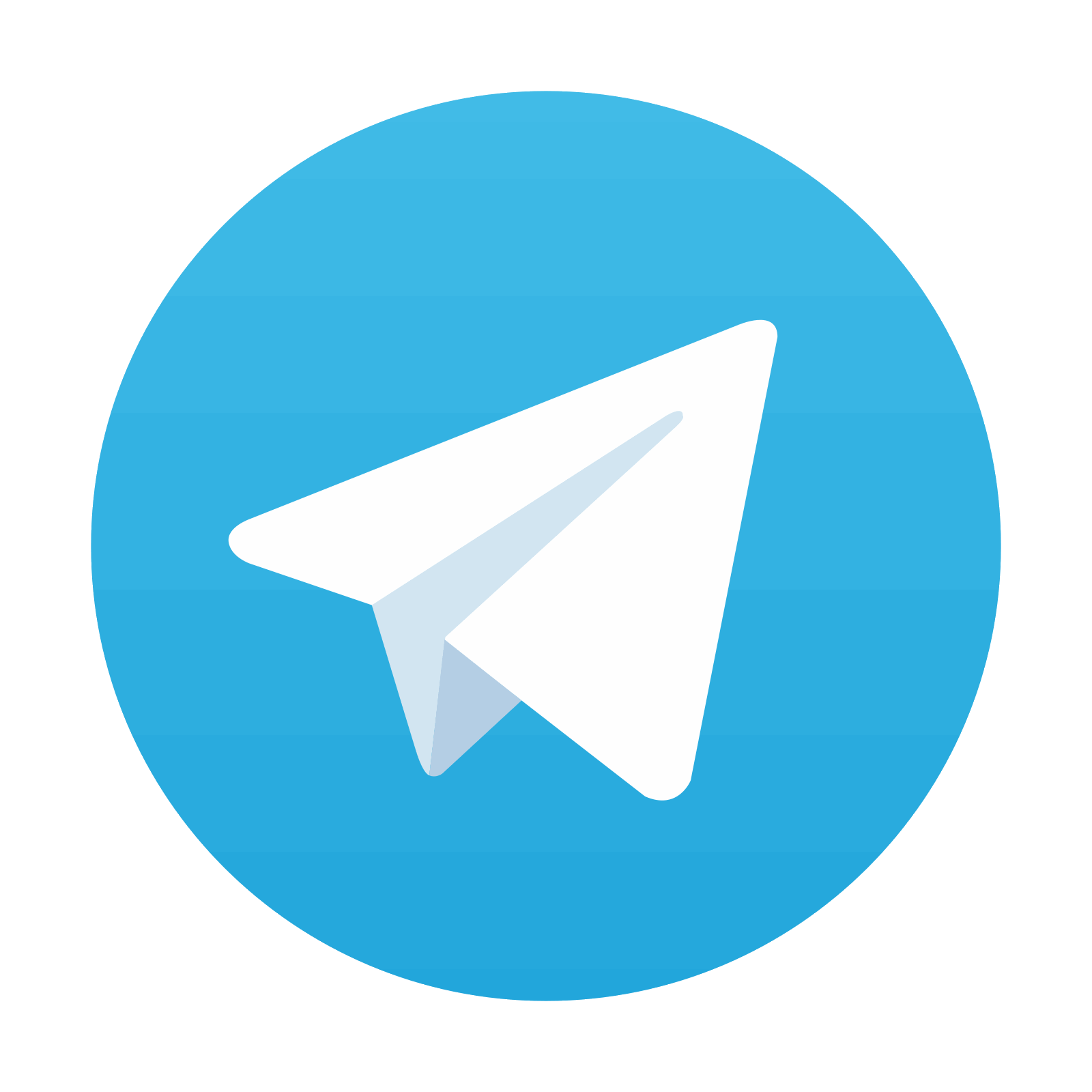
Stay updated, free articles. Join our Telegram channel

Full access? Get Clinical Tree
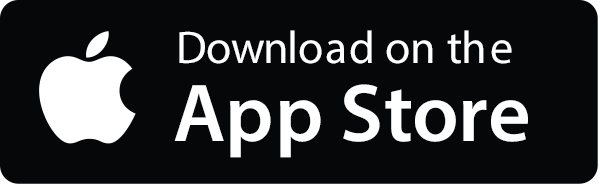
