Key Points
- ▪
Quality needs to be an integral characteristic of the system in which care is delivered. Improving the quality of care often requires reorganization of the way we work. A challenge to the anesthesia team is to combine efficiency in perioperative care (especially the operating room) with safety and the best quality possible.
- ▪
The growing demand from patients, clinicians, insurers, regulators, accreditors, and purchasers for improved quality and safety in health care requires that anesthesiologists and members of the anesthesia team persistently evaluate the quality of care they provide.
- ▪
Improving quality of care requires measuring performance. Clinicians have an enhanced ability to obtain feedback regarding performance in their daily work, in part because of the increasing use of information systems. Unfortunately, consensus has not been reached on how to measure quality of care.
- ▪
The goal of measurement is to learn and improve. The measurement system must fit into an improvement system; clinicians must have the will to work cooperatively to improve, and they must have ideas or hypotheses about changes to the current system of care. Also, the clinical team must have a model for testing changes and implementing those that result in improvements.
- ▪
Outcome measures, including in-hospital mortality rates, have been the basis for evaluating performance and quality. However, hospital mortality alone provides an incomplete picture of quality, does not include all domains of quality, and does not measure the overall success of the full cycle of care for a specific medical condition. A balanced set of structures (how care is organized), processes (what we do), and outcome measures (results of care in terms of patient’s health over time) is needed to evaluate the quality of care overall.
- ▪
Efforts to improve quality of care require development of valid, reliable, and practical measures of quality. Identification of clinical care that truly achieves excellence would be helpful not only to the administration of anesthesia, but also to health care overall.
- ▪
Developing a quality measure requires several steps: prioritizing the clinical area to evaluate; selecting the type of measure; writing definitions and designing specifications; developing data collection tools; pilot-testing data collection tools and evaluating the validity, reliability, and feasibility of measures; developing scoring and analytic specifications; and collecting baseline data.
- ▪
The best opportunities to improve quality of care and patient outcomes will most likely come not only from discovering new therapies, but also from discovering how to better deliver therapies that are already known to be effective.
- ▪
Safety is an integral part of quality that is focused on the prevention of error and patient harm. The airline industry is often lauded as an exemplar of safety because it has embraced important safety principles, including the standardization of routine tasks, the reduction of unnecessary complexity, and the creation of redundancies. Anesthesia care teams have also adopted these principles, although many opportunities remain to further bolster patient safety.
- ▪
Healthcare providers can organize their quality improvement and patient safety efforts around three key areas: (1) translating evidence into practice, (2) identifying and mitigating hazards, and (3) improving culture and communication. Although each of these areas requires different tools, they all help health care organizations evaluate progress in patient safety and quality.
Acknowledgments
The editors and the publisher would like to acknowledge Dr. Elizabeth Martinez (b. 1966–d. 2013) for her contributions to previous editions of this chapter. Her work served as the foundation for the current chapter.
This chapter greatly benefited from the thoughtful editing of Claire Levine.
The need for improving quality and reducing the cost of health care has been highlighted repeatedly in the scientific literature and lay press. Improving care, minimizing variation, and reducing costs have increasingly become national priorities in many countries. Quality improvement (QI) programs that address these issues not only improve delivery of care but also have a positive effect on practitioner job satisfaction and organizational commitment.
The goal of this chapter is to present a practical framework for developing and implementing QI programs in anesthesiology and critical care medicine that are both scientifically sound and feasible. To accomplish this goal, we review the science and approaches to QI, present measures that help evaluate whether QI programs have resulted in improvements, and describe examples of successful QI efforts.
What Is Quality?
Definition of Quality
W. Edwards Deming, scholar, professor, author, lecturer, and consultant to business leaders, corporations, and governments defined quality as “a predictable degree of uniformity and dependability with a quality standard suited to the customer.” This early definition of quality, in the context of QI, stems from its application to industrial production. However, when the term quality is applied to health care, the subtleties and implications of treating a human being are of prime importance, as opposed to the concerns involved in producing consumer goods. Use of the term quality in the context of health care can sometimes lead to defensive attitudes, economic concerns, and even ethical debates.
In the healthcare sector, quality can have various meanings to different people. For example, a daughter may evaluate quality by the level of dignity and respect with which her elderly mother is treated by a nurse. A cardiac surgeon may see quality as a percentage of improvement in the function of a heart on which he or she has just operated. A business may judge quality by the timeliness and cost effectiveness of the care delivered to its employees and its effect on the bottom line. Finally, society may evaluate quality by the ability to deliver care to those who need it, regardless of their cultural or socioeconomic backgrounds.
Despite the numerous definitions of quality in both business and medicine, a unified definition of quality in the context of QI should exist in health care. This definition of quality may have implications for both its measurement and its improvement. In order to help standardize the definition of quality in health care, the Institute of Medicine (IOM) published its own definition in a 1990 report titled Medicare: A Strategy for Quality Assurance. The IOM, which has since been renamed the National Academy of Medicine (NAM), defined quality as “the degree to which health services for individuals and populations increase the likelihood of desired health outcomes and are consistent with current professional knowledge.” Inherent in this definition are the elements of measurement, goal orientation, process and outcomes, individual and society preferences, and a dynamic state of professional knowledge. This definition of quality in health care has gained widespread acceptance. A similar definition is offered by the U.S. Government Department of Health and Human Services, which defines quality in public health as “the degree to which policies, programs, services, and research for the population increase desired health outcomes and conditions in which the population can be healthy.”
Aims of Quality in Health Care
In the 2001 report, Crossing the Quality Chasm, six aims for quality in health care were outlined . These aims of safety, effectiveness, patient-centeredness, timeliness, efficiency, and equity included and extended the issues of patient safety described in their earlier report To Err Is Human. The aims have been adopted by many organizations, including the Institute for Healthcare Improvement (IHI), a United States nongovernmental agency devoted to advancing QI and patient safety in health care. These aims serve as a basis on which quality is evaluated and improved and are described as follows.
- 1.
Safety. No patient or healthcare worker should be harmed by the healthcare system at any time, including during transitions of care and “off hours,” such as nights or weekends. Errors may be categorized as either failure of an action to occur as planned, such as the administration of a wrong medication to a patient, or having the wrong plan altogether, such as misdiagnosing and subsequently mistreating a patient. As much as possible, patients should be informed about the risks and benefits of medical care in advance. If a complication does occur, medical staff should make full disclosure, provide assistance to the patient and family, and exercise due diligence in preventing any recurrences of the error.
- 2.
Effectiveness. Effective medicine requires evidence-based decisions about treatment for individual patients, when such evidence exists. The best available evidence should be combined with clinical expertise and patient values in forming a treatment plan. With effective care, medical practitioners avoid underuse by providing a treatment to all who will benefit and avoid overuse by refraining from giving treatment to those unlikely to benefit.
- 3.
Patient-centeredness. Patient-centered care is respectful of individual patient preferences, needs, and values and uses these factors to guide clinical decisions. More specifically, according to Gerteis and colleagues, patient-centered care encompasses respect for patients’ values; coordination and integration of care; information, communication, and education; physical comfort; emotional support that relieves fear and anxiety; and involvement of family and friends. The dramatic increase in access to health information on the Internet has resulted in more patients who are well informed and proactive in their care. Patient-centered care embraces this trend and shifts more of the power and control to patients and their families. Examples of patient-centered care include shared decision making, patient and family participation in rounds, patient ownership of medical records, schedules that minimize patient inconvenience, and unrestricted visitation hours.
- 4.
Timeliness. Reduced wait time is important to both patients and healthcare practitioners. Long waits signal a lack of respect for a patient’s time. Furthermore, delays may not only affect patient satisfaction, but may impair timely diagnosis and treatment. For healthcare workers, delays in availability of equipment or information may decrease job satisfaction and the ability to perform their jobs adequately.
- 5.
Efficiency. Rising costs have increased scrutiny of waste in health care; this includes waste in labor, capital, equipment, supplies, ideas, and energy. Improved efficiency reduces waste and results in an increased output for a given cost. Examples of efficiency measures include mean length of hospital stay, readmission rate, and mean cost of treatment for a diagnosis. The elimination of waste can result in better quality of care for patients at the same or lower cost.
- 6.
Equity. Equitable care does not vary in quality based on personnel. The NAM defines equitable care at two levels. At the population level, equitable care means reducing or eliminating disparities between subgroups. At the individual level, it means absence of discrimination based on factors such as age, gender, race, ethnicity, nationality, religion, educational attainment, sexual orientation, disability, or geographic location.
Another framing of quality is the “quadruple aim” proposed by Bodenheimer and Sinsky and adopted by the IHI. These four aims include better care, better outcomes, lower cost, and better work life for the healthcare workforce. This last aim was added to the IHI’s previous “Triple Aim” in recognition that increasing clinician burnout represents a threat to high-quality care.
Deming’s System of Profound Knowledge
Before learning about frameworks and tools for improvement, it helps to have an understanding of the theory behind improvement work. W. Edwards Deming wrote about two different types of knowledge: subject matter knowledge and profound knowledge. Subject matter knowledge is professional expertise, such as expertise in anesthesiology. Profound knowledge is the knowledge of improvement. The most significant improvement occurs where these two types of knowledge overlap. Deming divides profound knowledge into four different categories: appreciation of a system, the theory of knowledge, understanding variation, and psychology.
The first area of profound knowledge is appreciation of a system. A system is a network of interdependent components working together for a common aim. It is often said that “Every system is perfectly designed to get the results it gets.” If a system is underperforming, it is because it has unintentionally been designed to underperform. If this is the case, it is our responsibility to manage the system to get the results we want.
The second part of Deming’s profound knowledge is the idea that knowledge requires a theory. Information by itself is not knowledge. For example, a dictionary contains information, but it is not knowledge. We must have a theory behind our improvement work, not just data, if we are going to learn.
In order to learn, we must additionally understand variation and how to react to it. Deming says that “life is variation.” Common cause variation is variation that is inherent to the process. Special cause variation is variation from causes that are not inherent to the process but arise from specific circumstances. A process which only has common cause variation is in statistical “control.” On the other hand, a process that has both common cause and special cause variation is an unstable process. Two common errors in improvement work are acting upon common cause variation as if it were special cause, and acting upon special cause variation as if it were common cause.
The last area of profound knowledge is psychology. This is often the most challenging part of improvement work. Deming believed in intrinsic motivation, and the need to nurture people’s joy in work and intrinsic motivation to learn. More recently John P. Kotter describes eight steps to change in his book The Heart of Change . These are increase urgency, build the guiding team, get the vision right, communicate for buy-in, empower action, create short-term wins, don’t let up, and make change stick.
Approaches to Quality Assessment
Quality Assurance Versus Continuous Quality Improvement
Although the terms continuous quality improvement (CQI) and quality assurance (QA) were used interchangeably in the past, substantial differences existed between the two. Most medical CQI systems were built on the foundation of a traditional QA system that used standards to define quality. Standards can be defined as an “acceptable” level of performance. For example, a standard for overall mortality after cardiac surgery is less than 3%; however, is 3% (vs. 4% or 2%) mortality after cardiac surgery acceptable? Similarly, a standard for head injury evaluation is a computerized tomography (CT) brain scan within 4 hours of admission, but in certain circumstances, patients with head injury may warrant a CT scan sooner than that.
Most standards are inherently arbitrary and often lack consensus among medical professionals. Additionally, QA systems typically react only when a standard is not met. Examples of traditional standard-based QA systems were peer review systems and morbidity and mortality reviews. These systems often exist to flag certain cases or practitioners for intense review. Practitioners may regard this intense review as a punishment because only “failures” or “bad apples” are identified, and process failures are not connected with the outcome on every case. Thus, QA systems are inherently judgmental and, if not carefully administered, can hold practitioners responsible for random causes over which they have no control. CQI systems, on the other hand, recognize that errors occur and require different responses. Often excellence in health care is not identified by analysis of QA systems. Excellence is sometimes defined by the lack of failure. Is there a difference between good (acceptable) and excellent health care?
Systems within health care are a series of interlinked processes, each of which results in one or more outputs. CQI systems, as opposed to QA systems, include an explicit approach to process and the use of specifications to improve a process or outcome. A specification is an explicit, measurable statement regarding an important attribute of a process or the outcome it produces. Specifications identify variables that need to be measured, but typically do not set acceptable limits or standards. Once specifications have been defined in a CQI system, all outputs or cases, not just failures, are evaluated against these specifications. The system then attempts to correct errors by fixing the process rather than the people. Thus, CQI aims to change the process and prevent quality failures before they happen by building improvements into the process. To quote Philip Crosby, “The system for causing quality is prevention, not appraisal.”
Frameworks for Improvement
Model for Improvement
The journey toward improvement can be made more efficient and more effective with a systematic approach. The Model for Improvement, developed by the training and management consulting company Associates in Process Improvement ( http://www.apiweb.org ), is one such approach adopted by organizations across varied disciplines and is currently the approach used by IHI. It is a structured, dynamic model that applies the scientific method to testing and implementing a change. In 1939, Walter A. Shewhart, a physicist, engineer, and statistician, introduced the science of modern QI. He introduced a three-step scientific process of specification, production, and inspection, stating that “these three steps must go in a circle instead of in a straight line.” In the 1940s, his protégé, W. Edwards Deming, applied these concepts to government and industry and developed the Plan, Do, Study, Act (PDSA) cycle ( Table 5.1 ). A modification of the PDSA by the addition of three fundamental questions (as explained in the next paragraph) resulted in the Model for Improvement ( Fig. 5.1 ).
Step | Description |
---|---|
Plan | Make a plan for the test of change. Include predictions of results and how data will be collected. |
Do | Test change on a small scale. Document data, observations, and problems that occur. |
Study | Use data gathered from previous stages to build new knowledge and make predictions. Knowledge is gained from both successful and unsuccessful changes. |
Act | Adopt the change, or use knowledge gained to plan or modify the next test of action. |

Beginning an improvement project with the three fundamental questions for improvement helps set a clear direction for the project, define what success will look like, and hypothesize successful interventions. The three fundamental questions for improvement are:
- 1.
Aim: “What are we trying to accomplish?” The aim (or objective) for improvement should be specific, measurable, actionable, relevant, and time-specific (also referred to as a SMART aim). Ideas for improvements may come from interviewing those involved or affected by the process, such as staff or patients. Ideas may also come from examining previous data on operational, clinical, or financial processes.
- 2.
Measure: “How will we know if change is an improvement?” Ideally, measures should be linked directly to the aim or goal of the project and should ensure that the interests of the stakeholders of the process are represented. Quantitative measures should be used when possible to measure change over time. These measures provide the feedback that enables one to know whether or not the change is an improvement. However, not all projects have an easily quantifiable outcome and the outcome may be more qualitative. It is worth the time and effort to identify opportunities to translate goals into quantifiable outcomes if possible. These can be easier to use to communicate success.
- 3.
Changes: “What changes can we make that will result in improvement?” Ideas for changes that result in improvement often start with observations, modeling the success of others, and brainstorming. The more intimate the understanding of a process and its key drivers, the higher the likelihood of generating successful changes.
The three fundamental questions are followed by a PDSA cycle, which is the framework for testing and implementing previously generated ideas for change. Improvement may require multiple cycles of preferably small tests of change over time. By testing changes on a small scale before implementation, risk is mitigated. Small tests of change may also help overcome individuals’ resistance to change. Through repeated cycles, increased knowledge is acquired, and actions are continuously modified or changed. Measures defined in the first part of the model help determine whether or not a change is a success. These measures are often plotted over time on run charts or control charts ( Figs. 5.2 and 5.3 ). Knowledge can be gained from both successful and unsuccessful tests! Finally, PDSA cycles both test a change and implement a successful change on a larger scale or in diverse clinical areas.
Lean Methodology and Six Sigma
In addition to the Model for Improvement, CQI initiatives have many other frameworks. Two of these frameworks, Lean Production and Six Sigma, are briefly discussed here. These frameworks are sometimes combined, as in “Lean Six Sigma.” Regardless of which framework is employed, benefits are gained by retaining a structured and consistent approach to CQI.
Lean methodology has its roots in Japanese manufacturing, particularly in the Toyota Production System. More recently, Lean has found success in the healthcare industry. Two notable examples of its use are Virginia Mason Medical Center and ThedaCare, Inc., both of which have transformed their organizations through the application of Lean principles. In fact, ThedaCare reported $3.3 million in savings in 2004 with reduced accounts receivable, redeployed staff, reduced phone triage times, reduced time spent on paperwork, and decreased medication distribution time.
Lean methodology is focused on creating more value for the customer (i.e., the patient) with fewer resources. Every step in a process is evaluated to differentiate those steps that add value from those that do not. The ultimate goal is to eliminate all waste so that every step adds value to a process. Other key components of Lean include reducing unevenness of workflow—for instance, what we might find in intensive care unit (ICU) admissions or emergency cases—and eliminating the overburdening of people and equipment. Five principles govern Lean improvement:
- 1.
Define the value that the customer is seeking. Virginia Mason Medical Center has a “patient-first” focus for all its processes.
- 2.
Identify and map the value stream. If evaluating preoperative assessment, map the physical flow of a patient from the scheduling of a procedure through the day of surgery (history and physical, preoperative counseling, laboratory tests, imaging, consultations). In this process, all of the steps are accounted for, including the back-and-forth flow of the patient to the front desk, to the laboratory, and so on. Time spent during each step of the process should be documented.
- 3.
Smooth the flow between value-added steps. Eliminate steps that do not add value to the overall process and are likely a poor use of time or effort on the part of the caregivers or the patient. An example of this process might be eliminating unnecessary tests or consultations in a patient’s preoperative evaluation and reducing excess wait times that are the result of correctable inefficiencies.
- 4.
Create pull between steps. Customer demand should trigger the start of a downstream process. Examples include opening operating rooms (ORs) or increasing staffing based on surgical demand, as opposed to having a fixed amount of time for each surgeon or surgical division.
- 5.
Pursue perfection by continuing the process until you have achieved ultimate value with no waste.
The transformation of Motorola in the 1980s from a struggling company to a high-quality, high-profit organization helped give rise to the Six Sigma methodology. Two key fundamental objectives of Six Sigma are a virtually error-free process and a large focus on reducing variation. In fact, a Six Sigma process, or a process whose frequency falls six deviations from the mean, corresponds to just 3.4 errors per million.
Health care often falls far short of this standard. In a 1998 report, Chassin reported that hospitalized patients harmed by negligence were at a four sigma level (10,000/million), patients inadequately treated for depression were at a two sigma level (580,000/million), and eligible heart attack survivors who failed to receive β-adrenergic blockers were at a one sigma level (790,000/million). In contrast, Chassin found that anesthesiology was the one healthcare specialty that approached the six sigma level, with deaths caused by anesthesia as low as 5.4/million. In comparison with health care, airline fatalities were a two sigma process (230/million) and a traditional company operated around four sigma, the equivalent of 6200 errors/million. Considering that errors are often tied directly to cost, this error rate has significant financial implications.
Six Sigma is similar to the Model for Improvement in that it makes use of a simple framework to guide improvement, in this case using Define, Measure, Analyze, Improve, Control (DMAIC). The DMAIC steps are described in Table 5.2 . As mentioned earlier, many organizations have found the greatest benefit by combining elements of different methodologies in their CQI work. One popular example of this is Lean Six Sigma, which combines improvements in flow and value with reduction in error and variation. Furthermore, individual tools from these strategies, such as PDSA cycles or DMAIC processes, can be applied where appropriate.
Step | Description |
---|---|
Define | Define the goals of the improvement project. Obtain necessary support and resources and put together a project team. |
Measure | Establish appropriate metrics. Measure baseline performance of the current system. |
Analyze | Examine the system for possible areas of improvement. |
Improve | Improve the system through implementation of ideas. Statistically validate improvements. |
Control | Institutionalize the new system and monitor its stability over time. |
The Value Framework in Health Care
Since quality in health care is focused on patient outcomes, another approach to quality is the value framework. Quality relative to cost determines value. Hence, in health care, value is defined as the patient health outcomes achieved per dollar spent. Value should define the framework for performance improvement in health care. Value includes goals already embraced by health care such as quality, safety, patient centeredness, and cost containment; the value framework allows for a way to integrate these goals.
Because value is always defined around the customer, in the healthcare industry it is what matters most to patients, and unites the interests of all the stakeholders in the healthcare system. Thus, when value improves, not only do patients, payers, providers, and suppliers all benefit, but the economic sustainability of the healthcare system also improves. As such, value should be the overarching goal of healthcare delivery. According to Porter, the failure to adopt value as the central goal in health care and the failure to measure it, are the most serious failures of the medical community.
Value measurement today is limited and highly imperfect. Value should be measured by outputs not inputs. Thus, value is dependent on patient health outcomes and not the volume of services delivered. The only way to accurately measure value is to track individual patient outcomes and costs longitudinally over the full cycle of care, which can vary from 30 to 90 days for hospital care and 1 year for chronic care.
Value is not measured by processes of care utilized by a patient. While process measurement is an important component of improvement, it should not be substituted for measurement of patient outcomes. Outcomes and cost should be measured separately. Outcomes, the numerator of the value equation, refer to the actual results of care in terms of patient health and should consist of a set of multidimensional outcomes that, when considered together, constitute patient benefit. Cost, the denominator of the value equation, should include total costs involved in the full cycle of care for the patient’s medical condition. Most physicians do not know the full costs of caring for a patient, thus they lack the information to make real efficiency improvements.
Outcomes measurement is critical to driving rapid improvements in health care. Without a feedback loop that includes the outcomes achieved, providers lack the information they require to learn and improve. Effective outcome measurement is hampered by several problems. First, there is a lack of consensus as to what constitutes an outcome. Second, electronic medical record (EMR) systems often do not facilitate the capture of longitudinal outcomes measures with appropriate scope; these systems may focus too narrowly or too broadly, giving only a partial view of patient outcomes. Third, outcomes such as infection rates may vary substantially by medical condition. Finally, true outcome measurement has been limited because the cost of gathering longitudinal patient results is high, due in part to fragmented organizational structures and poor EMR interoperability.
Cost is the most pressing issue in health care. Current cost measurement approaches have not only hampered our understanding of costs but also contributed to approaches involving cost-containment. A focus on cost-containment rather than value improvement can be dangerous and is often self-defeating. Two major problems associated with cost measurement include: (a) cost aggregation, wherein we often measure and accumulate costs based on how care is organized and billed for, that is, costs for departments, discrete service areas, and line items such as supplies or drugs and (b) cost allocation where the costs of healthcare delivery are shared costs, involving shared resources and as such are normally calculated as the average cost over all patients for a department. A good example of this is the hourly charge for the OR. However, to truly understand costs, they must be aggregated around the patient, rather than discrete services, and shared costs must be allocated to individual patients on the basis of each patient’s actual use of the resources involved. Finally, the perspective used to calculate costs matters and patient costs including lost work may not be included in the analysis.
Proper measurement of outcomes and costs is the single most powerful lever for improving healthcare delivery and although current measurements are highly imperfect, the process of measurement has begun. As Michael Porter outlines in the framework papers underpinning his value commentary in the New England Journal of Medicine , if all the stakeholders in health care were to embrace value as the central goal and measure it, the resulting improvements would be enormous.
Quality Improvement Measures and Tools
The concept of using measurement to drive improvement has its origins in both medicine and industry. The use of data to improve patient health originated in the mid-1800s with two pioneers, Florence Nightingale and John Snow. Nightingale used data on mortality among British soldiers to drive improvements in sanitation in field hospitals. Similarly, Snow used data on the incidence and geographic location of cholera to make the connection between the incidence of the disease and water obtained from the Broad Street water pump. In the early 20th century, Ernest Codman, a surgeon at Massachusetts General Hospital, was the first to advocate tracking of patient outcomes so that adverse events could be identified and improvements in care made for future patients. In the 1960s, Avedis Donabedian emphasized the importance of measurement and described a model for evaluating quality of health care based on structure, process, and outcomes— structure being the environment in which health care is provided, process being the method by which it is provided, and outcomes being the result of the care provided. More recently, in 1991, Paul Baltaden and Don Berwick developed the IHI, which has become one of the leading organizations in the application of improvement science to health care.
In QI, measurement can serve many purposes. It can be used to identify problems and establish baseline performance, inform and guide QI projects, select and test changes for improvement, and assess and align progress with organizational goals. Selecting and developing measures that are useful can be challenging. Optimal measures must be comprehensive, carefully defined, tailored to the target audience, and involve minimal measurement burden. Target audiences usually include clinical staff, so measures should address and align with clinical targets for specific patient populations with whom the staff work. Measures should pass the face validity test with clinical practitioners delivering care. National or organizational measures, if applicable, can also be used, but they may not always be relevant or credible to the local target audience. Within an organization, target audiences should include system leaders, so measures should also align with organizational priorities and strategic goals.
Process and Outcome Measures
Measures should include the following:
- 1.
Process measures that address the processes of healthcare delivery (e.g., perioperative β-adrenergic blocker administration for patients, antibiotic administration for prevention of surgical site infection)
- 2.
Outcome measures that address patient outcomes from delivery of these services, such as clinical and functional outcomes or satisfaction with health (e.g., morbidity, mortality, length of stay, quality of life)
- 3.
Balancing measures that address the possible consequences of changes in the process (e.g., when process improvements are made to improve efficiency, other outcomes, such as patient satisfaction, should not be adversely affected)
Each of these measures has advantages and limitations. A comprehensive set of measures should include at least one process, outcome, and balancing measure. In addition, structural measures, such as ICU nurse-to-physician staffing ratios, can be important to include when appropriate.
Healthcare providers readily accept process measures because they demonstrate the degree to which caregivers can influence a process with the intention to improve patient outcomes. Practitioners generally feel more accountable for the process of care than its outcomes, because outcomes may be affected by many other variables. An obstacle to using process as a measure of quality is sustainability; frequent updating is required as the science of medicine advances.
Process measures, which evaluate how care is delivered, may be easier to measure and implement than outcome measures and can provide important insight into care. Process measures can provide immediate feedback regarding performance, allowing for rapid improvements in care. If an outcome occurs infrequently, providers will be unable to obtain meaningful feedback on outcomes on a timely basis. For example, evidence of improved rates of catheter-related bloodstream infections (CRBSI; an outcome measure) may require 12 to 24 months of data (because few patients develop infections), whereas improved adherence to evidence-based practices to reduce infections (process measures) may be observed within a week (because all patients can be evaluated to determine whether they received the intervention).
Process measures have two other important advantages. First, they generally have face validity for providers, meaning that providers believe they can use the data to improve care; and second, because risk adjustment is less important, broad implementation is feasible. Moreover, joint efforts among providers, professional societies, and external government or payer agencies have made process measures more feasible.
To be valid, process measures should have causal links to important outcomes; a change in the process should produce a desired change in outcome. One of the best opportunities to improve patient outcomes may well come from discovering how to deliver therapies (processes) that are known to be effective in producing a desired outcome. For example, hand hygiene and application of chlorhexidine to sterilize the skin site before insertion of a central venous catheter (CVC) are two of five processes known to reduce CRBSI. Process measures such as these are indicators of whether patients reliably receive evidence-based interventions known to prevent complications.
Although process measurement is useful and should continue, there is no substitute for measuring outcomes, whose principal purpose is not comparing providers but enabling innovations in care. Process measurement should largely be an internal effort, but should not be the means of external measurement and reporting of quality and value. As mentioned above, measuring value requires measuring actual outcomes over time.
Outcome measurement refers to the actual results of care in terms of patient health over time; for each medical condition there is a set of multidimensional outcomes that together constitute patient benefit. These include survival, functional status, and sustainability of recovery. Outcome measures relate directly to the health status of the patient. Patient satisfaction with care is a process measure, not an outcome. Patient satisfaction with health is an outcome measure. However, current measures for outcome often focus on the immediate results of particular procedures or interventions rather than on the overall success of the full cycle of care of a medical condition.
The relative focus on outcome and process measures will depend on balancing the collection of data between that which is scientifically sound and that which is feasible. In general, a balanced set of process and outcome measures helps inform improvement efforts and provides evidence that efforts have made a difference in the lives of patients.
For measurement to be effective, the following principles are important. First, measures should focus on something that the improvement team has the power to change and should initially be simple, small-scale measures that focus on the process itself and not on people. Second, measures should be practical, seek usefulness—not perfection—and fit the work environment and cost constraints. Third, data for measurement should be easy to obtain; finding ways to capture data while the work is getting done allows measures to be built into daily work. Fourth, qualitative data (e.g., reasons for patient dissatisfaction in the patients’ own words, observations to contextualize quantitative data) are highly informative and should complement quantitative data (e.g., percentage of patients satisfied with care). Finally, when using measures, balance is key; a balanced set of measures can help answer the question, “Are we improving parts of our system at the expense of others?”
Measurement should not overwhelm the change process. Improvement teams should minimize the burden of measurement whenever possible. Measurement can have both direct and indirect consequences on resource use, provider behaviors, and patients. Measurement of performance and outcomes of care can be costly, especially if the data collection process is manual and involves chart reviews. The burden of measurement is reduced with an EMR system and computerized order entry, although these information technology systems are costly to implement and maintain. Additionally, these resources may not be equally available throughout a system or organization, leading to disparities in the care provided.
Measurement fixation is an unintended consequence on healthcare staff behavior that may occur with the use of process measures. For example, when a process measure such as “the percentage of diabetic patients who received an action plan” is used rather than an outcome measure such as “improved patient understanding about diabetic management,” the measure is perceived by the clinician as defining what is important. Thus, measurement of the process becomes the priority, rather than the intended outcome. Alternatively, the clinician may become so focused on what is being measured that different aspects of care are not equally prioritized. In addition, a predominance of process rather than outcome measures can stifle innovation by scripting a process, thus inhibiting process-level innovation. Practice variation does have some utility because medical practice is dynamic, and it is through the trial of new methods of care that innovation occurs. Finally, QI performance measures may not match patient preferences for clinical care. Performance measures that do not take patient preference into account can lead to decreased patient satisfaction, trust, and confidence in their healthcare practitioners and system. Thus, selection of a set of appropriate measures with the attributes described previously can be a balancing act that includes weighing the tradeoffs involved.
Consumers, payers, and employers are increasingly requesting outcome measures to both improve care and decrease cost. Even national governmental bodies are influencing the measurement and reporting of quality in health care. In the United States, the Centers for Medicare and Medicaid Services (CMS), the single largest healthcare purchaser, requires hospitals and physicians to participate in the Quality Payment Program (QPP). This program requires providers to demonstrate quality by either participating in an Advanced Alternative Payment Model (akin to a quality collaborative or participants in a bundled payment model) or by accumulating points through the Merit-based Incentive Payment System. The United Kingdom Quality and Outcomes framework is an analogous system. These quality mandates have fundamentally changed the ways that clinicians, hospitals, and health systems engage in and report QI activities.
Analysis and Display of Quality Improvement Data
Interpretation of data and understanding of process variation are fundamental to QI work. Data elements central to improvement are first and foremost—those data are collected as a basis for action. Second, interpretation of data is made within the context of the process. Last, the analysis technique should filter out noise in the process. Aggregate data or summary statistics typically do not filter out noise in the system and do not present a broad enough context to point practitioners in the direction of proper action or process improvement.
Shewhart postulated that data contain both signal and noise; to be able to learn, one must separate the signal from the noise. CQI science defines two types of variation within a process: random variation and specific variation. Random variation, also known as common-cause variation, results from differences in the inputs that a process receives or inherent factors in the process itself. Random variation is the random background noise within a system and occurs in the process all the time. Specific variation, also known as special-cause or attributable variation, is not present all of the time as background noise, but rather arises from one or more specific causes that are not part of the system. A process is considered to be unstable when specific variation exists, and efforts should be made to learn about the special causes for this variation. A stable process exists when specific variation no longer occurs, leaving only random or common variation. CQI aims to eliminate specific variation for every process so that only random variation remains. A standards-based QA system fails to distinguish random cause from special cause and attempts to correct all variation. Attempts to correct random variation will necessarily fail—a process CQI defines as “tampering.” When a process exhibits only random variation, the process should be evaluated to determine whether it is functioning at an acceptable level. If it is not, the process will need to be changed so that the average is moving in the desired direction. Standardization of a process is often the key to reducing random variation and improving a process.
Run Charts and Control Charts
Run charts and control charts are graphic displays of data that enable observation of trends and patterns over time. They are the best tools for determining whether improvement strategies have had an effect. A run chart (see Fig. 5.2 ), also called a time series chart, plots the variable or measure being studied on the vertical axis and plots the time on the horizontal axis. The average, or centerline, is the median. At least 12 data points are required to establish a baseline, and at least 20 to 25 data points are required to detect trends and patterns. Run charts should be annotated with tests of change to provide the context within which data can be interpreted. Four rules can be used with run charts to determine whether nonrandom patterns exist or to detect whether the change has led to an improvement:
- 1.
A shift is indicated by six or more consecutive points above or below the median.
- 2.
A trend is indicated by five or more points all increasing or all decreasing.
- 3.
A run is defined as a series of consecutive points on the same side of the median line.
- 4.
An astronomical data point is an unusual point that is obviously different in value (an outlier).

Because change is by nature temporal, run charts in which data are presented over time are powerful tools for interpreting data within the context of the process.
A control chart (see Fig. 5.3 ), also known as a Shewhart chart, is an extension of a run chart and is used to distinguish between specific and random variation. As on a run chart, the variable is plotted on the vertical axis and time on the horizontal axis. However, with a control chart the centerline or average is the mean, rather than the median, and the upper control limit (UCL) and lower control limit (LCL) are calculated. The UCL and LCL correspond to ±3 sigma from the mean. A process is considered to be “in control,” or stable, when data points are within these control limits. Random variation, or variation that is the result of the regular rhythm of the process, produces a stable process. However, in an unstable process that contains variation from special causes, data points exceed the UCL or LCL.

Failure Modes and Effects Analysis
A failure modes and effects analysis (FMEA) is a tool to help identify problems in a process before they occur and cause harm. An FMEA can help you decide where to target your improvement efforts. In addition, the proactive approach makes it especially useful before implementing a new process.
An FMEA reviews the steps in a process, the potential failure modes (what could go wrong), failure causes (why would the failure happen?), and failure effects (what would be the consequences?). (IHI, QI Essentials Toolkit) After listing the steps in a process, a risk profile number is calculated based on the likelihood of occurrence, likelihood of detection, and severity. Improvement efforts are then targeted at the steps with the highest risk profile number.
A simplified FMEA is a quicker version of the FMEA that can help guide improvement work. A simplified FMEA consists of listing the steps in a process, listing potential failures that could go wrong for each step, and brainstorming interventions for each of those possible failures. Below is an example simplified FMEA for programming infusion pumps that was used in an improvement project to reduce medication errors.
Putting It All Together: An Example Quality Improvement Project
The following is an example of how the QI methodology previously discussed could be used to address a practical problem. A hypothetical example of reducing medication errors in an anesthesiology department is described.
An anesthesia group was concerned over the large number of medication errors that were occurring in their department. They formed a multidisciplinary group including an anesthesiologist, nurse anesthetist, anesthesia resident, and pharmacist. They addressed the first question in the Model for Improvement—“What are we trying to achieve?”—by writing a SMART aim. Their SMART aim was to reduce medication errors in the Department of Anesthesiology from three errors per month to one error per month within 12 months.
The next question in the Model for Improvement is “How will we know that a change will result in an improvement?” In order to monitor their progress, they constructed a run chart of medication errors per month in their department. They captured data through a previously existing self-reporting system.
The last question of the Model for Improvement is “What changes can we make that will result in an improvement?” To better understand the errors that were occurring, the group categorized the medication errors into categories and constructed a Pareto Chart. A Pareto Chart is a bar chart in which categories are listed in descending order. The group learned that three categories of errors accounted for about 80% of the total number of errors. These categories were infusion pump errors, acetaminophen errors, and antibiotic errors. They decided to focus their initial efforts on these three categories. They created process maps for these three categories to better understand the current process and develop possible interventions. Finally, to help organize their theory of improvement, they created a key driver diagram listing the drivers they thought would affect their aim, as well as possible interventions targeting the drivers.
Now that they had a theory of improvement, they started testing their interventions. They tested their ideas using PDSA cycles. They adopted the successful tests, adapted tests with mixed results, and abandoned the failed tests. Some of their tests included requiring a sticker from pharmacy and requiring a two-person double check of infusion pumps. As their testing and implementation continued, they saw the centerline, or median, of the run chart decrease from three to two. The run chart rules indicated this was a significant change. The group was excited that they had decreased the number of medication errors in their department, but they had not yet reached their aim of no more than one medication error per month. They decided to continue testing new ideas until the median decreased to less than one error per month.
Dashboards and Scorecards
A dashboard of measures functions like an instrument panel for an aircraft or automobile and provides real-time feedback on what is happening. Balanced scorecards, or “whole system measures,” are similar to dashboards and are used to provide a complete picture of quality. Developed by Kaplan and Norton, the balanced scorecard is defined as a “multidimensional framework for describing, implementing, and managing strategy at all levels of an enterprise by linking objectives, initiatives, and measures to an organization’s strategy.” A set of measures should reflect the culture and mission of an organization. Viewed collectively, this set of measures provides a gauge of current performance and can also guide the direction for future organizational improvement efforts. A balanced set of measures is critical to ensure that improvement efforts in one area do not adversely affect outcomes in other areas.
Additional Quality Improvement Evaluation and Communication Tools
In most situations, QI frameworks such as the Model for Improvement or Lean Sigma are sufficient to help guide the development, testing, implementation, and spread of improvement. However, to better understand problems within a system or process, QI professionals have developed or adapted a number of methods and tools. Some of these methods and tools help with viewing systems and processes and organizing and communicating information. These are described in the following section.
Understanding how a process or system works is fundamental to improving it. Process mapping is a method to gain this understanding. A flow diagram, or flow chart, is an improvement tool used during process mapping; it provides a visual picture of the process being studied, wherein the series of activities that define a process is graphically represented. Flow charts identify and clarify all steps in the process. They also help the team understand the complexity of the process and identify opportunities for improvement.
Failure mode and effects analysis is a systematic, proactive method of identifying and addressing problems associated with a process. It uses a standardized approach to analysis that includes identifying the various steps in a process and addressing their failure modes, effects, and possible interventions.
A key driver diagram (KDD) ( Fig. 5.4 ) is another approach to organizing the theories and ideas for improvement that a team has developed. The KDD presents the aim or outcome of the project with both the theories (key drivers) behind the improvement and ideas for test of change. Initially, the driver diagram helps lay out the descriptive theory behind the improved outcomes. As these theories are tested, the driver diagram is updated and enhanced to develop a predictive theory. KDDs are extremely useful because they provide a shared mental model for the team during its improvement efforts.
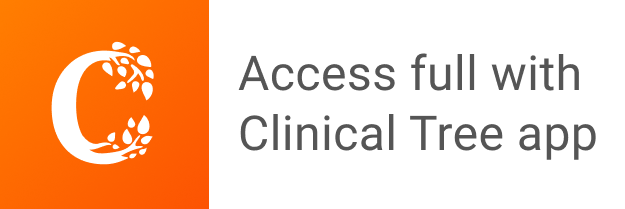