Abstract
Aim
The objective of our research is to evaluate and compare the performance of ChatGPT, Google Bard, and medical students in performing START triage during mass casualty situations.
Method
We conducted a cross-sectional analysis to compare ChatGPT, Google Bard, and medical students in mass casualty incident (MCI) triage using the Simple Triage And Rapid Treatment (START) method. A validated questionnaire with 15 diverse MCI scenarios was used to assess triage accuracy and content analysis in four categories: “Walking wounded,” “Respiration,” “Perfusion,” and “Mental Status.” Statistical analysis compared the results.
Result
Google Bard demonstrated a notably higher accuracy of 60%, while ChatGPT achieved an accuracy of 26.67% ( p = 0.002). Comparatively, medical students performed at an accuracy rate of 64.3% in a previous study. However, there was no significant difference observed between Google Bard and medical students ( p = 0.211). Qualitative content analysis of ‘walking-wounded’, ‘respiration’, ‘perfusion’, and ‘mental status’ indicated that Google Bard outperformed ChatGPT.
Conclusion
Google Bard was found to be superior to ChatGPT in correctly performing mass casualty incident triage. Google Bard achieved an accuracy of 60%, while chatGPT only achieved an accuracy of 26.67%. This difference was statistically significant ( p = 0.002).
1
Introduction
The field of AI natural language processing has witnessed a significant transformation with the advent of advanced language models, leading to remarkable advancements in various tasks. Notably, prominent examples of these models are ChatGPT and Google Bard [ , ].
ChatGPT, an advanced AI language model initially released in November 2022, shows great promise in various medical applications. One of its significant contributions is in the field of diagnosis, where it has consistently outperformed traditional tools like Google search and symptom checkers in online diagnosis [ ].
Additionally, ChatGPT serves as an educational tool for emergency physicians and paramedics. A proof-of-concept study has confirmed its effectiveness in providing engaging and enjoyable teaching experiences for medical professionals [ ]. Moreover, ChatGPT plays a substantial role in public health by supporting disease surveillance, outbreak management, and resource allocation [ , ].
While the Google Bard is an AI chatbot released by Google on March 21, 2023. It mimics human-like conversations using natural language processing and machine learning. The Bard can be used across digital platforms, giving genuine responses and helping in areas like emergency medicine, public health, and disaster management [ ].
In a study comparing ChatGPT, Google Bard, and the paid version of GPT-4 for preparing for neurosurgery oral boards with advanced management cases, the paid GPT-4 scored remarkably well at 82.6%. It did better than both the free ChatGPT3.5 and Google Bard [ ].
Both ChatGPT and Google Bard have limitations and challenges in how they are used. Their quality and reliability can be affected by inconsistent information, infrequent updates, and a lack of validation by experts. Moreover, because natural language is complex and often ambiguous, there can be errors and misunderstandings in how users input their queries [ , ].
A mass casualty incident (MCI) involves a significant number of individuals requiring medical attention [ ]. These incidents can result from various causes such as natural disasters, accidents, or terrorist attacks. While the specifics can differ between countries, MCIs generally involve situations that overwhelm local medical resources [ ]. The defining feature is when there are more patients than available healthcare resources can handle, typically exceeding ten patients [ ].
The START triage method was established in 1983 by the Newport Beach Fire Department and Hoag Hospital in California. It plays a crucial role in quickly and efficiently categorizing MCI victims by injury severity. [ ] START, which stands for Simple Triage and Rapid Treatment, employs four categories to prioritize victims: deceased/expectant (black), immediate (red), delayed (yellow), and walking wounded/minor (green). These categories consider factors like the victim’s ability to walk, respiratory rate, pulse or capillary refill, and mental status, with the sole intervention being opening the airway for non-breathing victims.
The START triage helps first responders in determining treatment priorities and evacuation, ensuring efficient resource allocation [ ]. It involves critical decisions about providing on-site treatment or immediate transportation to the nearest hospital [ ]. Accurate and consistent categorization is vital during an MCI, as errors (over-triage or under-triage) can significantly impact disaster response, potentially leading to loss of lives and resource constraints [ ]. While AI tools in emergency medicine have been explored in previous research, there is limited comparison of these chatbots’ accuracy in START triage.
The objective of our research is to evaluate and compare the performance of ChatGPT, Google Bard, and medical students in performing START triage during mass casualty incidents. We used a validated questionnaire [ ] and compared the results of MCI triage.
2
Methods
Our study is a cross-sectional analysis to assess how well ChatGPT and Google Bard perform in mass casualty incident (MCI) triage using Simple Triage And Rapid Treatment (START) triage. We use mixed methods of quantitative descriptive analysis to evaluate their overall MCI triage performance and content analysis to assess their performance in four headings 1. Walking wounded, 2. Respiration, 3. Perfusion, and 4. Mental state. Lastly, we compare their accuracy to that of medical students using the same triage questionnaire performed by Sapp et al. [ ].
2.1
Materials
For this research, we used ChatGPT-3.5, developed by OpenAI in San Francisco, CA, and freely available for public use [ ]. We also used the Google Bard model, which ran on PaLM 2 and was updated on June 7, 2023. The data was collected in Malaysia and analyzed on July 5, 2023 [ ]. No ethical considerations are needed since all data used are from open source and secondary data.
In the study, with written permission, we employed a validated mass casualty incident triage questionnaire from Sapp et al. [ ]. The questionnaire’s 15 scenarios were expertly crafted by Emergency Medical Services (EMS) Medical Directors and Emergency Faculty affiliated with the University of North Carolina School of Medicine. They have extensive training and experience in crisis management and emergency aid. The scenarios were carefully selected to ensure diverse triage levels and START criteria adherence.
Each scenario provided detailed patient information, including age, symptoms, vital signs (such as breathing rate, heart rate, and capillary refill), and the method of transportation to the hospital. The triage questionnaire considered various medical and traumatic conditions, excluding sarin gas exposure [ ]. The triage questionnaire included four cases classified as “Red” (Immediate), four as “Yellow” (Delayed), four as “Green” (Minor), and three as “Black” (Deceased) status. The complete triage questionnaire is available in Appendix 1.
The mean accuracy scores of medical students were obtained from a previously published study conducted with two consecutive classes of first-year students in 2008 and 2009, a total of 315 students, at the University of North Carolina School of Medicine in Chapel Hill. These students had participated in START triage training and had completed a paper-based triage exercise during their orientation. The study’s findings were reported by Sapp et al. [ ].
2.2
Data collection
Our study tested ChatGPT and Google Bard’s ability to perform START triage using the prompts ‘Do you know START triage?’ and ‘Can you perform START triage?’. After confirming their ability, we individually presented questions from the mass casualty triage questionnaire (see Appendix 1). We recorded all responses from both AI chatbots in an Excel spreadsheet for detailed analysis.
2.3
Data analysis
After the mass casualty incident START triage, we analyzed ChatGPT and Google Bard’s performance on the mass casualty triage questionnaire. We categorized their performance into three types: 1) Correct-triage, 2) Over-triage, and 3) Under-triage [ ]. We checked ChatGPT and Google Bard’s triage performance by collecting data and calculating their proportions in all three triage areas.
For content analysis, we thoroughly analyzed the responses of ChatGPT and Google Bard. We focused on four themes: walking wounded, respiration, perfusion, and mental status. Using START adult triage guidelines as a reference, we categorized their performance as either correct or incorrect to assess their accuracy in these areas [ ]. According to the questionnaire, correct responses were those that appropriately reflected the patient’s circumstance and triage decision using the START triage algorithm. Responses that didn’t match were considered wrong. We recorded all questions and replies from ChatGPT and Google Bard performances in a Microsoft Excel spreadsheet.
We analyzed the data using percentages and two non-parametric statistical tests. First, we used the Kruskal-Wallis test to compare the average accuracies of three groups (ChatGPT, Bard, and medical students) due to our small sample size and non-normally distributed data. Second, we used the Mann-Whitney U test to compare any two groups, and we performed these tests using IBM SPSS Statistics 23.
3
Results
We categorized the results into three groups: Correct-triage, Over-triage, and Under-triage, based on response accuracy. ChatGPT’s performance showed a notable over-triage rate in 10 out of 15 cases (66.67%), assigning higher care than necessary. Only 4 out of 15 cases (26.67%) were accurately identified for the appropriate level of care. There was a very small number of under-triage cases, 1 out of 15 (6.67%), indicating missed opportunities for higher care when needed.
Google Bard correctly identified and assigned 9 out of 15 patients to the right level of care, making accurate decisions for 60% of them. However, for 6 patients (40%), it assigned a higher level of care than necessary, possibly leading to excess attention and resources. On the positive side, none of the patients were under-triaged, meaning all who needed higher care were correctly identified and treated accordingly. Medical students performance from Sapp et al. shows overall accuracy of (64.3%), overall under-triage (12.6%), and overall over-triage(17.82%) [ ]. Overall performance is shown in Fig. 1 .
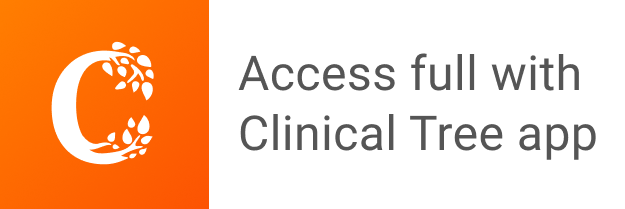