Learning From the Data—Discovery Informatics for Critically Ill Children
Randall C. Wetzel
The collection, analysis, interpretation, and application of data from myriad medical transactions occurring during healthcare processes will improve healthcare and patients’ outcomes in the next decade more than the biomedical sciences have in the last century (1,2). At the moment, this hope is perhaps more hype than reality; however, the promise is being realized daily in pediatric critical care medicine. Since the beginning of medicine we have been exhorted to pay attention to the patient’s data, to examine the evidence of disease, and to learn from our practice. This has not changed—what is changing is the information technology revolution that will radically assist our ability to do this and thus improve the care we provide our patients through what the National Science Foundation calls Discovery Informatics (3).
MEANINGFUL USE OF MEDICAL DATA—CAPTURE, INGEST, CURATE, ANALYZE, AND LEARN
Building on a growing awareness of the importance of information technology in healthcare signaled by the
2009 Health Information Technology for Economic and Clinical Health (HITECH) Act, Medicare and Medicaid Electronic Health Records (EHR) Incentive Programs now provide financial incentives for the “meaningful use” of certified EHR technology to improve patient care. To receive an EHR incentive payment, providers must show that they are “meaningfully using” their EHRs by meeting thresholds for a number of objectives. Centers for Medicare & Medicaid Services (CMS) has established the objectives for “meaningful use” that eligible professionals, eligible hospitals, and critical access hospitals must meet to receive an incentive payment. The enthusiasm of the federal government for the installation, adoption, and use of information technology for healthcare is evident by CMS‘s commitment of 27 billion dollars for the meaningful use initiative. Nevertheless, there is a problem: “right now computational and biomedical research travel largely on uncoordinated parallel tracks” (4). Since 2000 the National Institute of Health (NIH) and National Library of Medicine (NLM) have launched scores of initiatives to bring the fruits of computation to healthcare such as the Biomedical Information Science and Technology Initiative (BISTI), which bridges all NIH institutes (www.bisti.nih.gov). “People might make an algorithmic advance that will eventually have some impact in biomedical research but it’s not a coordinated effort. … The two fields speak different languages, so it’s really tough to translate state of the art developments in computer science, artificial intelligence (AI) and math into things that will be useful in biomedical research” (4). Although this is a necessary first step, the meaningful use of complex healthcare data will require more than just installing EHRs.

Healthcare generates massive amounts of data. We have continuously captured clinical data, laboratory results, mountains of clinician notes (doctors, nurses, social workers, etc.), business information, diagnostic coding, therapeutic data, and even outcomes data. In addition, to this great volume of data, there is obviously great variety in the data collected and increasingly stored, and the data come at us with great velocity—overwhelming our human data management systems. We all suspect that these data have great value and they also have the quality of veracity—that is,
they tell us what has occurred around patient care process, often in great detail. These five v’s, volume, velocity, variety, value, and veracity, attributes of medical data, are the same as those frequently used to describe so-called ‘Big Data.’ Big Data can be loosely described as massive amounts of data that pose new and overwhelming challenges for “traditional” data management and computational approaches. The challenge of Big Data, in turn, has led to innovative developments in acquiring, curating, storing, and analyzing very large amounts of continuously streaming data – such as we increasingly see in healthcare (5,6).

Big Data is all around us. Each engine of the jet flying from Los Angeles to New York generates 10 TB of data every 30 minutes. In 2013 Internet data, mostly user-contributed, will account for 1000 exabytes (an exabyte is a unit of information equal to one quintillion [1012] bytes). Open weather data collected by the National Oceanic and Atmospheric Association have an annual estimated value of $10 billion. Every day we create 2.5 quintillion bytes of data. Ninety percent of the data in the world today have been created in the past 2 years. Google receives 2 million search requests every minute, and responds to these requests with innovative machine learning (ML) search technologies. Every industry has benefited from the capture and analysis of Big Data often in real time. The recent America’s cup victory by the Oracle Team USA was powered by real-time Big Data analytics analyzing gigabytes of data per hour during and after each race. Formula 1 motor racing has become a Big Data-driven exercise, informing drivers in real time, organizing pit stops, and redesigning and rebuilding the race cars (Peter van Mannan’s Ted talk: https://www.ted.com/talks/peter_van_manen_how_can_formula_1_ racing_help_babies, to understand this and how it is being applied in neonatal critical care.)
Like these sports, our specialty is time constrained, valuable, high-risk, mission critical, requires teamwork, and is very complex. Yet the care we deliver in our ICUs continues to be driven by what the human eye sees, the ear hears, and the brain analyzes. Although our brains are superior analytic and pattern recognition biologic entities, the data upon which they act are often limited and often dependent on human attention and recall, and influenced by memory, bias, prejudice, exhaustion, distraction, and other systematic sources of human error. All of this is based on our practice experience, and we revere the ability to recall clinical experience and combine these actual experiences into clinical anecdotes upon which we base a significant amount of our practice. Unfortunately, recall is our problem; practice is long, but memory short.
“Discovery Informatics focuses on computing advances aimed at identifying scientific discovery processes that require knowledge assimilation and reasoning and applying principles of intelligent computing and information systems in order to understand, automate, improve and innovate any aspect of those processes.”
Yolanda Gil, NSF workshop on
Discovery Informatics (3)
Fortunately, we are living in the revolutionary era of Big Data. Many segments of our economy have been radically transformed by collecting, analyzing, and learning from large amounts of data captured during their normal production processes. Whether this is used to improve manufacturing, to enhance the value of social media, to provide marketing advantages or for national security reasons, Big Data and its analysis are currently all the rage and are here to stay. In research, a recent Nature editorial commented: “Researchers need to adapt their institutions and practices in response to torrents of new data—and need to complement smart science with smart searching” (7). This adaptation is urgent in healthcare. Perhaps the last great domain waiting to benefit from the acquisition and analysis of Big Data is healthcare—although this is rapidly changing. Recently, the Human Genome Project in the 1990s has done for healthcare Big Data research what President Kennedy’s commitment to go to the moon in 1963 did for computer science.
At its heart, analysis of Big Data is about discovering new knowledge and therefore about learning. It can inform us about quality improvement, process improvement, process efficiency, safety, and product reliability, and can reveal insights about how best to manage our patients—surely the most important topics in healthcare today. We need to both improve and accelerate the data, to predictive modeling, to therapeutic action cycle to achieve the dual goals of improving care while bringing down costs. This approach will improve not just our clinical care but also many aspects of the practice of intensive care medicine (Fig. 12.1).
Surely we can bring to bear an information technology “cognitive prosthesis” to enhance our skills and ensure that what actually happens to our patients is accurately recalled and analyzed. These captured data, detailing medical interactions and their outcomes, can be used to enhance and expand our experience. Furthermore, the amount of information in our ICUs has burgeoned. When care was informed by looking at paper flow sheets of a few vital signs recorded every 15 minutes, human ability to analyze these sparse data was not strained. Now critical care requires the interpretation of multiple waveforms, combined with blood gases, laboratory results, MRIs, and the data streaming from our observational armamentarium of what is occurring within our patients. It is daunting. Additionally, that may be only 1 of 20 patients who are critically ill in an ICU. Engineers would consider the data management of a single ICU a streaming terabyte problem. Yet our practice has changed little, while the world around us has been transformed by Big Data analytics. Add to this the often quoted realization pointed out by Miller in 1956: “The span of absolute judgment and the span of immediate memory impose severe limitations on the amount of information that humans are able to receive, process, and remember.” This has been referred to as the Magic Number 7 (8). The observation that we are limited to managing only seven continuous data streams at a time suggests that managing hundreds of simultaneous data streams from scores of patients is not merely a daunting (if not impossible) task but one that might be expected to be rife with error, missed information, poor safety, poor quality, little time to reflect on patient care issues, frustration, and bad outcomes.
In this setting of streams of data telling us how our patients are responding, intensivists must not only consume and process
these data but also take into account what we are doing to our patients. There are myriad transactional occurrences for every child in the ICU every hour from drips, to ventilators, to drug infusions and injections, sedation, paralysis, antibiotics dialysis, and the results of all of the interventions. These transactions occur during the continuous collection of physiologic data from critically ill children. The intensivist is expected to not only observe but also analyze and act on this information for the benefit of our patients. In this sea of data, what is a clinician to do? How can he or she avoid drowning in the continuous barrage of voluminous, variable, high-velocity data and instead learn from it to guide practice-the care of our next patient? This is essentially the informatics challenge to which many industries have risen, and that healthcare is just discovering. Finally, we must also learn from these data. This is called practice-based learning or evidence-based healthcare (2). Appropriate data management will allow us to continuously learn from routinely collected healthcare data (9).

Thankfully, we live in a connected, computationally sophisticated world (Fig. 12.2). There is little new about the call to observe and learn from our patients; after all, Hippocrates exhorted us to carefully collect and record the evidence about patients and their illnesses, and to learn from that data to help our future patients. In fact, healthcare is based on the careful observation of patients and how they respond to our interventions —these all form natural experiments from which we can learn. In fact, failure to learn from our practice and experience is an unethical failure of our responsibility to our next patient. Yet in our ICUs, we have done literally millions of experiments, captured and perhaps even observed the data—but then
thrown it away, not subjected it to analysis, and failed to learn from it. Until recently, with the advent of the EHR, accessing large amounts of detailed clinical data has been extremely difficult. But we live in a different world. What is new is the informatics era in which we live that enables us to do this in an increasingly effective fashion.
thrown it away, not subjected it to analysis, and failed to learn from it. Until recently, with the advent of the EHR, accessing large amounts of detailed clinical data has been extremely difficult. But we live in a different world. What is new is the informatics era in which we live that enables us to do this in an increasingly effective fashion.
So how do we learn from the data generated in terabyte quantities as we practice every day? There are many ways to learn: experientially, didactically, interactively, and, yes, in healthcare, anecdotally—by our practice and experience— often dependent on our recall. Yet we have come to mistrust the anecdote, and for good reasons based to some extent on
the failure of human recall. Instead, we have relied on experimental testing and the powerful research tool of falsification of hypothesis—so-called evidence-based medicine. The “evidence” is that derived from carefully controlled clinical trials or well-designed observational studies. These are increasingly hard to perform, expensive, and often unable to be performed owing to the lack of clinical equipoise; and although valid, results may lack external validity and only poorly inform what we can do for our next patient (is my patient like these patients?), and require internal validity and exacting experimental test statistics. This tool has served medicine well. Nevertheless, not surprisingly, intensivists frequently lament that only a minority of our practice is evidence based and that the majority of our practice is unexamined, resulting in idiosyncratic, diverse, and often contradictory management of equivalent patients by multiple intensivists across many ICUs. Clearly, there are large parts of intensive care that require further knowledge discovery to determine optimal therapies and best practices and to improve outcomes.
the failure of human recall. Instead, we have relied on experimental testing and the powerful research tool of falsification of hypothesis—so-called evidence-based medicine. The “evidence” is that derived from carefully controlled clinical trials or well-designed observational studies. These are increasingly hard to perform, expensive, and often unable to be performed owing to the lack of clinical equipoise; and although valid, results may lack external validity and only poorly inform what we can do for our next patient (is my patient like these patients?), and require internal validity and exacting experimental test statistics. This tool has served medicine well. Nevertheless, not surprisingly, intensivists frequently lament that only a minority of our practice is evidence based and that the majority of our practice is unexamined, resulting in idiosyncratic, diverse, and often contradictory management of equivalent patients by multiple intensivists across many ICUs. Clearly, there are large parts of intensive care that require further knowledge discovery to determine optimal therapies and best practices and to improve outcomes.
Research is the discovery of new knowledge, the recognition of new relationships, and the understanding of fundamental processes. For thousands of years of human existence, new knowledge was discovered empirically—by observation
and deduction, relying on the accurate collection of data from which conclusions could be drawn. If the observations were “true,” then the deductions were true. These provided experimental proofs. Yet our human ability to maintain and manage sufficient data, and recall it accurately without bias is limited, and thus this limited the power of deduction to move us forward as rapidly as the new inductive method of science. Falsification of hypothesis was a way to know what was not true, and if we failed experimentally to falsify the null hypothesis, the hypothesis was upheld—at least until it too fell to the sword of disproof. This is a very powerful experimental technique at the heart of the scientific method that has revolutionized healthcare. Demonstrate once with good experimental design that a therapy is of no value, and it falls out of use. Failure to support the null hypothesis—there is no difference between a drug and a placebo—and the drug is adopted. But this too is now failing. And we live in a different world.

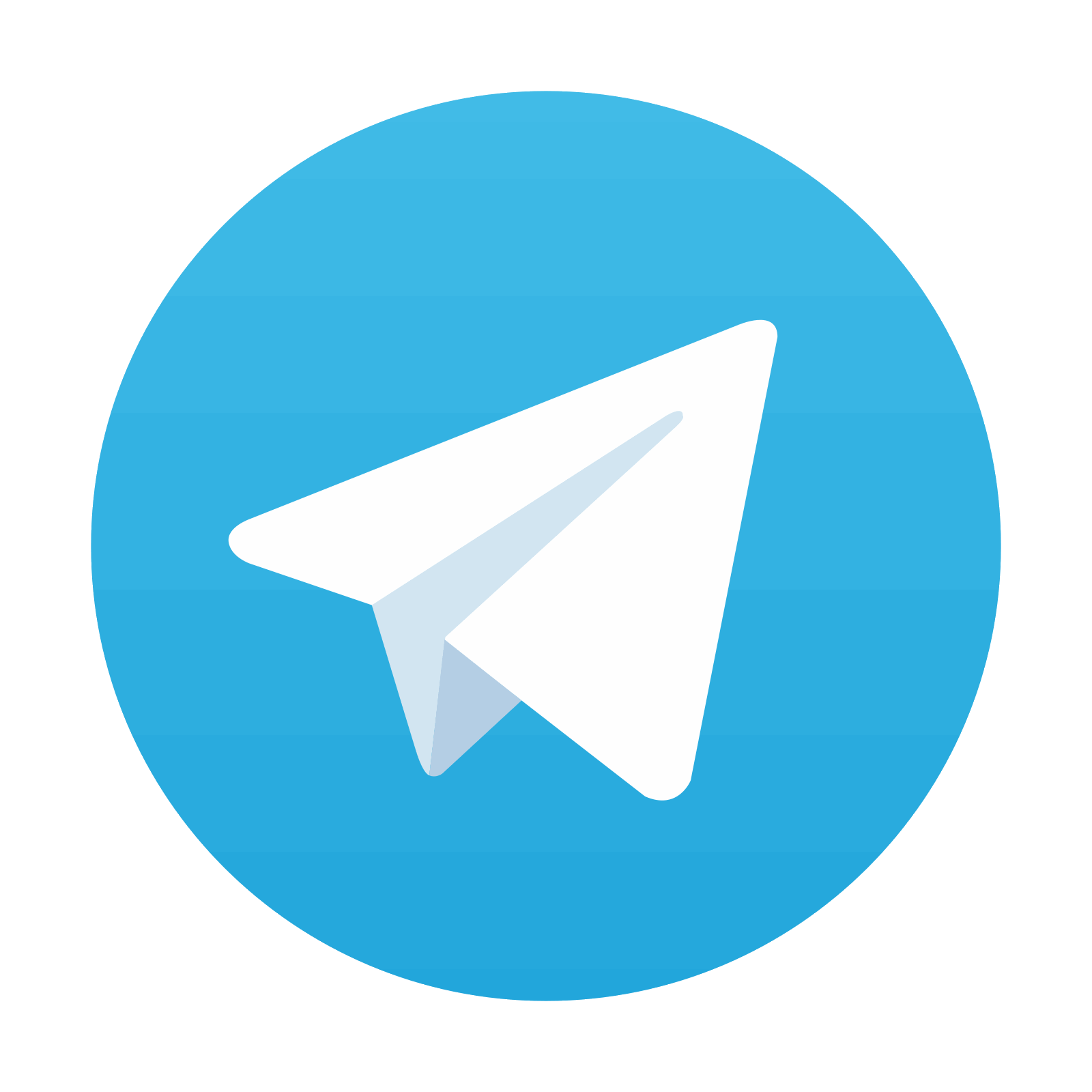
Stay updated, free articles. Join our Telegram channel

Full access? Get Clinical Tree
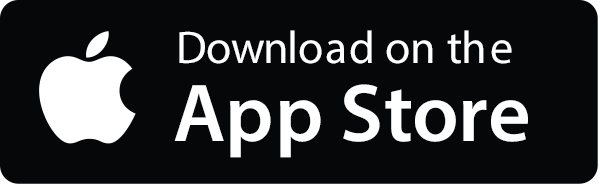
