Key Points
- ▪
Clinical research describes the characteristics and mechanisms of disease and injury in humans and investigates drugs, devices, diagnostic products, and bundles of care with the aim of providing high-quality evidence to guide clinical practice that improves patients’ lives.
- ▪
Investigators are constrained by the need to draw conclusions based on a sample of patients, providers, or systems rather than the whole of the population of interest, and this introduces random, systematic, and design error that threatens internal and external validity.
- ▪
Observational (or nonexperimental) studies involve allowing nature or clinical care to take its course, without any major modification due to study-related procedures. However, nonexperimental designs are prone to systematic error, such as selection and information bias.
- ▪
In experimental studies the investigators do not let nature (or clinical care) take its course, but actively intervene to test a new intervention. Randomization and blinding reduce the risk of random and systematic errors in experimental studies, but generalizability can be limited.
- ▪
Systematic reviews synthesize the medical literature using a transparent search strategy that every reader can replicate and update, and accompanying metaanalyses produce aggregate estimates of treatment effects.
- ▪
A carefully designed protocol is the foundation of all clinical research, facilitating the review, conduct, and eventual publication of the project. Prior planning of sample size and statistical analyses is essential.
- ▪
Appropriate ethics, registration, and regulatory approvals; financial, data, and human resource management; plans for monitoring patient safety and data integrity; and plans for publishing the results and sharing the data are vital to the proper conduct of clinical research.
- ▪
A quality improvement cycle of reflection, feedback, and forward planning is beneficial. Giving patients an opportunity to comment on the studies in which they participated gives researchers fresh insight and opens up new avenues of research.
The measure of greatness in a scientific idea is the extent to which it stimulates thought and opens up new lines of research. Paul A.M. Dirac
Introduction
Research is systematic investigation for the creation of generalizable knowledge. Clinical research describes the characteristics and mechanisms of disease and injury in humans, and investigates drugs, devices, diagnostic products, and bundles of care for human use. Clinical research also includes investigating the interactions of health professionals, students, and other stakeholders with the health care and health education systems. Most studies address an aspect of quality of care or education such as safety, effectiveness, patient-centeredness, timeliness, efficiency, and equity.
Miller’s Anesthesia includes references to tens of thousands of clinical research studies. The main purpose of this chapter is to describe how these studies were designed and conducted. The chapter connects directly with Chapter 91 , which elaborates on how to interpret and use evidence for clinical decisionmaking. Other related chapters include Chapter 1, Chapter 2, Chapter 4, Chapter 5, Chapter 8 , and Chapter 30 . Our goal is to describe how clinical research originates and to inspire our readers to create and support the use of high-quality evidence that is essential in guiding clinical practice that focuses on improving patients’ lives.
Key Principles
Researchers want to produce results that will be useful to clinicians in improving patients’ lives. However, they are constrained by the need to draw conclusions based on a sample of patients rather than the population of interest as a whole. The random, systematic, and design errors introduced by studying a sample affect the confidence with which the association found in the study can be claimed to truly represent the causal effects of the exposure on the outcome (“internal validity”) and impair the generalizability of the results to the source population for the sample and the population of interest as a whole (“external validity”). These errors will be discussed briefly here and will be highlighted in subsequent sections of this chapter.
Random Error
Random error (or “chance”) in the association between an exposure and an outcome is introduced by variation between the individuals in the study and within individuals over time, and variation between and within measurements made during the study. Random error is equally likely to cause false acceptance and false rejection of the null hypothesis (i.e., the hypothesis that there is no effect). The risk of drawing false conclusions increases with decreasing sample size and more statistical testing.
Systematic Error
Systematic errors (or “biases”) also threaten identification of the true causal effect that the exposure had on the outcome in the sample. They can arise from nature (confounding), selection features of the study (selection bias), or measurement features of the study (information bias). In contrast to random error, which distorts the results of a study in both directions, systematic error distorts only in one direction (i.e., toward acceptance or rejection of hypotheses).
Confounding
Confounding is a bias that arises when an association between an exposure and an outcome fails to take account of a third factor (a “confounder”) that is associated with both the exposure and the outcome. Confounders can be risk factors, preventive factors, or surrogate markers for another cause of the outcome, but they cannot be intermediary steps in the causal pathway between an exposure and an outcome. They may be known and measured (“known knowns”), known and unmeasured (“known unknowns”), or unknown and unmeasured (“unknown unknowns”). In observational studies, design (e.g., sampling restriction, matching) and analysis (e.g., statistical adjustment) options only address the distribution of measured confounders. Randomization distributes measured and unmeasured confounders evenly between groups (with increasing reliability in larger studies). Concealment of allocation prevents selective recruitment, and blinding prevents confounding that results when knowledge of group allocation changes processes of care or behavior.
The importance of confounding varies with the type of study. In studies focusing on causality (e.g., whether smoking causes lung cancer), investigators attempt to collect and manage all possible confounders, yet they must always take residual confounding into account or use randomization and blinding to ensure that known and unknown confounders are balanced between the groups. In studies focusing on prognosis (e.g., studies predicting the likelihood that a patient will survive the proposed surgical procedure) any variable that contributes to improved prediction can be used in the prediction model.
Selection Bias
Bias can be introduced by the methods used to select a population of interest, to identify and sample a source of such patients, to recruit and retain those patients, and to disseminate the results. Observational studies are prone to bias with respect to selection of sources of exposed and unexposed patients, or cases and controls. Patients and their treating team can introduce bias by participating or not participating based on the exposures and outcomes under study. Prospective cohort studies and randomized trials are prone to bias with respect to differential loss to follow-up. Studies without preplanned and publicized protocols and statistical analysis plans are prone to bias with respect to selection of outcomes to report (favoring outcomes with statistically significant differences between groups), and studies that fail to find a difference between groups with respect to the primary outcome (“negative” studies) may result in “publication bias,” that is, they are either never submitted or not selected for publication by journal editors.
Information Bias
This type of bias can be introduced by inaccurate measurement or classification of exposures, outcomes, and other measured variables. This error arises when survey instruments and diagnostic tests are invalid or unreliable and may be differential (affecting groups differently) or nondifferential (affecting groups similarly). Recall bias arises when recollection of past exposures is affected by whether the patient experienced the outcome or not. Socially desirable response bias arises when participants give answers based on their assumptions about the investigators and society. Patients who are not assessed for the primary outcome (i.e., whose information is “missing”) can cause random or systematic error, and this should be assessed with sensitivity analyses.
Design Error
Design errors limit the usefulness of research to clinicians, even if the research is free of random and systematic errors, by affecting its generalizability. Examples of such design errors include studying exposures that are expensive or difficult to implement; comparing new treatments with placebos or weak treatments rather than the best available option; assessing outcomes that are not relevant to patients and the community; and seeking to prove that a new treatment is superior to an old treatment, when proving that it is equivalent or non-inferior would be more useful.
Statistical Inference
The P -value is the “probability under a specified statistical model that a statistical summary of the data (e.g., the sample mean difference between two compared groups) would be equal to or more extreme than its observed value.” For example, P = .05 means that under the null hypothesis there was a 5% chance of observing results at least as extreme as those seen in the study. P -values can indicate how incompatible the data are with a specified statistical model, but do not measure the probability that the studied hypothesis is true nor that the data were produced by random error alone. Confidence intervals are better suited to reflecting the size and precision of the treatment effect than P -values: the 95% confidence interval refers to the fact that if the same study were repeated many times and the confidence intervals were similarly calculated for each case, 95% of such intervals would include the true treatment effect. The minimal clinically important difference is important when assessing confidence intervals. If the lower limit of the confidence interval excludes the minimal clinically important difference, then the effect of the treatment is likely to be important. Bayesian inference overcomes some of the limitations of P -values and confidence intervals. Instead of interpreting the frequency of a phenomenon, Bayesian interference incorporates prior evidence, biological plausibility, and pre-existing beliefs into the calculation of the probability of a treatment effect.
Study Design
The study designs used in clinical research are outlined in Fig. 89.1 and Box 89.1 . In this chapter, we use the terms “retrospective” and “prospective” to describe the timing of measurement of the exposure and outcome relative to the start of the study, rather than to describe the direction of enquiry (i.e., outcome → exposure or exposure → outcome). We use the US National Institutes of Health definition of a clinical trial (“any research study that prospectively assigns human participants or groups of human participants to one or more health-related interventions to evaluate the effects on health outcomes”).

A case study reported on a patient who received light anesthesia and was aware.
An ecological study reported on the incidence of awareness in hospitals with low or high per-patient volatile anesthetic use.
A survey asked anesthesiologists about their routine use of light or deep anesthesia and the incidence of awareness in their practice.
A case-control study found patients with awareness, matched them with patients without awareness, and then determined if they had received light or deep anesthesia.
A case-cohort study found patients who had light anesthesia, matched them with patients who had deep anesthesia, then determined if they had been aware.
A retrospective cohort study examined existing records to determine the incidence of awareness in patients who had received light or deep anesthesia.
A prospective cohort study enrolled patients having general anesthesia and followed them to determine the incidence of awareness in patients receiving light and deep anesthesia.
A within-patient comparison of two processed EEG monitors determined each monitor’s ability to predict awareness.
An audit compared current practice with a national evidence-based guideline on prevention of awareness during general anesthesia.
A prediction study reported on the development of a risk score for awareness based on a large cohort of patients having light and deep anesthesia.
A before-and-after study enrolled a cohort of patients having anesthesia without EEG monitoring and then a cohort having anesthesia with EEG monitoring and compared the incidences of awareness.
A randomized controlled trial randomized patients to light anesthesia or deep anesthesia and compared the incidence of awareness in each group.
A systematic review searched for clinical trials examining the relationship between light and deep anesthesia and awareness, and conducted metaanalysis to determine the pooled risk of awareness in patients receiving light or deep anesthesia.
EEG, Electroencephalographic.
Observational Studies
Observational (or nonexperimental) studies involve allowing nature or clinical care to take its course, with assignment to the intervention based on usual practice, and without any major modification due to study-related procedures, such as recruitment or data collection. An observational study is not a clinical trial, therefore the contradictory term “observational trial” should be avoided. Ethical and pragmatic considerations often mean that an observational design is the only approach to answer a research question. For example, most research evaluating the impact of cigarette smoking on lung cancer relies on nonexperimental methods, since assigning smoking as an intervention is unethical. In general, observational studies can demonstrate associations between exposures and outcomes, but do not necessarily prove a causal relationship. Several factors must be carefully considered, including the consistency of findings across multiple high-quality studies, biological plausibility, and whether clear dose-response and temporal relationships exist between exposures and outcomes. This is the case for cigarette smoking: the weight of observational research eventually established cigarette smoking as a cause of lung cancer.
Since most biomedical research is observational in design, several initiatives have been undertaken to improve the conduct and reporting of such studies. For example, the Strengthening the Reporting of Observational Studies in Epidemiology (STROBE) statement makes recommendations for the reporting of three specific types of observational studies, namely cross-sectional, case-control, and cohort studies. However, strong evidence that these tools improve the design and reporting of observational studies is lacking.
Descriptive Studies
Descriptive studies aim to describe the characteristics of patients in a sample, such as baseline features (e.g., age, sex, comorbid disease), processes of care (e.g., anesthesia type), and outcomes (e.g., mortality, stroke). Unlike analytic studies, they do not seek to define relationships among these characteristics.
Case Reports and Series
Case reports describe exposures and outcomes in individual patients, while case series provide the same information for groups of patients. Case reports and series are suited to describing newly recognized uncommon exposures and outcomes, such as drug-related adverse effects. As these studies focus on individuals with a specific exposure or outcome, no direct comparisons are possible with unexposed individuals or those without the outcome. Despite these inherent limitations, case reports and series have made important contributions to medicine. For example, a case report first identified lipid emulsion as a successful treatment for local anesthetic cardiotoxicity and a case series of anesthetic-related deaths in a family first identified the pharmacogenetic disorder malignant hyperthermia.
Descriptions of New Interventions
These studies are a subtype of case reports and series that describe new interventions (e.g., drugs, devices, diagnostic products, monitors, bundles of care, survey instruments, guidelines), but without any comparison against a control group. Such studies have provided valuable information to advance medicine. Important examples include the first use of the non-depolarizing muscle relaxant curare and the initial clinical experience with the laryngeal mask airway. With respect to diagnostic tests, “test research” determines the properties of the test itself (i.e., sensitivity, specificity, likelihood ratios, positive and negative predictive values). However, predictive values in particular are dependent on the prevalence of the outcome in the study population. A better approach (“diagnostic research”) determines the extent to which adding a new diagnostic test improves the likelihood of arriving at the correct diagnosis compared with existing diagnostic criteria. Diagnostic tests should always be evaluated using both methods, especially if the new test is simpler, cheaper, less invasive, or less burdensome to the patient than the existing test.
Analytic Studies
Analytic studies seek to measure associations between exposures and outcomes. In contrast to descriptive studies, where all patients have the specified exposure (e.g., a laryngeal mask airway ) and/or outcome (e.g., successful resuscitation from local anesthetic cardiotoxicity ), in analytic studies patients with and without the exposure of interest (e.g., early childhood exposure to anesthesia) and outcome of interest (e.g., poor educational performance) are required in order to assess associations beyond those expected by chance alone.
Ecological Studies
Ecological studies or “aggregate risk studies” measure exposure status and outcome status as average values across groups of individuals. They are particularly suited to exposures and outcomes that are routinely measured in populations. A major limitation of this approach is that exposure status and outcome status may not be linked at the individual level, leading to the “ecological fallacy,” where individuals within the group-level unit with the outcome of interest may not have been the individuals with the exposure of interest. The potential for this bias was highlighted in a study of hospital-level use of neuraxial anesthesia for hip fracture surgery. Higher hospital-level rates of neuraxial anesthesia use were associated with lower overall mortality, but there was no evidence of such an association at the individual level. In fact, at hospitals with higher rates of neuraxial anesthesia use, all patients had better outcomes after hip fracture surgery, regardless of the type of anesthesia they received, suggesting that the causal mechanisms for hospital-level effects were factors other than neuraxial anesthesia use.
Cross-Sectional Studies
Cross-sectional studies assess the exposure status and outcome status of individuals at the same time (or within a short and stable interval). They are suited to exposures that do not change over time, such as genetically-determined characteristics or chronic stable health conditions. Cross-sectional methods have been used to determine whether tibial nerve ultrasound can detect diabetic peripheral neuropathy and whether a preoperative screening questionnaire detects obstructive sleep apnea. Most surveys (see later) are cross-sectional studies since respondents typically complete the survey at a single time-point. Cross-sectional studies are ill-suited to establishing causal relationships because of the “causality dilemma” (i.e., they do not permit clear delineation of the temporal link between putative exposures and outcomes). For example, children presenting for dental treatment and their accompanying parent were tested for anxiety. Forty percent of the children and 60% of the parents were anxious preoperatively, but the investigators could not determine with any certainly if these states were related and, if so, the direction of effect.
Case-Control Studies
Case-control studies assemble participants based on their outcome status; that is, patients who experienced the outcome (cases) and patients who did not (controls). Once the sample is assembled, exposure status is ascertained by looking back in time ( Fig. 89.2A ). Case-control studies are inexpensive, amenable to quick completion, and suited to the study of rare outcomes, such as postoperative stroke and ischemic optic neuropathy. However, they are susceptible to selection bias, especially in relation to the controls, who should be drawn from the same underlying population as the cases. Thus, in a hypothetical study of stroke following cardiac surgery, it would not be appropriate to select cases who had complex aortic arch procedures and controls who had straightforward coronary artery bypass grafting procedures. Investigators can further avoid selection bias by matching cases with up to five controls based on prognostically important characteristics (e.g., age, sex), using more than one control group, and measuring exposure status with equal rigor across cases and controls (avoiding information bias).

Cohort Studies
Cohort studies assemble participants based on their exposure status and follow them forward in time to ascertain the presence of outcomes. Both the exposure and outcome need to be relatively common to limit the size of the cohort required. Cohort studies can be conducted prospectively and retrospectively. In a prospective study, the cohort is assembled in the present and followed into the future (see Fig. 89.2B ). This allows investigators to carefully measure exposure status (without recall bias based on knowing the outcome); delineate temporal relationships between exposures and outcomes; and implement standardized follow-up. Standardized follow-up is particularly important when the outcomes might be missed or when baseline characteristics might influence surveillance for the outcomes. For example, standardized troponin estimation is required to detect myocardial infarction and injury, because these outcomes may be silent and some patients are less likely to be tested than others (i.e., females, low-risk surgery patients). Prospective cohort studies are more expensive and take longer to complete than case-control studies and retrospective cohort studies.
In retrospective cohort studies, cohort assembly and follow-up occur entirely in the past (see Fig. 89.2C ). Very large databases make addressing uncommon exposures and outcomes feasible using this design. The data needed for the research (i.e., patient characteristics, processes of care and outcomes) can be captured from one or more pre-existing sources, including paper and electronic medical records, administrative and legal databases, government and clinical registries, and databases assembled for research purposes. In anesthesiology, increasing access to these sources has facilitated growth in such research. Retrospective cohort studies are quicker and less expensive to conduct than prospective cohort studies. However, they are limited by the available data sources, which may vary in completeness and accuracy, and be influenced by the intensity of follow-up protocols. For example, the reported incidence of postoperative myocardial infarction varied considerably between prospective studies that used standardized surveillance and retrospective studies that used registries of usual clinical practice.
Case-Cohort Studies
Case-cohort studies are a subtype of cohort studies where exposed patients are matched to unexposed patients, then followed forward in time to measure outcomes. They can be conducted either prospectively or retrospectively and are suited to the study of rare exposures. Matching reduces the influence of differences in prognostically important characteristics (e.g., age, comorbid status) between exposed and unexposed individuals. For example, a retrospective cohort study evaluating the impact of early childhood anesthesia on educational outcomes matched exposed and unexposed children based on gestational age at birth, maternal age at birth, year of birth, sex, and location of residence. Matching on large numbers of baseline characteristics generally is not feasible because of the difficulties in identifying suitable individuals. In these cases, propensity score matching is an alternative that can help assemble a matched cohort with very similar baseline characteristics for both exposed and unexposed individuals. Importantly, however, propensity score matching does not remove bias related to imbalances in unmeasured confounders between the groups.
Studies Assessing Changes or Differences in Individuals
Some studies make serial measurements in individuals to assess changes over time. Examples include studies that characterize the pharmacokinetics and dynamics of anesthetic drugs or postoperative acute pain trajectories. These studies are a subtype of cohort study that incorporate longitudinal repeated measurement of an outcome measure. Statistical analyses of these data must account for correlated measurements in individuals over time. Other studies make parallel measurements in individuals to assess differences between these measurements. Examples include comparing coagulation testing methodologies during surgery or disability scoring instruments after surgery. Bland-Altman analyses which test investigator-defined limits of agreement are an appropriate analysis technique when comparing tests, while scales are often compared using correlation on measures such as validity, reliability, and responsiveness. Some of these studies could equally be described as “experimental” (see later) because the investigators control the intervention.
Studies Assessing Practice Against a Gold Standard (“Audit”)
Audits are variants of cohort studies that involve assembling a cohort of patients and determining whether practice complies with an external standard. The term “audit” is sometimes used inaccurately to describe studies that determine the standard that clinical practice achieves (i.e., evaluation) or compare practice with an investigator-derived standard (i.e., research). The extent of compliance with the standard can be compared based on different exposures. Examples of audits include the compliance of venous thromboembolism and surgical site infection prophylaxis with national guidelines. An important issue for such studies is ensuring that the external standard is reasonably valid and accepted within the wider community.
Studies Developing and Validating Prediction Tools
The goal of these studies is to develop tools (e.g., scores, prediction models, risk calculators) that accurately predict outcomes in individual patients. A good clinical prediction tool should be simple to use, exhibit good discrimination (i.e., the extent to which the tool correctly assigns higher predicted risk estimates to individuals who experience the outcome), and demonstrate acceptable calibration (i.e., the extent to which the observed outcome event rates agree with event rates predicted by the tool). These studies first use statistical methods to evaluate associations between exposures (e.g., baseline characteristics, processes of care) and outcomes in one cohort of patients; use the results to develop scoring systems or prediction models; and then validate them in another cohort of patients. Cohort study datasets are the preferred sources of development cohorts because randomized controlled trial datasets may not be generalizable. Different statistical methods can be employed to develop these scores and models, including logistic regression modeling for categorical outcomes, Cox proportional hazards modeling for time to event outcomes, recursive partitioning (i.e., decision tree analysis), and machine learning techniques (e.g., artificial neural networks). Examples from the perioperative setting include the Assess Respiratory Risk in Surgical Patients in Catalonia (ARISCAT) score for predicting postoperative pulmonary complications, the American College of Surgeons National Surgical Quality Improvement Program (NSQIP) calculator for predicting surgical risk, and the Acute Physiology and Chronic Health Evaluation (APACHE) score for predicting hospital mortality in critically ill patients.
Surveys
Surveys collect information from individuals (patients, families, staff, students) or organizations (hospitals, universities, employers) about facts and attitudes. Most are cross-sectional studies assessing exposures and outcomes at the same time. Factual surveys ask for information or test knowledge. Attitudinal surveys ask about attitudes, beliefs, and intentions. Surveys can be descriptive (describing responses from the whole group) or analytic (comparing responses in sub-groups). Surveys must be carefully planned and executed in order to protect participants and provide reliable conclusions. Response bias (non-response, incorrect response, or untruthful response) is a particular issue with survey research and must be explicitly considered in survey design and reporting. A systematic review of 240 surveys published in six anesthesia journals revealed that reporting was inconsistent, particularly with respect to articulating a hypothesis, describing the design, calculating sample size, providing confidence intervals, and accounting for non-responders. Compliance with design and reporting checklists may improve the quality of survey research.
Health Services Research
Health services research (also known as health systems research or health policy and systems research) has been defined as a “multi-disciplinary field of scientific investigation that studies how social factors, financing systems, organizational structures and processes, health technologies, and personal behaviors affect access to health care, the quality and cost of health care, and ultimately our health and well-being. Its research domains are individuals, families, organizations, institutions, communities, and populations.” While typical clinical research focuses on the epidemiology, risk factors, prognosis, and interventions related to specific disease entities (e.g., ischemic heart disease, postoperative respiratory failure), health services research focuses on the delivery of health care. Health services research and clinical research differ with respect to their focus, but they have considerable overlap with respect to their research methodologies (i.e., how studies are designed to answer specific research questions). Thus, health services researchers also employ surveys, observational designs (as described previously), and experimental designs (as described in the section to follow). In addition, qualitative research methods are employed, such as thematic analyses of individual interviews and focus groups. Qualitative methods are particularly suited to identifying potential underlying reasons for clinician and patient behaviors within healthcare settings. Examples of health services research in the perioperative setting include a retrospective cohort study to evaluate variation in rates of preoperative medical consultation for major surgery, a prospective cohort study of critical care utilization after major surgery, a mixed qualitative-quantitative methods study of a standardized operating room to intensive care unit handoff process, and a stepped wedge cluster randomized trial of a multifaceted implementation of perioperative safety guidelines.
Experimental Studies
In experimental studies, the investigators do not let nature (or clinical care) take its course, but actively intervene to test a new intervention. Experimental studies are almost always of a parallel group design where patients or clusters of patients are allocated to an intervention or control treatment (i.e., placebo, usual care, or current best practice) by the investigators and then tested for outcomes ( Table 89.1 ). Newer designs include cluster randomized, factorial, stepped wedge, and adaptive studies.
Allocation | ||
---|---|---|
Timing of Data Collection | Randomized | Nonrandomized |
After intervention | Any baseline differences could bias the results. | Comparisons may be confounded by differences between departments or organizations at baseline. |
Before and after intervention | Allows for specific comparison of change net of any baseline differences. Enables comparisons to be made between sites that change most or least. | Controls for baseline differences possible. Rates of change are less confounded than cross-sectional data. |
Unrandomized Studies
If the investigator allocates the intervention or control to study patients in a nonrandom manner, this introduces selection bias and potential for imbalance between the groups at baseline in terms of risk for the primary outcome. Overestimation or underestimation of the true treatment effect may then occur.
Quasi-Randomized Studies
Quasi-randomized (or quasi-experimental) studies attempt to select patients for the intervention or control in a less obvious but still nonrandom manner, for example, by surgical specialty, day of the week, date of birth, or by using a cut-off score for a certain characteristic. Quasi-randomized designs are seldom considered acceptable in contemporary clinical research because it is very hard to conceal the allocation, prevent selection bias, and ensure blinding. Some quasi-randomized designs may allow limited inference about causality (e.g., those that include a pre-test in the intervention and control groups to establish comparability). Sometimes randomization is not possible and quasi-randomized designs may be the only way to address the research question. The Risk Of Bias In Non-randomized Studies of Interventions (ROBINS-I) tool helps readers to assess the quality of observational studies that evaluate interventions without the benefit of randomization.
The before-and-after study is a simple and tempting study design that has been used for centuries to compare patient status before and after a new intervention. For example, John Snow compared the incidences of cholera in a neighborhood of London before and after removing the local water pump handle. In modern research, these studies can be conducted entirely prospectively, partly prospectively (the “after” group), partly retrospectively (the “before” group), or wholly retrospectively. The “before” intervention is often current usual care and the “after” intervention is often a new treatment. For example, an enhanced recovery pathway for colectomy patients was reported to be associated with decreased opioid administration, earlier return of bowel function, and reduced length of hospital stay compared to the old approach. The methodological challenge here is the influence of time: these improvements could have resulted from the new pathway or from changes in case-mix or unmeasured parallel improvements in care. If the effects of such parallel improvements are large enough, they might even obscure the fact that the new pathway is actually worse in terms of patient outcomes. Difference-in-differences approaches can be used to address time-related trends in outcomes. These approaches assume that trends unrelated to the exposure are the same in both groups. Before-and-after studies are particularly vulnerable to the Hawthorne effect (i.e., the observation that people perform better when they know that they are being observed). The observational study design in which data are collected in individual patients before and after an intervention is addressed above.
Cross-Over Studies
The cross-over study is a more robust form of the before-and-after design. Here each patient receives all the interventions and control treatments, separated by wash-out periods to eliminate carry-over effects from the previous treatment. Cross-over studies are well suited to patients taking chronic medications (e.g., analgesics for chronic pain ). A major benefit of the cross-over design is that patients are their own controls, which eliminates the confounding issues seen in parallel group designs. The order in which patients receive the intervention and control treatments can be nonrandom or random (the latter will eliminate time effects). In addition, administration of the intervention and control treatments can be blinded to eliminate placebo and nocebo effects.
Randomized Studies
The ascendency of large simple randomized trials as the most robust form of primary research in anesthesia, intensive care, and pain medicine had its roots in the evidence-based medicine movement. The cardinal features of a high-quality randomized controlled trial are successful randomization and blinding, and a sufficient sample size to reveal clinically important treatment effects on patient-centered outcomes.
Randomization
The many methodological problems associated with observational studies and nonrandomized experimental studies boil down to the distorting influence of confounders (see above). The purpose of random allocation is to ensure that all confounders (known and unknown) are evenly distributed between the intervention and control groups at baseline. The success of randomization in evenly distributing these characteristics is critically dependent on the sample size of the study. After successful randomization any remaining differences in baseline characteristics will be the result of chance alone and patients in the intervention and control groups should have the same probability of experiencing the primary outcome. The study will then have a small likelihood of distorted results from random and systematic error. This is the primary reason that large randomized trials are considered the gold standard for experimental studies in medicine. Randomization schedules can be generated using simple, block, stratified, or covariate adjusted techniques. Allocation concealment is an essential step in preventing selection bias (i.e., recruiting patients based on knowledge of the treatment to which the next study patient will be allocated).
Blinding
Randomization can effectively eliminate confounding, but it is not enough to guarantee unbiased results. Simply knowing about the treatment allocation can influence the behavior of the investigators, subsequent clinical management by the treating team, and even the symptoms that the patients experience, creating a new imbalance in confounders and undoing the benefits of randomization. The solution here is blinding: hiding the treatment allocation from the observers collecting study data, the patient, and/or the treating team. Blinding observers, patients, and treating team members in phase III and IV drug trials ( Box 89.2 ) is the norm, as matched blinded experimental and control drugs are easy (although not inexpensive) to produce. Other interventions are harder to blind (e.g., intravenous albumin solutions ) and others cannot be blinded (e.g., epidural local anesthetics in chronic pain patients ). In such cases, it is important to conceal allocation as long as possible to prevent this knowledge from influencing processes of care. Every effort should be made to blind outcome observers in randomized trials.
Phase I
Phase I trials test a new intervention for the first time in a small group of participants to evaluate safety.
Phase II
Phase II clinical trials study a new intervention in a larger group of participants to determine efficacy and further evaluate safety.
Phase III
Phase III trials study the efficacy of a new intervention in a large group of people by comparing it to other interventions or routine care and monitor adverse effects.
Phase IV
Phase IV trials study an intervention after it has been marketed, and monitor its effectiveness in the general population, collect information about adverse effects, and investigate its use in a different condition or in combination with other therapies.
Superiority, Equivalence, and Non-Inferiority
Randomized trials are often geared toward demonstrating that the intervention results in better outcomes than the control (“superiority”). However, a randomized trial can be designed to demonstrate that the two treatments produce the same results (“equivalent”) or that the one produces results at least as good as the other (“non-inferiority”). Equivalence and noninferiority designs are useful if the new intervention might be simpler, safer, and/or cheaper than the current treatment, and demonstrating equivalence or non-inferiority would be sufficient to change practice.
Factorial Designs
Factorial designs allow testing of more than one intervention in a single clinical trial. Rather than performing a separate randomized trial for each intervention and each combination, patients in factorial studies are separately randomized to two or more different interventions (that is, they receive none, some, or all of the experimental interventions). This design is efficient and allows testing of interactions between the interventions. For example, in a factorial trial of six interventions for the prevention of postoperative nausea and vomiting, 4000 patients were randomized to one of 64 possible combinations of six antiemetics. This design allowed the investigators to conclude that these antiemetics were similarly effective and acted independently. In another example, 5784 patients were randomized to aspirin or placebo, and in a partial factorial, 4662 of these patients were also randomized to tranexamic acid or placebo. This allowed the investigators to determine that there were no interactions between the interventions with respect to death, thrombotic complications, or major hemorrhage.
Cluster Randomized Designs
Most studies are randomized at the patient level. Cluster randomized trial designs are needed when randomization at the patient level is not possible or is methodologically unsound. This is particularly the case for process of care interventions because the fidelity of the intervention is dependent on the execution by the treating team and blinding is often not possible. Examples include cross-over cluster randomized trials of selective decontamination of the gut in intensive care patients (even though the intervention is only applied in patients randomized to the treatment group, changes to bacterial colonization profiles will extend to all the patients in the unit) and randomized trials of the introduction of medical emergency teams to treat deteriorating hospital patients.
Stepped Wedge Designs
Cluster randomization means that some hospitals or clinical areas implement the new intervention, and some must remain with the existing model of care. Before-and-after studies are contaminated by the effects of time and cross-over cluster randomized trials have the disadvantage that clusters that were first randomized to the new intervention must revert to the existing model of care. A stepped wedge design circumvents some of these ethical and methodological issues by ensuring that each cluster first provides data for the control condition and then crosses over to the new intervention ( Fig. 89.3 ). This may improve recruitment of centers and patients. The duration of these periods differs for every cluster, but at the end of the study period there will be an equal amount of data from control and intervention periods. This eliminates—to a large extent—the effects of time. Stepped wedge designs were originally used in vaccination studies, exploiting the natural limitation that vaccination programs can never be rolled out over an entire region in a very short time frame. They are considered alternatives for randomized trials of complex interventions, provided that there is a high likelihood of a positive effect of the intervention and a very low risk of harm.

Analyses of Published Research
Systematic Reviews
The explosion in published medical knowledge and the requirement for quick answers to urgent clinical questions means that reliable synthesis of the medical literature is invaluable. Traditional narrative reviews are prone to author biases (sometimes even outright conflicts of interest), because authors can “cherry pick” the literature for evidence supporting their own opinions. Systematic reviews pose an explicit research question and publish a transparent search strategy that every reader can replicate and update. The Preferred Reporting Items for Systematic Reviews and Meta-Analyses (PRISMA) statement is available to guide authors of systematic reviews. However, the quality of reporting and methodological quality of reviews remains inconsistent.
Metaanalyses
When multiple randomized trials have addressed similar research questions, interventions, and outcomes, the results can be mathematically aggregated in a metaanalysis, the goal of which is to estimate the aggregated effect of the intervention. A high-quality metaanalysis of large well-conducted randomized trials is considered the highest level of evidence to guide practice, although results of metaanalyses do not always agree with subsequent very large trials. The Cochrane Collaboration is dedicated to performing systematic reviews and metaanalyses that inform clinical practice. The Collaboration’s software tools (“RevMan” and “Covidence”) generate analyses, graphs, and metrics about the quality of included studies. Funnel plots of treatment effect against study precision are used to detect publication bias toward studies with large treatment effects (typical of small trials) ( Fig. 89.4 ).

Individual Patient Metaanalysis
A more powerful form of metaanalysis aggregates results using individual patient data, rather than aggregated data from the included studies. The benefits of this approach are better characterization of outcomes and the opportunity to perform new subgroup analyses. The challenges of this approach include obtaining the raw data (with one paper reporting that only 25% of these metaanalyses retrieved all individual patient data ) and reliably merging trial databases containing the original data into the new pooled database. A standard aggregated data metaanalysis will illuminate whether an individual patient data metaanalysis will yield sufficient new information to justify the additional time and effort.
Study Protocol
A carefully designed protocol is the foundation of all clinical research, facilitating the review, conduct, and eventual publication of the study ( Fig. 89.5 ). However protocols are often incomplete and inconsistent with their corresponding published reports. In response, templates have been developed, including by the World Health Organisation, the International Council for Harmonisation, national research funding agencies, and the Equator Network (the Standard Protocol Items: Recommendations for Interventional Trials [SPIRIT] statement ). The effectiveness of these templates in improving the quality of protocols is yet to be determined. Amendments to clinical research protocols must be approved by the coordinating institutional review board (human research ethics committee) and must be reflected in the trial’s registration. The International Committee of Medical Journal Editors recommends that investigators make their protocols publicly available before any results are published, for example by posting them on institutional websites or publishing them in peer-reviewed journals.

Hypotheses
All clinical research—from a case report through qualitative and observational projects to a multicenter clinical trial—begins with a research question. These questions arise from a myriad of sources: the existing literature, the previous work of the investigators, interactions with colleagues, observations during clinical work, and discussions with patients and their families. A well formulated research question guides the literature review (which establishes the need for the study); informs the study design, methods, and sample size; and limits the potential for error and bias. The Population-Intervention-Comparator-Outcome-Timeframe-Setting (PICOTS) format is a popular framework among clinical researchers and improves the quality of research questions. In an observational study, the “intervention” and “comparator” can be patient-based (e.g., with or without intraoperative hypotension) or care-based (e.g., treated in a metropolitan or rural hospital). In a clinical trial, the intervention and comparator are randomized. During protocol development, research questions are transformed into formal hypotheses. The structure of the hypothesis depends on whether the investigators predict that one group will have superior, equivalent, or non-inferior outcomes to the others. Regardless of this prediction, two-sided hypothesis testing is preferable: that is, entertaining the possibility that standard treatment is better or that the treatments are not equivalent.
Population
Selection of participants depends on the purpose and context of the study, and is a key differentiator between explanatory and pragmatic studies. Explanatory (efficacy) studies, such as observational studies of new drugs and devices or phase I to III clinical trials, include highly selected patients in highly controlled settings, to reduce variability between patients and maximize treatment effects. The generalizability of explanatory studies therefore is limited. Pragmatic (effectiveness) studies, such as phase IV clinical trials, include typical patients receiving real-world care. Random sampling is approximated by approaching all patients who have indications for the treatment and who present themselves for care. In practice, however, only a subset of patients is approached. Further nonrandom selection occurs when patients decline to participate or fail to complete data collection. Although national funders promote diversity among participants, children, elderly people, pregnant and lactating women (indeed, women in general), culturally and linguistically diverse people, and people with disabilities are still underrepresented in clinical research. An additional factor is failure to identify underrepresented people (e.g., transgender people) among recruited participants. Ideally studies should be powered to allow analysis of major sub-group effects.
Interventions and Comparators
Nearly all clinical research involves an intervention (e.g., a drug, device, procedure, diagnostic test, bundle of care, etc.). In observational studies, the intervention is administered as part of usual care, whereas in experimental studies the intervention is administered by or on behalf of the investigators. Many studies involving an intervention also involve a comparator (e.g., placebo, usual care, or current best practice). The comparator should be the best proven intervention, except when no such intervention exists. In this case, a placebo may be appropriate. Usual care needs to be defined at the outset and monitored for change over time. Current best practice needs to be supported by guidelines and ensured during the study. For a study to be ethical, there must be uncertainty in the expert community about the relative merits of the intervention(s) and comparator(s), and the study must be designed to resolve it (clinical equipoise). Complex perioperative interventions and comparators may not be fully or properly implemented in pragmatic studies, and this variation may be nonrandom. For this reason, patients are usually analyzed in the groups to which they are assigned (intention-to-treat analysis).
Outcomes
Outcomes are events that may result from or be associated with an action taken during a study. Ideally, outcomes in clinical research influence the way clinicians practice and the choices that patients make. Although patient-reported outcomes are subjective, they can greatly enhance the impact of the study if properly developed and implemented. Binary outcomes that can be expressed as probabilities are easier to interpret than continuous outcomes, which can be converted to binary outcomes by defining an appropriately validated cut-off value. Composite outcomes can be a useful means of assessing the impact of an event or intervention but can be misleading if the components are dissimilar in frequency, magnitude, direction of effect, or importance. Surrogate outcomes occur between the event or intervention and the true outcome, and are used to draw conclusions about the true outcome. To be valid, the relationship between the surrogate and true outcome must be precisely defined. Occasionally surrogate outcomes indicate the direction and magnitude of the effect of the intervention on the true outcome (e.g., the effect of anti-arrhythmic drugs on ventricular ectopic beats and sudden death post-myocardial infarction ). The primary outcome is the focus of the study and will be the basis of the sample size calculation. Secondary and safety outcomes should reflect the important beneficial and adverse effects of the event or intervention. Careful definition of outcomes and the timing of their measurement are fundamental to protocol development.
Sample Size
A study needs a sufficient number of participants to provide reliable conclusions about the specified outcomes and treatment effects. If the number of study participants is too low, the investigators could incorrectly conclude that there is no treatment effect. If the number of study participants is too large, it may delay the availability of information that is vital to patient care. In both cases resources will be wasted and participants will be exposed to unnecessary risk. A sample size calculation therefore should be conducted in the design phase of all studies. In qualitative and observational research, investigators should justify the reasons for their sampling frame (the population from which the sample is drawn). This should be based on the availability of interviewees or relevant data in an appropriate format, or the investigators’ expectations about the numbers of eligible participants and/or their estimates of acceptable 95% confidence intervals around the incidence of the primary outcome. In comparative studies, sample size calculations are based on the expected difference in the primary outcome between the groups (effect size), the variance in the effect size (for continuous variables), and the risk that the investigators are willing to accept of false positive (α; type 1 error) and false negative (β; type 2 error) findings. Effect sizes and variances can be estimated from the literature, pilot studies, statistical methods, or opinions about minimal clinically important differences. The planned sample size will also depend on the number of groups, number of anticipated dropouts, and statistical analyses planned. The protocol should provide sufficient information to allow replication of quantitative sample size calculations. In the anesthesia literature this is frequently not the case. Post hoc calculation of statistical power using the results of a trial is inappropriate; the width of the confidence intervals around the primary outcome is a better indicator of the reliability of the result.
Data Analysis Plan
The details of data analysis are outside the scope of this chapter, and increasingly outside the scope of clinician researchers and reviewers. Statistics training or collaboration with biostatisticians or experts in qualitative data analysis is commonly required to reach the level of statistical excellence required by regulators, funders, and journals. Statistical input is vital during the planning of clinical research, particularly in relation to the sample size calculation. The protocol should also include a plan for description of the data and analyses of the primary and secondary outcomes, subgroup and adjusted analyses, sensitivity analyses, interim analyses and stopping rules, and protocol adherence, as applicable. The detailed statistical analysis plan for large observational studies and clinical trials is often published before the unblinded data are available, and at least should be predefined. Peer-reviewed journals may require submission of the statistical analysis plan with the manuscript, require statistical checklists, and employ statistical editorial teams to assist with the evaluation of manuscripts.
Supporting Studies
Feasibility and Pilot Studies
Most clinical studies are inspired by prior research of some kind. Increasingly, however, preliminary work is specifically undertaken to demonstrate the viability of a future large observational or experimental study. A feasibility study tests whether a future study can be done, examining the knowledge and interest of practitioners, availability of eligible patients, their willingness to participate, ease of applying the intervention and collecting data, qualities of the primary outcome, and resource requirements of the study. A pilot study is a type of feasibility study that tests the proposed hypotheses of a future study, without being scaled to test the effectiveness of interventions nor the strength of associations. Pilot studies are frequently used to inform sample size calculations for the future studies, although this process may be flawed. The rationale for the sample size of the feasibility study itself should be congruent with the feasibility objectives, but need not involve a formal sample size calculation. Pilot studies are subject to the same ethical and regulatory requirements as definitive studies.
Sub-Studies
Sub-studies are an efficient means of investigating additional research questions using additional data collected in subsets of participants in large clinical trials. They are subject to the same ethical and regulatory requirements as the main study, and ideally are planned simultaneously. Sub-studies can investigate additional outcomes related to the randomized intervention, in which case they can be considered nested randomized trials. They can investigate the associations of additional unrandomized exposures (e.g., biomarkers) with the same outcomes, in which case they can be considered nested cohort studies. Finally, they can investigate the associations of additional unrandomized exposures with additional outcomes: these are also nested cohort studies and are an efficient use of a unique cohort. The effect of additional randomized exposures is more properly evaluated using a factorial study design. Principal design considerations for sub-studies include adequate sample size and limiting the burden on investigators and patients.
Sub-Analyses
Sub-analyses are an efficient means of investigating additional research questions using data collected for another primary research purpose. Some sub-analyses are planned before the main study begins (e.g., population subgroup analyses). Others are planned after the data have been collected or the main results have been published and may address unanticipated events or discoveries. In any case the statistical analysis plan should be finalized before the analyses begin. Sub-analyses can investigate the associations of unrandomized exposures (e.g., baseline characteristics, processes of care, measured variables) with the primary and secondary outcomes, or the detailed effects of the randomized intervention on secondary outcomes. Propensity scoring methods are increasingly used to determine the probability of a participant receiving a nonrandomized treatment given a particular set of baseline characteristics, and to compensate for this by stratifying, matching, weighting, or adjusting on the basis of the propensity score. However these methods are heavily reliant on the collection of appropriate and complete baseline data, and do not reduce confounding by unmeasured or unknown factors (e.g., the reasons the anesthesiologist chose a particular technique or defended a particular blood pressure).
Ethical and Regulatory Considerations
Ethics Approval
All research on humans must be conducted within a system that ensures the safety and privacy of the participants. This system can be local, national, or multinational. The degree of scrutiny is based on the potential for risk, discomfort, inconvenience, burden, and threats to privacy. Depending upon the jurisdiction, low-risk research (e.g., audits and surveys) may be conducted without review under policies that promote ethical conduct, or under expedited review processes. Research that is not low-risk is considered by an institutional review board. The board approves the processes for obtaining consent or may waive or qualify consent if the risk to participants is low. Deferred consent is also possible in urgent care research in some jurisdictions. Approaching patients for the first time about a study on the day of surgery is acceptable to patients, but participation rates are lower than before the day of surgery.
Registration
Clinical trial registration was introduced in response to concerns about publication bias and selective reporting. Additional aims of registration include reducing waste from unnecessary duplication of studies and improving access to clinical trial participation and results for patients. Initial efforts involved mandating registration of a minimum dataset of protocol information before the enrollment of the first patient. Subsequently efforts were expanded to mandating public disclosure of aggregated trial results, including those that were negative or inconclusive. These efforts have not been completely successful. For example, in a report about anesthesiology registration, although rates of registration had improved since 2007, 62% of clinical trials published in six specialty journals in 2015 were still inadequately registered. Further culture change among funders, institutional review boards, investigators, and publishers will be required. Registration of observational research is encouraged, but is currently voluntary, partly because of concerns that registration may stifle exploratory analyses.
Regulatory Approval
Federal, national, and state government agencies regulate the manufacture, import/export, supply, marketing, and surveillance of drugs and medical devices, with the aim of optimizing access to safe and effective therapeutic goods. There is some harmonization between jurisdictions. Regulatory authorization is required for clinical trials of therapeutic goods that are not yet approved (phase I-III trials) or are being tested for an indication outside the current approval (phase IV trials). The sponsor, institutional review board, and regulator collaborate in protecting patients who are exposed to unapproved therapeutic goods during clinical trials. This collaboration can be especially complex for international investigator-initiated clinical trials, and must be carefully considered in the planning phase.
Data Sharing
Sharing of individual patient data from clinical trials is in the public interest, on scientific, economic, and ethical grounds. Third parties may wish to verify trial results, correct errors, explore new hypotheses, or use the data in individual patient data metaanalyses. Funding agencies, publishers, investigators, and the pharmaceutical industry have issued position statements about data sharing. Retreating from its initial proposal of mandating data sharing, the International Committee of Medical Journal Editors currently requires investigators to include a data sharing plan in the trial’s registration and a data sharing statement in the primary manuscript. These statements and plans must disclose whether, what, with whom, and how data will be shared. This position recognizes the current lack of policies, resources, and culture that will protect the interests of patients and investigators. In order to be effective and responsible, data sharing must be planned from the outset of a clinical trial, as it involves clarifying the need for patient consent, constructing appropriate data management systems, and securing adequate funding.
Study Management
Financial Management
Good clinical practice requires investigators and sponsors to document and agree on the financial aspects of a study. Budgets and contracts are approved as part of the research governance process. All clinical research has a cost: even a case report requires retrieval of medical records, preparation of illustrations, and investment of investigator time. The cost of research is increasing in line with the increased size and complexity of clinical research. At the same time, the ability or inclination of health services to absorb costs that are not directly related to patient care is decreasing. Investigators therefore must obtain funding from other sources, including government agencies, commercial enterprises, and philanthropic organizations. This process can be time-consuming and stressful. One study estimated that 34 working days of the principal writer’s time was spent preparing each new proposal for a national funding round. Only 20% of these proposals were funded. Streamlined and flexible application processes, and rules regarding resubmission of unsuccessful proposals, may reduce workload and increase success.
Data Management
The protocol should list each item of data to be collected, its source, and the timing of measurement. The ethics review process addresses whether the proposed data collection meets privacy and data security requirements. In explanatory studies, such as phase I-III clinical trials, the volume of data collected can be large, whereas in pragmatic studies data collection should be limited to vital measurements only. Three main options are available for data collection: (1) a bespoke case report form; (2) data extraction from existing sources (such as medical records, registries, and administrative databases); and (3) a hybrid approach. Case report forms can be printed or electronic, with electronic forms offering mechanisms to ensure complete and accurate data entry. Data will then be transferred to a database that is usually established specifically for the study. Increasingly anesthesia research protocols require linkage of records of individual patients or groups across unrelated databases, requiring the input of health informaticians and raising issues of privacy and data security.
Human Resource Management
The human resources associated with clinical research include the investigators, trial coordinators, treating clinicians, and patients. Ethics and governance processes address whether the legitimate interests and rights of these parties are protected during the study. Investigators and trial coordinators should be suitability qualified, experienced, and competent for the research they propose to undertake, and ideally should have International Council for Harmonisation E6 Good Clinical Practice certification (a requirement of some funding bodies ). Clinical trial networks can play a big role in nurturing careers and fostering collaboration. Treating clinicians need to be advised about their role in implementing the protocol and be engaged in ensuring the success of the study. This is especially important in protocols that require the continuous attention of the treating clinician during an anesthetic or critical care unit stay. Recruiting patients to anesthesia and critical care studies can be difficult, because of time constraints and multiple competing priorities. A systematic review of recruitment strategies identified that open rather than blinded treatment allocation, and phone follow-up to written invitations to participate, significantly improved recruitment. Loss to follow-up is very low in anesthesia and critical care studies, because time frames for data collection are often short and patients are in the operating room or hospital for most or all of the study. A systematic review of retention strategies in ambulatory settings reported that only monetary incentives improved retention.
Adverse Event Reporting
Adverse event reporting is a crucial step in ensuring the safety of participants in all clinical research ( Box 89.3 ). An adverse event is any unfavorable and unintended occurrence associated with the use of a medicinal product, whether or not it is related to that product. Adverse events can be categorized by severity (intensity), seriousness (effect on patient outcome), expectedness (observed before), and causality (attributability to the medicinal product). Expedited reporting of adverse events to regulators and institutional review boards is an essential part of phase I to III trials. For phase IV trials, universal safety endpoints and unexpected adverse events are reported periodically by the trial’s data and safety monitoring board. Only serious unexpected events that would be reported as part of routine clinical care are reported immediately to regulators and institutional review boards. The primary report of a clinical trial should include a table of adverse events by system and/or severity. Adverse event reporting is burdensome and costly, and therefore should be aligned with the risk to patient safety of the medicinal product.
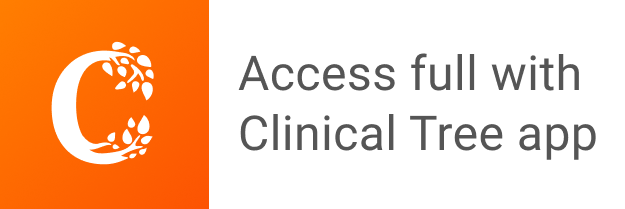