Introduction
Artificial intelligence has been defined as the study of algorithms which give machines the ability to reason and perform functions such as problem-solving, object and word recognition, inference of world states, and decision-making. In simple words, it is the human handshake with a machine.
Artificial intelligence in biosciences, including anesthesiology, critical care, and pain medicine, brings about a Brobdingnagian amount of excitement and expectation for the betterment of current treatment strategies.
Machine learning involving artificial intelligence helps develop algorithms that can assist devices in creating choices relating to the administration of anesthetic agents and thus determine numerous issues related to anesthetic management.
History of Artificial Intelligence
History of artificial intelligence dates back to
1950 when Mayo and Bickford used early machines for self-administration of volatile anesthetic agents via reading electroencephalograms (EEG) to monitor the depth of anesthesia. In this era, artificial intelligence created a phase of excitement among the various field of medicine. Then, came the phase of machine learning in the 1980s in which Servo anesthesia theory was additionally postulated, which is also the early integration of artificial intelligence into anesthesiology. Throughout the 1990s, target-controlled drug infusion (TCI) was brought into action, which used artificial intelligence technology for its practical demand. Since 2010, it is the section of deep learning which is gaining importance in recent years. Recently, automatic drug delivery systems of anesthetic robots, the assistant operating system of anesthetic technology robots, and the automatic system of anesthesia evaluation and diagnosis robots have developed rapidly.
Working Principle of Artificial Intelligence
There are three approaches to machine learning, that is, supervised learning, unsupervised learning, and reinforcement learning.
Supervised learning: It is the machine learning task of learning a function that maps an input to an output based on, for, example input-output pairs. In supervised learning, every example is a pair consisting of an associate degree input object (typically a vector) and the desired output value (also known as the supervisory signal). An example of a supervised learning study is to use electronic health records of patients to identify postinduction hypotension.
Unsupervised learning: It is a sort of machine learning that looks for antecedently undetected patterns in a data set with no pre-existing labels and minimal human supervision. In contrast to supervised learning that typically uses human-labeled data, unsupervised learning, additionally far-famed as self-organization, allows for modeling of probability densities over inputs. An associate example of unsupervised learning is a study carried out by Bisgin et al who used this technique to obtain data from Food and Drug Administration (FDA) drug labels like specific adverse events to classify drugs consequently.
Reinforcement learning: It refers to the method by which an algorithm is asked to aim at a particular task (e.g., deliver inhalational anesthesia to a patient) and to learn from its ensuant mistakes and successes.
An associate degree example of reinforcement is to assess a patient’s bispectral index (BIS) and mean arterial pressure (MAP) to manage the propofol infusion rates (in a simulated patient model).
Numerous techniques are utilized by the approaches mentioned above to machine learning. Typically used techniques are as follows:
Fuzzy logic: 1965 was the year when Fuzzy theory was published. A device that uses “fuzzy logic” is programmed to resemble human judgment; in associate degree, accelerated means even in complicated situations. Classical or normal logic allows for correct mathematical value of say 1.0 and incorrect value as 0.0, while fuzzy logic mathematical value might dwell between 0.0 and 1.0, that is, the partial value. Thereafter, comparison could also be created between probable theory and the extent to that a sentence is correct. For example, the probable theory could be “a laparoscopic cholecystectomy procedure will be scheduled for tomorrow,” while a true or real theory maybe “there is 90% chance of laparoscopic cholecystectomy procedure being scheduled tomorrow.” Thus, this sort of technique is quite similar to that of human decision-making with discrete or inaccurate information.
Classical machine learning: Here, characteristics are developed or chosen by the authority to help the algorithms explore the sophisticated data. The decision trees can be used to predict total patient-controlled analgesia (PCA) consumption from features such as patient demographics, vital signs, aspects of their medical history, surgery type, and PCA doses delivered with the promise of using such approaches to optimize PCA dosing regimens.
Neural networks and deep learning: Neural networks are driven by human nervous systems, transmitting signals in clusters of biological or, say, computational units, that is, neurons. Here, one network constitutes associate degree input bundle of neurons which has characteristics of the information. It has one concealed layer of connection which carries out the numerical modification of the initial data. The third layer is the output which ultimately provides the result. As compared to classical machine learning, where characteristics are hand-designed, deep learning has an auto-learning character based on the data itself. In anesthesiology, examples of neural networks and deep learning are monitoring of depth of anesthesia and control of anesthesia delivery.
Bayesian methods: This technique provides an account of the probability of an incident which is based on experience or data about factors that may influence that incident.
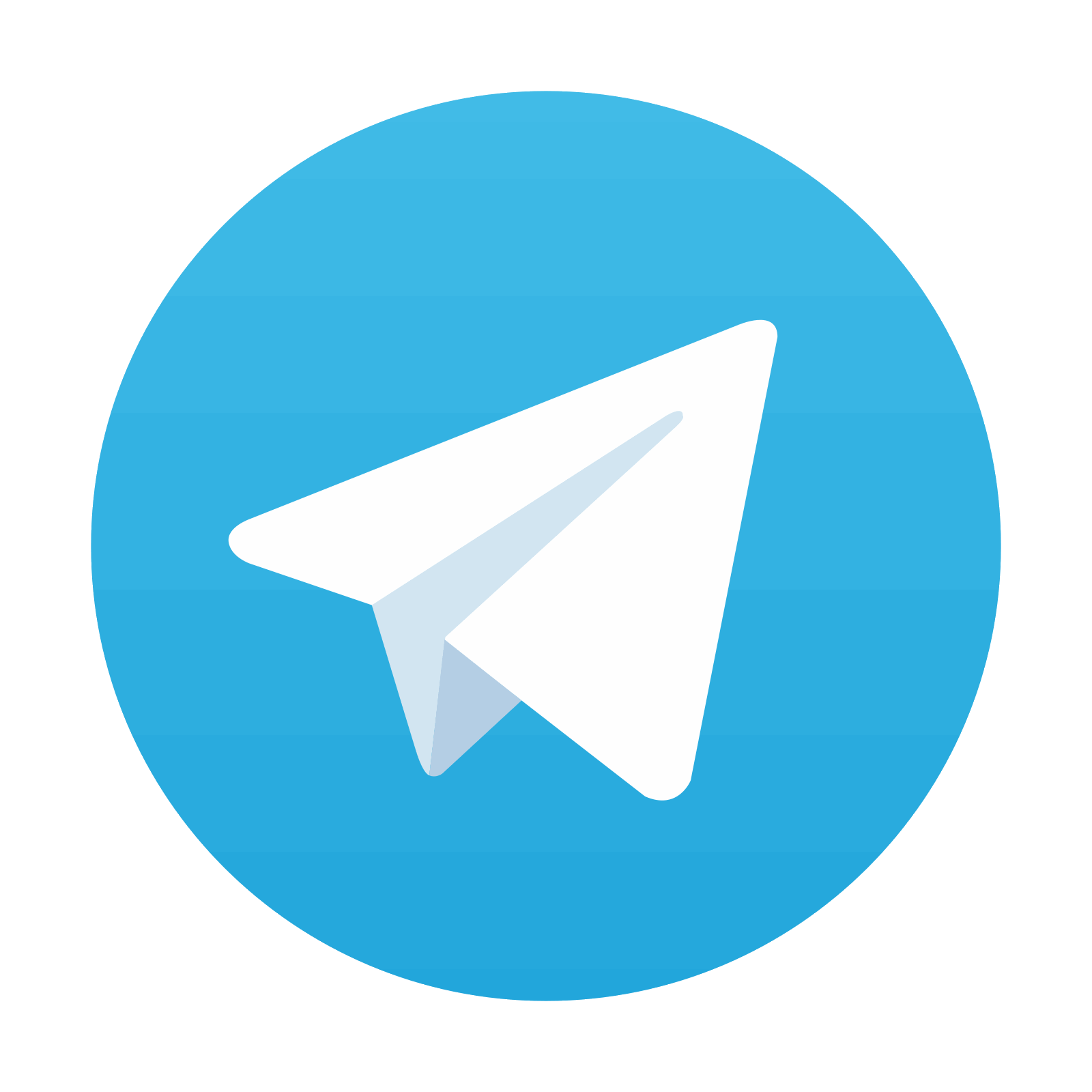
Stay updated, free articles. Join our Telegram channel

Full access? Get Clinical Tree
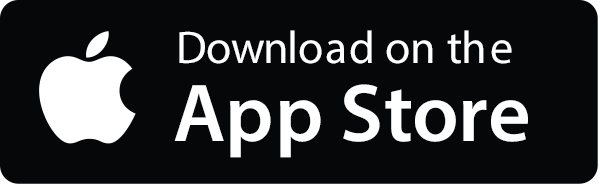
