Key Points
Risk stratification for surgery facilitates informed consent and appropriate patient selection.
Several tools exist for assessing operative risk, and each has strengths and weaknesses; which method is used depends on a balance between ease of use and accuracy.
Communication of individual risk using a combination of objective measures, clinical judgement and teamwork can help meet patients’ expectations.
High-quality decision-making is central to high-quality perioperative care.
Patients’ preferences and values should form the core of decision-making.
Despite widespread endorsement, the implementation of shared decision-making is slow.
Ask your patients ‘what matters to you?’
Introduction
Surgery and risk go hand in hand. It is of paramount importance to accurately quantify this risk on an individual basis, which in turn will facilitate informed consent and appropriate patient selection, enabling optimal resource allocation. In this chapter we will examine the importance of risk prediction, looking at selected risk-stratification tools, and discuss the role of shared decision-making.
Getting Risk Prediction Right – Why it Matters
Risk stratification needs not only to be accurate, but meaningful for the patient so that their needs and expectations can be met (Moonesinghe et al., 2013). With an ageing population and improved chronic disease management, a rising proportion of patients presenting for surgical options is elderly and has multiple co-morbidities. However, with advances in healthcare management, there are also multiple options for managing disease: for example, a patient presenting with symptomatic knee osteoarthritis may be offered physiotherapy, simple analgesics, arthroscopy or an uni-compartmental or total knee replacement. Understanding the relative risks and benefits of surgical care, based on individualised prediction of patient-centred outcomes, should ultimately empower patients and providers to work together towards improved outcomes.
Clear and sensitive communication of risk to patients and their families can take time, and should ideally be performed well in advance of surgery, using objective risk-stratification tools alongside clinical judgement. Clear documentation of all discussions which have taken place is very important and a key recommendation from the UK’s Knowing the Risk report, published in 2011 by the National Confidential Enquiry into Patient Outcome and Death (NCEPOD), was that mortality risk assessment should be made explicit to patients before surgery and clearly documented on the consent form (Findlay, 2011). However, communicating the risk of perioperative mortality does not necessarily mean that the full risks of perioperative care have been discussed. It has been shown that major perioperative morbidity afflicts up to 10 times more patients than inpatient death, and furthermore, it is associated with reduced long-term survival (Khuri, 2005; Moonesinghe, 2013); additionally, those who suffer post-operative complications may have a reduction in their health-related quality of life (Derogar et al., 2012). Patients who have a difficult post-operative period will inevitably have a longer length of hospital stay, utilising more health resources.
In addition to the advantages presented here for risk stratification, a related concept – risk or case-mix adjustment – may be applied using risk-prediction tools, and enables fair comparison of surgical outcomes between providers (Moonesinghe et al., 2013). Transparency and accuracy of reporting enables feedback, learning and advancement of clinical care (Chand et al., 2007); however, the consequences of presenting non-risk-adjusted outcomes may be considerable. For example, data can be easily dismissed as unfairly ‘biased’, and of greater concern, surgeons may be deterred from operating on high-risk patients (Westaby, Baig and Pepper, 2015).
Methods of Assessing Risk
There are many methods for assessing operative risk; this chapter focusses on selected risk-stratification tools. The development of risk scores and risk-prediction models is usually based on multivariable analyses of risk factors for a specific outcome (Moonesinghe et al., 2013). The calculation of risk scores (simple, linear scales of increasing risk) is relatively simple: such scores are quick to use in the clinical setting, and can allow comparison between patients; however, scores do not give individualised risk prediction for specific outcomes. Examples of risk scores are the American Society of Anesthesiologists’ Physical Status Score (Saklad, 1941) and the Lee Revised Cardiac Risk Index (Lee et al., 1999). Alternatively, systems such as the Physiological and Operative Severity Score for enumeration of Morbidity and Mortality (POSSUM) (Copeland, Jones and Walters, 1991) may give more information to patients and clinicians, by estimating an individual probability of the risk of a particular outcome by entering patients’ data into a multivariable regression model. While such models may provide more granular information, they can be time-consuming and complex to use on a daily basis (Moonesinghe et al., 2013). Additionally, some models have 30 variables or more: these can be cumbersome to use, and the constituent data may not always be routinely available; thus, clinicians may be less likely to integrate such complex instruments into routine practice (Nashef et al., 1999).
A single risk-assessment system would ideally be accurate for all types of surgery (including both elective or emergency), cost neutral and easy to use, and all variables for the score would be available in the preoperative period (Barnett and Moonesinghe, 2011). Current methods of assessing risk require us to strike a balance between ease of use and accuracy (Moonesinghe et al., 2013).
Understanding Accuracy of Risk-Stratification Tools: Discrimination and Calibration
Two characteristics used to judge the accuracy of a scoring system are discrimination and calibration. Discrimination describes the ability of a tool to correctly identify cases which have a particular outcome. It is usually presented as the area under a receiver-operator-characteristic curve (AUROC), which summarises sensitivity and specificity. Calibration describes how closely the number of predicted cases of an outcome matches the actual number observed. Calibration is usually expressed as a summary statistic for observed: expected ratios over a range of different predicted risks (for example, the Hosmer-Lemeshow statistic) where a p value of greater than 0.05 indicates adequate calibration.
Prediction of Organ-Specific Outcomes
The Lee Revised Cardiac Risk Index
In 1977, Goldman and colleagues developed a cardiac risk score for predicting individual perioperative cardiovascular morbidity and mortality in non-cardiac procedures using nine variables (Goldman et al., 1977). This was revised in 1999 to a score comprising six independent variables, the Lee Revised Cardiac Risk Index (RCRI) (Lee et al., 1999). A systematic review has studied the Lee RCRI and found it to be moderately good at predicting complications after non-cardiac surgery; however, it was less accurate at predicting mortality (Ford, Beattie and Wijeysundera, 2010). Limitations of the review included varied definitions of cardiac events and general low methodological quality of the studies included. While cardiac morbidity defined against historical criteria (e.g. myocardial infarction or heart failure) is important, it is also uncommon when compared with other perioperative complications. However, the recognition that minor fluctuations in cardiac biomarkers may herald myocardial injury, which has implications for post-operative survival, may lead to the development of risk-prediction systems for the new diagnostic criteria of myocardial injury after non-cardiac surgery (MINS) (Botto et al., 2014).
Prediction of Adverse Outcome in Specific Patient Populations
The Nottingham Hip Fracture Score
The Nottingham hip fracture score (NHFS) was developed and validated in a single centre in 2007 (Maxwell, Moran and Moppett, 2008) on a dataset of 5000 patients over a 7-year period. Age, gender, presence of co-morbidities, abbreviated mental test score, haemoglobin on admission, history of malignancy and domestic status (institutional residence) were all found to be independently predictive of mortality within 30 days of surgery. The equation has since been updated after a larger cohort including 7290 patients in three centres was studied, making it more externally generalizable (Moppett et al., 2012). As with many other risk-prediction systems, accuracy varies over the range of observed risk: the original NHFS equation overestimated mortality in higher-risk groups and the revised equation is accurate for the most prevalent group with a NHFS 4–6 (maximum score 13).
Prediction of Generic Adverse Outcomes in Heterogeneous Patient Populations
The American Society of Anesthesiologists’ Physical Status Score
This is the most well-known and commonly used method of estimating population risk, most likely due to its simplicity and ease of use. The American Society of Anesthesiologists’ Physical Status Score (ASA-PS) was devised in 1941 as a statistical tool for retrospective analysis of hospital records and has been revised several times (Saklad, 1941). It looks at the functional status of patients before surgery and categorises them into five subgroups. There is an additional category for patients undergoing organ donation. There is a clear link between underlying physical fitness and outcome, and this has been demonstrated in a number of settings; therefore, the ASA-PS has plausibility as a basic measure of patient health. However, it also has a number of limitations. Its very simplicity means that it cannot provide an individualised risk assessment. In addition, some studies have reported moderate-to-poor inter-rater reliability, meaning that the subjective nature of grading patients may introduce bias (Mak, Campbell and Irwin, 2002). A number of studies have found that the ASA-PS lacks sensitivity and/or specificity for complications or death after surgery (Moonesinghe et al., 2013); this may be in part due to the lack of operative information within the score. Despite these limitations, however, the ASA-PS remains a widely used system which has face validity as a basic measure of functional status.
The Charlson Age Comorbidity Index
The Charlson Age Comorbidity Index (CACI) was originally developed in 1987 for the purposes of risk adjustment and prediction of 10-year mortality in medical patients (Charlson et al., 1987). It has since been validated for predicting mortality in various surgical cohorts. A number of premorbid medical conditions are each assigned a score of 1, 2, 3 or 6, depending on the risk of death associated with each one: these are then summed to provide a total score to predict mortality. A strength of the CACI is that the data required are all available preoperatively; however, there may be observer bias when assessing the co-morbidities. Furthermore, as with the ASA-PS, no information on the type or urgency of surgery is required: surgical magnitude is clearly important when considering patient risk, and thus risk-prediction tools which incorporate a measure of surgical severity are likely to be more accurate.
The Physiological and Operative Severity Score for Enumeration of Morbidity and Mortality
This was originally developed as a risk-adjustment system for surgical audit (Copeland et al., 1991). Several perioperative variables were identified using multivariable logistic regression to predict 30-day morbidity and mortality. The Physiological and Operative Severity Score for Enumeration of Morbidity and Mortality (POSSUM) incorporates 12 physiological status variables (available pre-operatively) such as patients’ clinical symptoms, examination findings, blood results and electrocardiogram assessments. In addition, six surgical variables such as presence of malignancy and repeat operative procedures within 30 days are included, giving a total of 18 items. The surgical variables can be accurately assessed only during or after the procedure, therefore making preoperative risk assessment more challenging. Some studies have found POSSUM to predict accurately the individual patient risk of morbidity and mortality (Brooks, Sutton and Sarin, 2005; Pratt et al., 2008), and others have found a considerable overestimation of mortality (Bollschweiler et al., 2005; Campillo-Soto et al., 2006; Tambyraja, Kumar and Nixon, 2005; Tekkis et al., 2003). A new version of the POSSUM model was created in Portsmouth (Prytherch et al., 1998): the same physiological and surgical variables were used, but with alternative risk equation for mortality; however, there is no updated model for prediction of morbidity. Systematic review has found that although P-POSSUM can both over- and under-predict mortality in different settings, it is the most accurate risk-prediction model in heterogeneous patient populations (Moonesinghe, 2013). Many modifications of POSSUM models have been developed for specific patient populations, including orthopaedics, vascular surgery, head and neck surgery and colorectal surgery.
The Surgical Outcome Risk Tool
A novel risk-stratification method is the Surgical Outcome Risk Tool (SORT) (Protopapa et al., 2014). Using six preoperative variables (ASA-PS, age, presence of malignancy and operative urgency, severity and speciality), SORT predicts 30-day mortality after inpatient surgery (non-cardiac, non-neurological). SORT was derived by analysing data from the UK’s National Confidential Enquiry into Patient Outcome and Death (NCEPOD) ‘Knowing the Risk’ study, which was conducted in 2011 on more than 15,000 patients. SORT demonstrated excellent discrimination (AUROC 0.91) on internal validation; however, external validations have yet to be published.
Which Risk-Stratification Tool Should I Use?
Each model or score has strengths and weaknesses; thus, making an assessment of risk using several different tools may provide an optimal means of determining perioperative risk. No risk stratification tool derived using current analytical techniques will be consistently ‘perfectly’ accurate – this would require the incorporation of a potentially limitless number of variables, which would include metrics of quality relating healthcare delivery in a particular institution, as we know that outcomes for patients may be affected by the skills and behaviours of clinical teams. Perhaps therefore, the most important principle is to use a combination of objective measures alongside clinical judgement; these results should then be discussed with the team and the patient, in order to facilitate informed, shared decision-making by all stakeholders, and this dialogue should be clearly documented (Findlay, 2011).
Importantly, there is a lack of ‘impact studies’ in the literature, and there may be benefit to further research to look at what influence these tools have on clinical decision-making and therefore patient outcomes. (Moonesinghe et al., 2013). There is also a gap in our understanding of the accuracy of these tools for predicting complications following surgery. Understanding the risk of requiring a prolonged critical care admission necessitating a tracheostomy, or long-term renal replacement therapy after discharge from hospital, may be more relevant and meaningful for patients than the percentage risk of being alive or dead at 30 days. Of prime importance is to understand whether patients and clinicians share an understanding of patients’ expectations of surgery. Some patients may be more interested in knowing their likely post-operative quality of life or how long it will take to get back to work, than the relatively low risk of death after surgery. Understanding these issues can only be facilitated through individualised discussion with patients, particularly as their decision-making is likely to be shaped by highly contextual issues – for example, their diagnosis, the range of treatment options and their personal circumstances, including financial status and whether they have dependents who rely on them for care. Shared decision-making is to be aspired to, particularly as non-surgical options may be more appropriate or appealing to both patients and clinicians.
Shared Decision-Making
‘Shared decision-making is the principal mechanism for ensuring that patients get “the care they need and no less, the care they want, and no more”’ (Coulter and Collins, 2011).
In 1998, at the Salzburg Global Seminar, Valerie Billingham coined the phrase ‘No decision about me, without me’. Nearly 20 years on, the healthcare profession is embracing change as the concept of patient-centred care begins its journey from theory to plausible reality. A central tenet to empowering and engaging patients in their healthcare is shared decision-making, described as the ‘pinnacle of patient-centred care’ (Barry and Edgman-Levitan, 2012).
Right Procedure, Right Patient
Shared decision-making (SDM) supports a model of care that places patients’ wishes, preferences and lifestyle goals at the centre of decision-making. It moves away from the traditional ‘doctor knows best’ model towards a consultation that involves two experts (Coulter and Collins, 2011). The healthcare professional remains the expert in diagnostics and valid therapeutic options, while the patient is regarded as the expert in individual attitude to risk, values and preferences. The clinician supports the patient through the deliberation process to arrive at a decision based on what matters to them; this process is a series of potentially increasingly complex decisions with the ultimate aim of matching the right procedure to the right patient (Bader, 2014).
Drivers for Shared Decision-Making
The President’s Commission for the Study of Ethical Problems in Medicine and Biomedical and Behavioral Research Report (1982) was the first formal mandate to implement shared decision-making into healthcare. It described informed consent as an active process of decision-making. The commission report brought into question the validity of consent that has not involved an opportunity for patients to review all feasible options and express their preferences. More recently, the UK White Paper ‘Equality and Excellence: Liberating the NHS’ (2010a) stated healthcare decisions should be made in partnership with patients, rather than led solely by clinicians. Subsequent consultation documents highlighted that patients should be given choice over valid options; however, simply offering choice does not equate to improved shared decision-making. (Department of Health, 2010b, 2012). This led to collaborations between the NHS and organisations such as the Health Foundation and the King’s Fund, investigating the improvement science necessary to make shared decision-making a feasible reality (Coulter and Collins, 2011; The Health Foundation, 2013b).
Alongside these reports, Wanless (2002) suggests that patients need to be fully engaged in their healthcare, both to limit healthcare episodes (‘demand’) and to allow effective allocation of healthcare resources (‘supply’). He suggests the resultant reduction in healthcare costs is significant. Underpinning these political drivers is the moral and ethical obligation of clinicians to treat patients as autonomous individuals.
Patients Want More Involvement
Within the UK, annual national inpatient surveys are performed by the Care Quality Commission to gauge patient experience within the NHS (2013). In 2013, only 56 per cent of patients reported they were involved as much as they wanted to be in decisions about their healthcare. Deficits in preoperative decision-making and informed consent have recently been highlighted, predominantly through lack of support and advice to inform a shared decision (Ankuda et al., 2014).
Does Shared Decision-Making Work?
The evidence for shared decision-making, at present, remains uncertain. The lack of a clear definition and variability in the measurements of outcomes has resulted in heterogeneity amongst studies, which are therefore challenging to compare. In addition, shared decision-making is a process involving a series of interventions, rather than a single healthcare episode, increasing the complexity of randomisation strategies and outcome measurement. Particularly, the evidence within perioperative care is scarce and not well validated. Emergent evidence reveals there may be short-term benefits such as improving patient confidence, self-determination and satisfaction. However, whether these are translated into tangible, long-term advantages is yet to be elucidated. (Shay and Lafata, 2015; The Health Foundation, 2012).
While shared decision-making is advocated on the ethical principle of patient autonomy, quantifying the effectiveness of the processes integral to its delivery would be beneficial to guide service provision (Shay and Lafata, 2015). For example, the Cochrane Collaboration reviewed interventions to improve healthcare professionals’ adoption of SDM in three categories: those targeting patients only (such as decision aids); those targeting professionals only (such as education and feedback); and those targeting both professionals and patients. They concluded that the latter category resulted in greater implementation of SDM in the clinical environment. However, this evidence was based on a small number of studies and the quality of evidence was deemed low (Legare et al., 2014). Knowledge of the magnitude of effect on patient-reported outcomes may support service improvement, education and training strategies.
Implementing Shared Decision-Making
Despite recurrent recommendations that SDM should be a key principle within patient-centred care, adoption has been slow, particularly within the perioperative setting. SDM requires a cultural change moving away from paternalistic healthcare models. Fundamentally, it will be possible only through professional and patient engagement, in addition to specific decision-making support. Identifying and overcoming barriers to this engagement is the challenge on the horizon, as described in what follows.
Patient Engagement
Empowering and engaging patients in perioperative consultations is imperative for high-quality surgical decision-making. A recent systematic review investigating patients’ perceptions of healthcare suggests attitudinal barriers: in particular, a perceived ‘power imbalance’ between patients and professionals, with patients not feeling empowered to make decisions about their healthcare, and, perhaps most worrying, some patients having the view that the quality of healthcare delivered is directly related to congruence with doctors’ proposed treatment plans (Joseph-Williams, Edwards and Elwyn, 2014). Such evidence suggests that currently, patients seem to not fulfil an autonomous role in healthcare decisions.
Studies from Australia and the UK and ongoing work in America support the use of three questions to support patients in healthcare consultations and to promote shared decision-making (PCORI, 2013; Shepherd et al., 2011; The Health Foundation, 2013a). Though subtle differences exist in the exact terminology of the questions in these three studies, the underlying principle is that patients should be encouraged to ask these three questions during discussions about treatment options.
1. What are my options?
2. What are the possible benefits and harms of those options?
3. How likely are the benefits and harms of each option to occur?
This simple intervention appears to improve the quality of information patients receive without increasing consultation length. Shephard and colleagues (2011) suggested this intervention might improve shared decision-making and overcome attitudinal barriers to patient engagement.
For professionals, successful patient engagement relies on a change in culture away from traditional consultation models. Given the fact that patients in the perioperative period may spend a significant time outside the hospital in the preparation or rehabilitation phases of their perioperative journey, one strategy might be to ‘flip healthcare’ (Bisognano and Schummers, 2014). The underlying principle is a shift away from delivering ‘healthcare’ to promoting ‘health’: i.e. instead of focussing on treating a ‘disease’, to emphasise promoting health-enhancing behaviours by understanding patients’ beliefs, preferences and lifestyle choices.
Professional Engagement
Supporting shared decision-making involves the timely delivery of accurate, evidence-based and objective information to a patient and providing subsequent support through deliberation. Professional barriers may impede this process; clinicians may feel they do this already or hold paternalistic values regarding healthcare consultations. Perceived time and financial constraints may deter professionals; however, this has been refuted and indeed more time may be spent supporting patients through decisional conflict (Coulter and Collins, 2011).
A significant barrier to the implementation of SDM within perioperative care is lack of education and training. Relational skills and risk-communication skills have been highlighted as areas for focus when developing core competencies in shared decision-making (Legare et al., 2013).
Supporting Deliberation
Once options have been considered, a structured process of deliberation should ensue, to support patients making a decision based on their individual values. ‘Unlocking the patient’ through decision support and discussion remains a challenging step for perioperative care professionals, which as discussed earlier, requires appropriate training in relational and risk-communication skills.
A model for this process has been proposed by Elwyn and colleagues (2012, 2013) entitled the ‘Talk Model’. In their review of shared decision-making models, Elwyn and colleagues (2013) identified that the key stages in supporting deliberation were ‘team’, ‘option’ and ‘decision’, talk elaborated further later in this chapter (see Figure 24.1).
The ‘option talk’ stage may be supported by patient decision aids, which have been shown to improve patients’ knowledge and reduce decisional conflict. However, whether decision aids result in improved patient involvement in decision-making remains unclear (Stacey et al., 2014).
Conclusion
Risk stratification using appropriate tools in combination with clinical judgement should enable optimised allocation of healthcare resources and enable patients to make informed decisions based on their understanding of the risks and benefits of the treatment options available. Adoption both of the routine use of risk-stratification tools and of shared decision-making as part of the perioperative pathway has been challenged by professional and patient-related barriers and by the lack of a robust evidence base. Nevertheless, a central role for these processes in perioperative care is emerging as surgical patients, procedures and therefore decision-making all become increasingly complex. Placing the patient at the centre of decision-making is imperative, and strategies to preserve patients’ autonomy support the ethical code of practice for perioperative professionals.
References






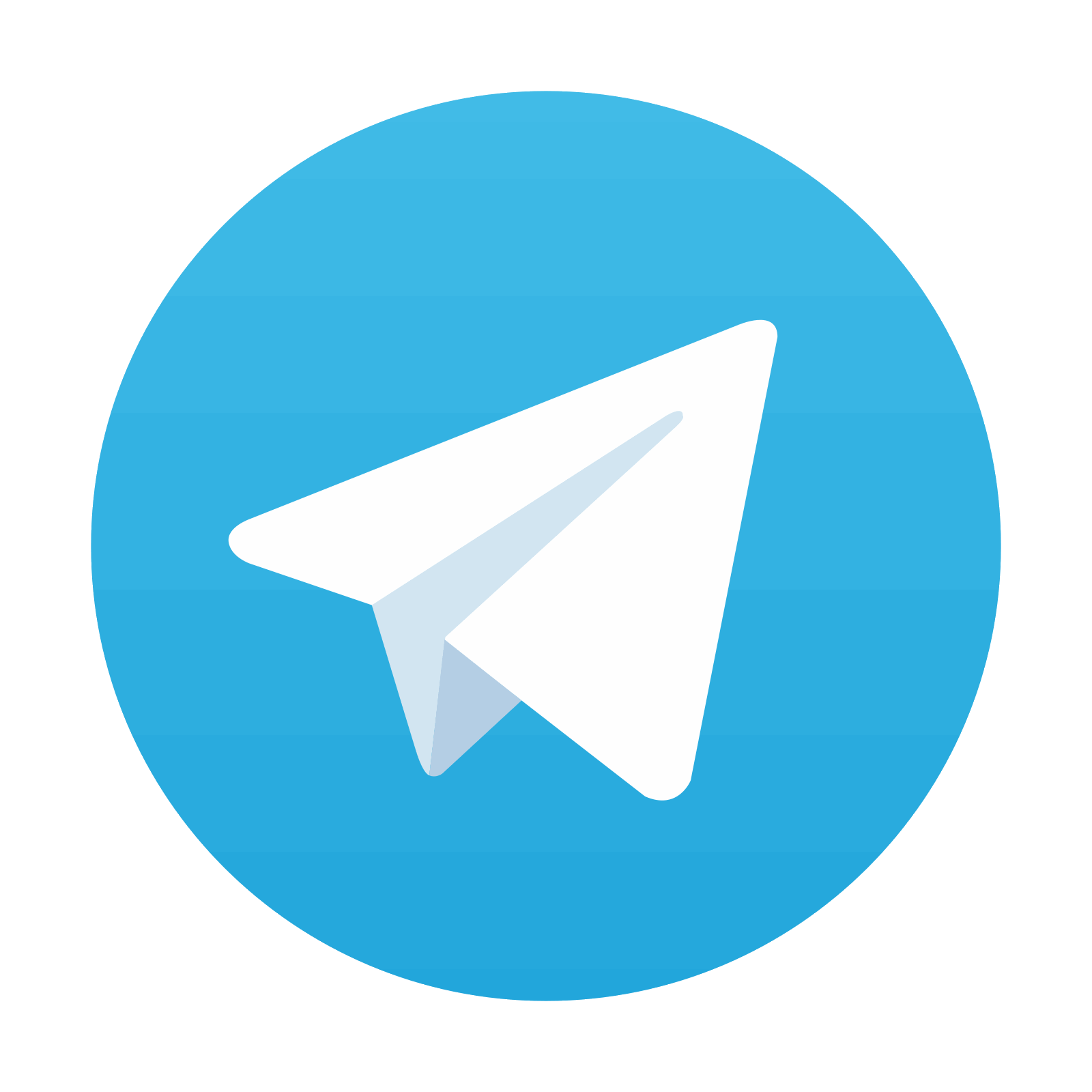
Stay updated, free articles. Join our Telegram channel

Full access? Get Clinical Tree
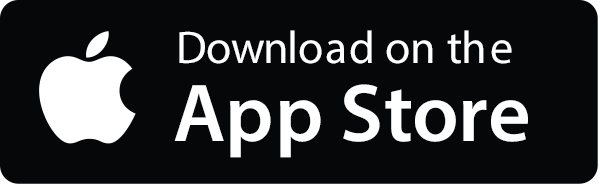

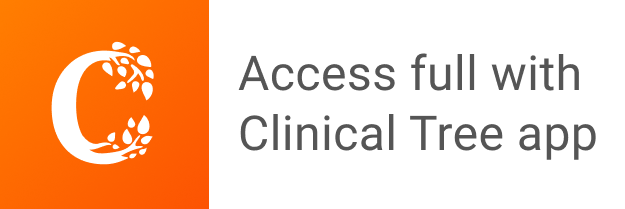