What is Needed to Establish Valid Predictors of WAD Recovery?
Linda J. Carroll
Fatemeh Vakilian

You are a clinician with a busy practice. You have in your office a 46-year-old woman who has neck pain after a recent car crash, in which the vehicle she was driving was struck from behind while she was waiting at a stop sign. The striking vehicle was going relatively slowly at the time of the crash, but could not stop in time due to icy conditions. Her current symptoms include neck pain and painful movement, with decreased range of motion and point tenderness, but no radicular symptoms. You know that your patient’s prior health was fairly good, although in the past few years, she has had recurrent problems with mild depression. She is now anxious about driving, and is fearful about how long the neck pain will last. What can you tell her? Which of these factors and circumstances predict a shorter recovery course, which a longer recovery course, and which are unrelated to the course of recovery?
The ability to predict a likely health outcome is crucially important in clinical care and in health policy development. Identifying those factors that aid in accurate prediction (i.e., valid predictors) is a critically important form of research. There is a plethora of studies reporting
predictors of recovery from whiplash-associated disorder (WAD); many of these studies contradict each other. The purpose of this chapter is to briefly outline some of the key questions to ask and aspects to look for when reading or conducting research whose goal is to identify valid predictors of WAD recovery outcomes.
predictors of recovery from whiplash-associated disorder (WAD); many of these studies contradict each other. The purpose of this chapter is to briefly outline some of the key questions to ask and aspects to look for when reading or conducting research whose goal is to identify valid predictors of WAD recovery outcomes.

Predictors of WAD recovery can be identified only through longitudinal research in which the potential predictor clearly precedes the health outcome of interest. In cross-sectional studies (where all data are ascertained at the same point in time), it is generally difficult to be certain about temporality; that is, where the “predictor” and “outcome” are measured at the same time, it often cannot be clearly established which came first. An example of this is pain coping style and pain severity. A cross-sectional study may identify an association between these two constructs. But that design cannot determine whether a particular pain coping style leads to greater pain severity or whether greater pain severity leads one to be more likely to adopt that particular pain coping style. However, this is not the only danger in making inferences about predictors using cross-sectional findings. Take the example of a cross-sectional survey that finds a higher proportion of women than men reporting persistent pain after a traffic-related WAD. Temporality is not in question here: We understand that being female predates the injury. Indeed, these findings might indicate poorer neck pain recovery in women than in men. However, those same findings could instead reflect that women have a higher risk than men of having neck pain after a traffic crash. If that is the case, even if women and men experience neck pain recovery at the same rate, there would be more women with persistent neck pain in that cross-sectional sample, simply because there were more women than men with traffic-related neck pain in the first place. This is an example of prevalence-incidence bias, which arises because prevalence is determined by both incidence (probability that a condition will occur) and duration of that condition.
The longitudinal design most commonly seen in the WAD literature on predictors of recovery is a cohort study. Ideally, potential participants are identified prospectively, enrolled in the study as soon as possible after experiencing a traffic injury, and are representative of the WAD population of interest. The study may compare recovery and predictors of recovery for different types of traffic injuries (e.g., WAD vs. ankle injuries) by following the cohort over time to assess similarities and differences in recovery of the two groups. Other times, there is no non-WAD comparison group, and all participants with WAD are followed over time to identify what pre- and postinjury factors predict recovery.

The most valid predictors come from a study that includes either the entire population of interest (the “target” population), that is, a population-based study that includes everyone with WAD in a particular jurisdiction or a representative sample of that population of interest. The target population—the population whom the researchers intend to draw conclusions about—should be clearly outlined in the study report and is defined by the inclusion/exclusion criteria. Representativeness in the sample is crucial because otherwise you may introduce selection bias.
Selection bias is a matter of internal validity of a study, and can occur when the actual sample is different from the target population [10]. This can happen when, for example, only 50% of those eligible for enrollment in a study (i.e., those meeting inclusion/exclusion criteria) agree to participate. Unless there is convincing evidence that respondents and nonrespondents are similar, it is likely that respondents are systematically different from those not participating (e.g., healthier or higher socioeconomic status), and this can lead to selection bias: the study findings may be incorrect. Selection bias can also be introduced through attrition. Dropping out from a study is rarely random, and a formerly representative sample may become nonrepresentative through, for example, selective attrition of those who are recovering
very well or, alternatively, those who are recovering poorly. Where selection bias is present, findings are invalid unless the bias is taken into account in the analysis or the interpretation of findings. At the very least, there should be some attempt to compare participants with nonparticipants, and to compare those who complete follow-up with those who do not.
very well or, alternatively, those who are recovering poorly. Where selection bias is present, findings are invalid unless the bias is taken into account in the analysis or the interpretation of findings. At the very least, there should be some attempt to compare participants with nonparticipants, and to compare those who complete follow-up with those who do not.
Generalizability is a matter of external validity [10]. Where there is low risk of selection bias (i.e., where the study sample is representative of the target population), study findings can be generalized to that target population as a whole. However, there can be no generalizability where internal validity is low. Note that a study that deliberately defines its target population narrowly but that obtains a representative sample of that narrow population does not suffer from selection bias. However, the narrow criteria that are used to define the target population will likely limit the researchers’ (and readers’) ability to generalize to a broader population; that is, such generalizations may not be valid.

In the current WAD literature on predictors of recovery, the most common sources of research participants are those making insurance claims for traffic injuries; those seen in ED after a collision; those enrolled/referred to intervention or other WAD studies, and “convenience” samples of persons recruited through newspapers, posters, etc. Each sampling frame has its strengths and weaknesses. For insurance sampling frames, some issues to consider when judging the likelihood of selection bias or poor generalizability to the broader WAD population are: Are there substantial barriers to seeking injury compensation? This will be highly jurisdiction dependent, since traffic insurance schemes vary widely. Can injured people obtain health care for WAD without making an insurance claim, i.e., does the claim’s sampling frame “miss” many of these persons with WAD? Where there are multiple options of insurance carriers, are there patterns (e.g., different socioeconomic characteristics) of why people seek insurance from one company
instead of another? To the extent that barriers to seeking insurance are minimal and most of those with WAD will make an insurance claim, where health care for traffic injuries is tied to making a claim and there is a single insurer for a particular jurisdiction, and where the study can ensure complete or almost complete inclusion of claimants in the study (or a representative sample), such studies reflect a representative sample of a target population of persons with traffic-related WAD. Thus, selection bias is minimized, and we can have more confidence that findings can be generalized to the larger WAD population.
instead of another? To the extent that barriers to seeking insurance are minimal and most of those with WAD will make an insurance claim, where health care for traffic injuries is tied to making a claim and there is a single insurer for a particular jurisdiction, and where the study can ensure complete or almost complete inclusion of claimants in the study (or a representative sample), such studies reflect a representative sample of a target population of persons with traffic-related WAD. Thus, selection bias is minimized, and we can have more confidence that findings can be generalized to the larger WAD population.
Another sampling frame often utilized in studies of WAD recovery is using consecutive (consenting) persons seen at ED. However, the adequacy of this as a sampling frame is also jurisdiction dependent since patterns of going to ED after a traffic collision vary. In some jurisdictions, an ED sampling frame may miss up to 50% of those with WAD [7]. To the extent that those who go to EDs after traffic collisions are systematically different from those who do not (e.g., may be more seriously injured, more anxious about injuries, have better access to health care, and so on), this may introduce selection bias if the target population of interest was the broader population of persons with WAD. In addition, it is often the case that one particular hospital ED is selected for study, which leads to questions about whether there are systematic differences in those who go to that ED as opposed to another ED (e.g., those with more severe injuries may go to a particular hospital, socioeconomic status may differ in those served by different hospitals, and so on). This, too, can introduce selection bias.
What about those sampling frames that include persons enrolled in intervention studies or referred to WAD research centers? The obvious advantage of this is that those agreeing to participate in intervention studies are generally more compliant, which will decrease nonparticipation and loss to follow-up and bias due to attrition. However, such participants may also be generally healthier and may be highly selected in other ways, so may not be representative of the larger WAD population to whom the researchers hope to generalize. In addition, when patients are referred to a study or a research center by their health care providers, we often have little clarity on how those health care providers made decisions about whom to refer and whom not to refer. Thus, not
only may there be substantial risk of selection bias, but that bias cannot be well addressed in the analysis or interpretation of findings. Convenience sampling of volunteers recruited from newspapers, posters, and so on can be fast and inexpensive, but such samples are highly unlikely to be representative. Convenience sampling has an important role in pilot studies or in studies whose goals are generating hypotheses, but unless the researchers can provide clear evidence that the sample actually represents a target population of interest, these types of studies are also at high risk of selection bias.
only may there be substantial risk of selection bias, but that bias cannot be well addressed in the analysis or interpretation of findings. Convenience sampling of volunteers recruited from newspapers, posters, and so on can be fast and inexpensive, but such samples are highly unlikely to be representative. Convenience sampling has an important role in pilot studies or in studies whose goals are generating hypotheses, but unless the researchers can provide clear evidence that the sample actually represents a target population of interest, these types of studies are also at high risk of selection bias.
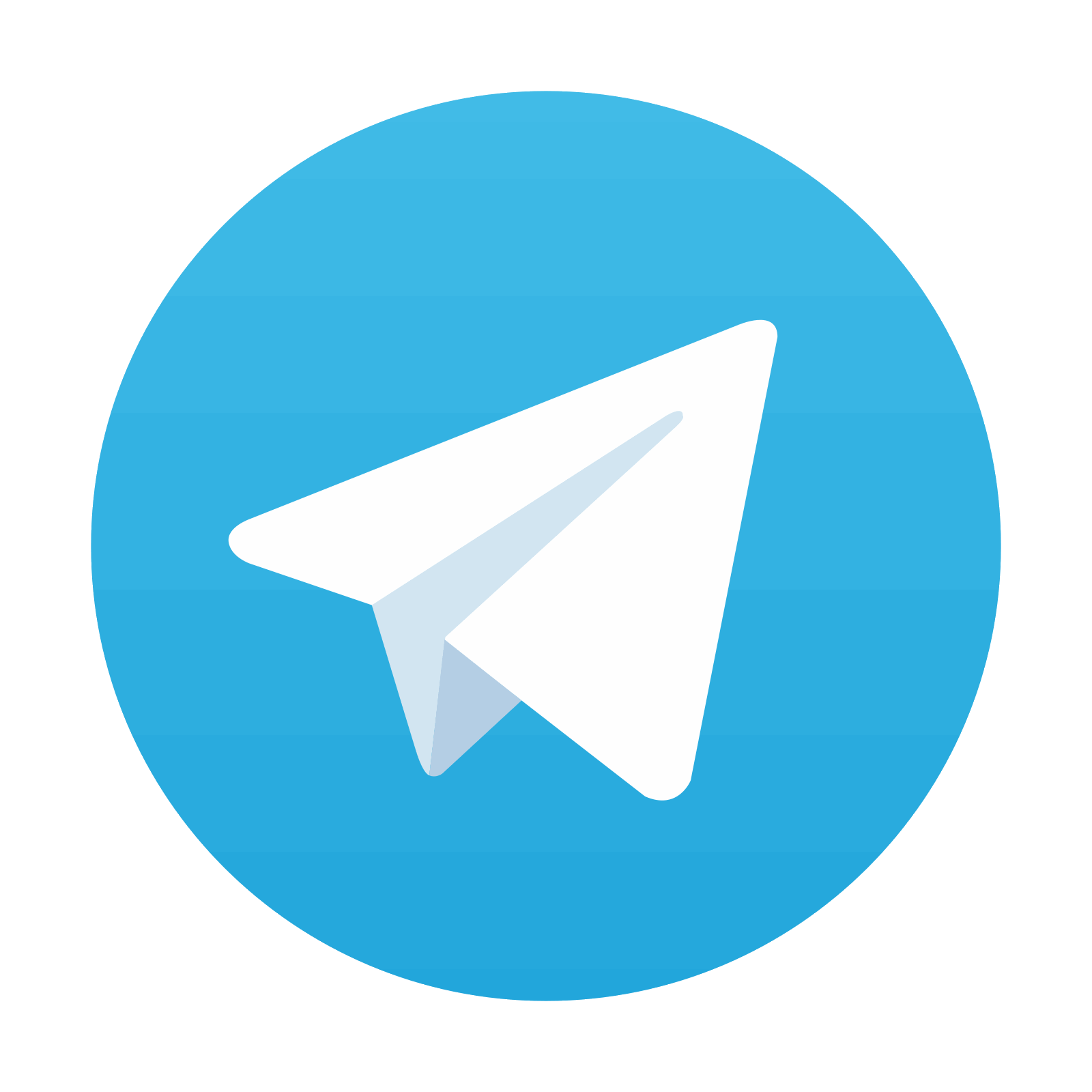
Stay updated, free articles. Join our Telegram channel

Full access? Get Clinical Tree
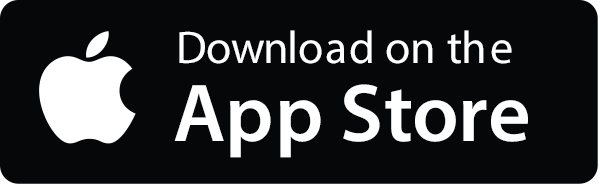
