There are many other study designs, most of which are variants of these basic ones, and many studies will include a combination of designs. As each design has its advantages and limitations, choice of design depends on the research question or hypothesis as well as feasibility and cost. Even the experimental design, considered to be the “gold standard,” is not always ethical, and results are only generalizable to patients like those who participated in the study. Moreover, no study is immune to bias, ie, any systematic error that threatens the validity of research findings.4 For instance, a study’s recruitment methods may enroll predominantly employed, upper middle class participants. These subjects tend to be more health conscious and perhaps get their health problems detected earlier when their disease is not so severe. Therefore, depending on the particular exposure and outcome being studied, the research findings may be biased by the inclusion of numerous subjects with these characteristics. There are endless ways in which a study can contain such systematic errors. Your past experience (or that of colleagues) may allow you to anticipate certain features of a study that would make it vulnerable to bias. Incorporate this information into your study design to avoid bias because, once a study is biased, its data cannot be “fixed” through statistical wizardry.
Basic Study Designs
1. Experimental (clinical trial) Exposure manipulated by the researcher 2. Cross-sectional Exposure and disease/outcome obtained at the same time or within a short period of time 3. Retrospective case-control Cases and controls selected and past exposures ascertained through interviews, medical records 4. Prospective cohort Exposed and unexposed individuals selected and followed to disease/outcome |
Studies in regional anesthesia will often follow the protocols of the experiment or clinical trial. The investigator will typically begin by randomly assigning patients to the “arms” of the experiment. For instance, in order to compare the effectiveness of a new anesthetic with an established standard, an investigator would randomly assign patients to receive one or the other of these medications. Similarly, patients might be randomly assigned to receive a novel combination of anesthetics to see whether the new cocktail is more effective or has fewer side effects than standard combinations of the medications.
Random assignment ensures that likelihood of assignment is equivalent for the treatment arms.
Because patients are not more likely to be assigned to one arm of the trial than another, the overall effect of random assignment is to reduce confounding by extraneous factors.
Random assignment ensures that likelihood of assignment is equivalent for the treatment arms. Because patients are not more likely to be assigned to one arm of the trial than another, the overall effect of random assignment is to reduce confounding by extraneous factors. However, random assignment can occasionally produce atypical groups. It is possible to assign high-BMI patients to one group and low- BMI patients to another. If such groups occur following random assignment, subjects should not be reassigned by the investigator. Fortunately, random assignment typically creates groups that are similar with respect to known (and even unrecognized) confounders.5
Random assignment is conducted in several ways. For simple randomization, one of the more popular methods is to use sealed envelopes. After consent to participate in the study has been given by the patient, the investigator or an assistant randomly picks an envelope that contains the group assignment for the patient. An equally valid method of random assignment involves the use of a printed table of random numbers (found in many statistics books) or computer-generated random numbers (an option in many statistical packages). A number in the table is pointed to with eyes closed. After this starting point is established by “blind stab,” the numbers are consecutively followed in a prespecified direction (top to bottom, left to right, or even diagonally). Numbers are taken in groups of 2s, 3s, or 4s, depending on whether < 99, 100- 999, or 1000+ patients are to be included in the study. If even numbers have been prespecified as the placebo group and odd numbers as the treatment group, any patient who consents when the number is even is assigned to the placebo group. If the next number is odd, the next patient who consents is assigned to the treatment group; however, if the next number is even, that patient becomes the second individual assigned to the placebo group. The process continues until all consenting patients are assigned to a group and may produce unequal numbers in the groups. Modifications to this scheme are incorporated depending on whether three or more groups are being studied. For example, numbers 01-33 could be assigned to the first group, 34-66 to the second group, and 67-99 to the third group.5
Other methods, such as blocked, stratified, weighted, or cluster randomization, may be useful5 and will be mentioned briefly. Blocked randomization isused when the investigator wishes to keep the number of subjects in each group very similar throughout the randomization process. Blocks are developed in which each treatment arm occurs the same number of times but in different orders. Because patients are then assigned in blocks, each treatment arm will have the same number of subjects. Stratified randomization is used when there is prior knowledge that it is important to balance a particular characteristic among groups. For example, to study the effects of two different epidurals on laboring women, the researcher may want to assign women by parity status to ensure similar numbers of primiparous and multiparous women. Within each of these strata, parturients are assigned to receive one of the two epidurals using the blocked method just described. Weighted randomization is used when there is a reason to have unequal numbers of subjects in the groups (perhaps more subjects are needed in a particular group in order to obtain a precise estimate of a key variable). This is easily accomplished by adapting the methods of simple randomization, eg, numbers 01-66 could be assigned to the first group and 67-99 to the second group. Finally, cluster randomization is used to allocate treatments to geographic areas rather than to individual subjects. Its relevance to regional anesthesia would include multicenter clinical trials.
Difference Between Descriptive & Inferential Statistics
There are two main subdivisions of statistics. Descriptive statistics portray features of the data that are of interest by organizing and summarizing the collection of observations.6 How this is done depends on the type of data collected. For example, types of peripheral nerve block (PNB) with respect to age of patient in years (a quantitative amount) will likely be presented as a mean and standard deviation of age for each of the blocks; however, frequency of blocks by peripheral vs neuraxial, by upper vs lower extremity surgery, or by gender of patient will likely be presented as numbers and percentages, as these qualitative data indicate class membership.
Inferential statistics uses information from study samples to test hypotheses about effects that are thought to be true in the population as a whole.6 Inferential statistics can be used to answer such questions as whether length of stay in the postanesthesia care unit (PACU) is significantly longer for patients who received femoral or sham block for anterior cruciate ligament repair.
Both areas of statistics are important. The distributional characteristics (ie, central tendency, spread, and shape) that constitute descriptive statistics are the very foundation of the more “glamorous” inferential statistics. Moreover, because descriptive statistics characterize key features of the data, it is an important tool for data cleaning. Are there missing values? If so, how much information is missing and why? Do the data make sense? Although an equal number of male and female patients may be expected to receive neuraxial anesthesia for lower extremity surgery, it would be an error to find a male receiving an epidural prior to delivery. Are there outliers; if so, are they true outliers or simply typographical errors? Did the patient weigh 702 lb or was weight written sloppily and the patient actually weighed 202 lb? By the end of this chapter, it should also be clear that the assumptions that must be met in order to apply inferential statistics properly are based on the distributional characteristics of descriptive statistics. Finally, statisticians will look at these characteristics to decide whether data need to be transformed before inferential statistics can be applied.
Measures of Central Tendency
The three most commonly used measures of central tendency are the mean, median, and mode.6 The mean is the balance point of the distribution and is responsive to the exact position of each score in the distribution. It is an indicator of skewness (ie, nonbell shapes) when used in conjunction with the median. However, because it is sensitive to each score in the distribution, the mean is more easily influenced by extreme scores than the median and the mode.
The median is the point that divides the upper and lower halves of a scale of ordered scores. Since it is not responsive to the exact value of the scores, it is less affected by extreme scores than the mean, and is often a better choice than the mean when describing central tendency for strongly skewed distributions. In fact, it is the only relatively stable measure for open-ended distributions.
The mode is simply the most frequently occurring score or the class interval that contains the largest number of subjects. The mode is easily “calculated” and is the only measure suitable for qualitative (class membership) data. Nonetheless, the mode is of little use beyond the descriptive level.
The three most commonly used measures of spread are the range, variance, and standard deviation.6 The range is simply the distance spanned by the highest and lowest scores in an ordered distribution. Thus it does not account for scores that fall between these two extremes. The range is easily “calculated” but is of little use beyond the descriptive level.
As noted by Fisher, variance is a key concept in statistics. Simply put, variance is the mean of squared deviations from the mean, Each score or observation in a sample is subtracted from the sample mean. These differences (deviations) are squared and summed, and the total is divided by the number of scores. Although vital to statistical inference, variance is of little use for descriptive purposes because people generally find it too difficult to think in terms of squares.
It is easier to think in terms of the standard deviation, which is merely the square root of the variance, The standard deviation is not only the most important measure to describe spread, but is of great use in inferential statistics. It is responsive to the exact position of each score in the distribution, but is quite resistant to sampling variation. As shown in the preceding formula, the standard deviation decreases as sample size increases.
Measures of Shape
Distributions come in many shapes, eg, rectangular, J-shaped, U-shaped. Shape can be described in terms of skewness and kurtosis.6 A distribution is positively skewed if its values appear to be pulled toward the higher end of the scale; conversely, a distribution is negatively skewed if its values appear to be pulled toward the lower end of the scale (as shown in 5 and 6, respectively, in Figure 83-1). The visual analogue scores (VAS) that are used to record patient ratings of pain are often positively skewed, indicating that most patients are comfortable and rate their pain at the low end of the distribution. Because the shape of the VAS distribution is not symmetrical and bell-shaped, it would be appropriate to categorize pain levels into discrete, reasonable ranges, eg, 0-3 none to mild pain, 4-7 moderate pain, and 8-10 excruciating pain. Nonparametric statistical procedures can be applied to test associations of interest that include these categories of pain.
Figure 83–1. A few common distributional shapes.
Kurtosis describes peakedness. Leptokurtic distributions have many values in the center and fewer in the tails of the distribution, giving them a peaked shape with “skinny” tails. In contrast, platykurtic distributions are less peaked so more values reside in the tails of the distribution, giving them a flatter shape with “fatter” tails. Finally, the mesokurtic distribution has more of a bell shape that approaches that of the normal curve.
The Normal Curve
There is only one standard normal curve. This gaussian curve, named for Carl Friedrich Gauss, a German mathematician (1777-1855), has particular distributional characteristics that are important for inferential statistics. It is symmetrical, bellshaped, and has a mean of 0 and a standard deviation of 1. As shown in Figure 83-2, its tails do not touch the horizontal axis because the distribution continues to infinity. Nonetheless, approximately 68% of the scores fall within ± 1 standard deviation of the mean, and roughly 95% of the scores fall within ± 2 standard deviations of the mean; hence, few drawings of the normal curve need to continue beyond ± 3 standard deviations. Table 83-4 gives areas under the standard normal curve for values of z. In particular, note that z scores of ± 1.96 correspond to 2.5% in each tail of the curve.
The convenient distributional characteristics of the gaussian curve allow us to calculate standard, or z scores, ie, . In effect, this simple calculation of score minus mean divided by standard deviation gives individual scores their addresses on the normal curve. As scores have been standardized to the same curve, we can compare performance on different measures. For example, a score of 95 on an English exam and a score of 75 on a math exam does not necessarily mean that the student performed better on the English than on the math exam. If the mean for the English exam was 100 with a standard deviation of 7.5, the student’s score of 95 is two-thirds of a standard deviation below the mean. If the mean for the math exam was 60 with a standard deviation of 10, the student’s score of 75 is 1.5 standard deviations above the mean. Thus this student actually performed better on the math exam than on the English exam.
Figure 83–2. The gaussian (standard normal) curve.
Sampling Distribution of the Mean
Recall that it is rarely possible to study an entire population, so we must look at a sample taken from the population and draw conclusions based on our particular sample. But in order to do this, we must first look at the notion of the sampling distribution of the mean. Instead of individual scores, the sampling distribution of the mean consists of the means of all samples of a specified size taken from the entire population.2 We then determine our particular sample’s “address” in this distribution.
The hypothetical parent population at the top of Figure 83-3 consists of four scores. The means of every possible sample of size 2 are calculated and distributed into the sampling distribution of the mean at the bottom of the illustration. From this sampling distribution we are able to determine the address of any sample mean (here, of size 2 ) that we might obtain. Thus a sample mean of 4.5 has an address approximately three-quarters of a standard deviation above the mean on this sampling distribution.
Central Limit Theorem
In order for the concept of sampling distribution of the mean to be useful, we must also consider the third distributional characteristic, shape. Here, we are fortunate to have an ally known as the central limit theorem. It states that the shape of the sampling distribution of the mean approximates a normal curve if sample size is sufficiently large. So, the question becomes “what is sufficiently large?” This depends on the shape of the parent population. If the parent population is normal, any sample size is sufficiently large. Depending on the extent of abnormality in the parent population, sample sizes between 25 and 100 are typically large enough for the sampling distribution of the mean to obtain the symmetrical bell shape with central peak and tapered flanks on either side.
Null Hypothesis
The null hypothesis (denoted as H0) is a statement that there is no effect (eg, there is no difference in length of PACU stay between patients given regional anesthesia and patients given general anesthesia). Ho is presumed true until proven false. We must decide whether to reject or retain H0 based on samples of patients given regional or general anesthesia. Our decision depends on the address of the test statistic on its sampling distribution (Figure 83-4). A test statistic whose address is in the extreme tails of the distribution is likely to have arisen from a distribution dissimilar to that described by the null hypothesis, and the conclusion would be to reject H0. Conversely, a test statistic whose address is in the middle of the distribution is likely to have arisen from a parent population similar to that described by the null hypothesis, and the conclusion would be to retain H0. P- values indicate how extreme a test statistic is and are used as a guide to rejecting or retaining the null hypothesis.
Table 83–4.
Proportions (of Area) Under Standard Normal Curve for Values of z
Data from Witte RS, Witte JS: Appendix D: Table A—Proportions under normal curve for values ofz. In Witte RS, Witte JS: Statistics, 7th ed. John Wiley & Sons, 2003.
Figure 83–3. A sampling distribution of the mean.
Figure 83–4. Hypothesized sampling distribution of z.
Alternative Hypothesis
The null hypothesis always makes a claim about one specific value. For example, if we test that there is no difference in time to onset of two anesthetics, we are testing that the difference in onset time between the two anesthetics is literally 0.0000. The alternative, or research, hypothesis (denoted as Hi or sometimes as Ha) generally complements the null hypothesis and makes a claim about a range of values. Since it is impossible to test infinitesimally every value in a range, we always test the null hypothesis despite our very real research interest in the alternative hypothesis.
The alternative hypothesis comes in three forms: twosided, one-sided with lower tail critical, one-sided with upper tail critical. If we state that the effect of a new anesthetic on intraoperative blood pressure is different from that of the more established anesthetic, we have not indicated directionality (the new anesthetic may either raise or lower intraoperative blood pressure), so the alternative hypothesis is two-sided. If we state that the effect of a new anesthetic is to lower or to raise intraoperative blood pressure, the alternative hypothesis is one-sided with lower tail critical or upper tail critical, respectively. Choice of the one-sided alternative hypothesis should be stated when your sole concern is about deviations in one direction. However, for most studies, the two-sided alternative hypothesis should be stated.
The null hypothesis and one of these alternative hypotheses is stated for each research aim of a study. These hypotheses are not questions and should not be written as such. They should not be influenced by preliminary peeks at the data, and ideally should be stated before data are even collected. Once statements about H0 and Hi are made, they cannot be changed to accommodate the empirical findings of the study.
Type I & Type II Errors
The type I and type II errors are probabilities:
a“Missing the boat,” or failing to convict a guilty person
b“Power” or rejecting Hq when Hq is false (1 —ß)
c“False alarm” or convicting an innocent person
The type I error is the probability of rejecting H0 when H0 is, in fact, true (there actually is no effect). The type II error is the probability of accepting H0 when H0 is, in fact, false (there actually is an effect). At issue is not whether one error is worse to commit than the other. Neither error can be avoided entirely, so investigators must decide how much error they can tolerate. Is it more important to avoid a type I error (perhaps stating that a particular anesthetic can be used at a lower dose because it provides excellent pain relief when it really does not, which might result in undue pain for the patient) or is it more important to avoid a type II error (perhaps stating that a particular anesthetic does not cause bradycardia when it really does, which might endanger the patient during surgery)?
After due consideration, the investigator sets the tolerable type I error by specifying oc. Typically, this is .05, that is, the investigator is willing to take a 5% chance of committing the type I error. Things are a bit more complex with the type II error inasmuch as a number of factors can affect the size of the type II error. The factor over which the investigator has most control is sample size. Studying a larger sample lowers the probability of committing the type II error (Table 83-5).
Larsen WJ: Human Embryology, 2nd ed. Churchill Livingstone, 1997.
Factors Affecting Type II Error (ß) |
|
Sample size: Larger sample size lowers ß. |
|
Discrepancy between what is hypothesized and what is true: Larger discrepancy lowers ß. |
|
Standard deviation of variable: Smaller Σ lowers β. |
|
Relation between samples: Dependent samples can lower β. |
|
Level of significance: Larger oc lowers β. |
|
Choice of Hi: β is smaller for a one-sided test than for a two-sided test. |
Data from Minium EW: Statistical Reasoning in Psychology and Education, 2nd ed. John Wiley & Sons, 1978.
Figure 83–5. Sample size estimation.
Principle of Sample Size Estimation
Figure 83-5 shows the underlying sampling distribution of the population means under both the null and alternative hypotheses. For simplicity, only one curve (that for a one-sided lower tail critical test) is shown for the alternative hypothesis. If the researcher has decided to set the type I error at, say, .05, the critical value (“address”), denoted in the figure as is —1.64 for the z statistic (see Table 83-4). Therefore, test statistics with addresses to the right of this critical value would result in retention of the null hypothesis, and addresses to the left of the critical value would result in rejection of the null hypothesis.
Power (1 —β) is determined by the sampling distribution of the alternative hypothesis. As previously mentioned, increasing sample size decreases variability. Thus, if the usual control over β is exercised (ie, increasing sample size), both curves pull apart, resulting in less overlap of their tails. The probability of committing a type II error (β) is lessened as the two curves pull apart. This is why it is important to obtain a reasonable estimate of the number of subjects needed in research studies. With too few subjects, the overlap of the curves may be so considerable that it may be impossible to detect a difference, even a sizeable one. With too many subjects, the overlap of the curves may be so slight that a small, clinically unimportant difference can meet or exceed the criterion for statistical significance. Factors that affect sample size are summarized in Table 83-6.
Degrees of Freedom
As the name implies, degrees of freedom (df) are the number of values, within a given set of values, that are free to vary. For example, in order to estimate the population variance (Σ2) from the standard deviation (S) of a sample of values, one of the scores cannot vary. Why? It is a mathematical truth that differences from a mean must sum to zero, that is, . So, given three scores (Xl, X2, X3 in the sample, the first deviation
might be +4, and the second deviation
might be -5. The third deviation
must therefore be +1. That is, the score X3 is not free to vary because it must take a value that will produce a deviation of + 1.
In general, a degree of freedom is used whenever a parameter is estimated, such as the population variance that was illustrated. Similarly, a degree of freedom is used to estimate each treatment effect being studied in a multifactorial analysis of variance procedure and to estimate each coefficient in a multiple regression model. This is the primary reason that statisticians are concerned about having sufficiently large sample sizes in research studies.
Factors Affecting Sample Size (n)
n increases as variance ( Σ2 ) increases. |
|
n increases as the significance level is made smaller (ie, as a decreases). |
|
n increases as the required power is made larger (ie, as 1 —β increases). |
|
n decreases with larger absolute value of the distance between the null and alternative means (that is, as |μ0 —Pj| increases). |
|
n is larger for two-sided than for one-sided tests. |
Data from Minium EW: Statistical Reasoning in Psychology and Education, 2nd ed. John Wiley & Sons, 1978,
Guidelines for Judging the Significance of a p-Value
If .01 < p < .05, then the results are significant. |
|
If .001 < p < .01, then the results are highly significant. |
|
If p < .001, then the results are very highly significant. |
|
If p > .05, then the results are considered not significant (sometimes denoted by NS). |
|
However, if .05 < P < .10, then a trend toward statistical significance is sometimes noted. |
Data from RosnerB: Fundamental of Biostatistics, 5th ed. Duxbury 2000.
p-Value
The p-value can be viewed from two very similar perspectives. Thus far, no formal statistical testing has been conducted; however, the concept of critical values has been described: A test statistic is computed and compared with an “address” determined by the tolerable type I error. Thus the p-value is the level of significance at which the test statistic is on the borderline between the retention and rejection regions of H0. This is frequently .05 (or .025 in both tails for the two-sided test). Although statisticians are not certain how Fisher chose the critical p-value of .05, he may have felt that this is the extent of type I error that most researchers could comfortably accept (Table 83?7).
The p-value can also be viewed as the probability of obtaining a test statistic as extreme as or more extreme than the actual test statistic obtained given that H0 is true. Most statistical software programs automatically provide p-values. The computer printout will contain notations attached to test statistics, such as p = .036, indicating that the probability of obtaining a test statistic at least as extreme as the one obtained is only 3.6%. And, as this is rather rare, Ho would be rejected. Note that the p-value of .036 should not be interpreted as a 96.4% chance that Ho is wrong. Put another way, p- values are calculated on the assumption that H0 is true, but they do not tell us whether that assumption is correct.7
Confidence Intervals
Confidence intervals expand our view beyond p-values. Figure 83-6 illustrates that for samples of a given size, intervals can be constructed that may or may not include the population parameter under scrutiny (here, the population mean, μ). Over the collection of all 95% confidence intervals that could be constructed from repeated random samples of a given sample size, 95% of them will contain μ. In reality, you would be unable to construct this figure because you will study only a single sample. Consequently, you would not know for certain whether the confidence interval from your particular sample actually does include the population value. However, you would know that, in the long run, 95% of the intervals from studies such as yours will contain the population parameter. Thus you will have an estimate of the population parameter with reasonable certainty.
Confidence intervals have several advantages. The p- value approach makes a statement about a derived statistic (usually the family of F, r, or chi-square statistics). This approach merely produces a yes or no answer about whether to retain Ho and could lead to confusion between statistical significance and clinical importance. Confidence intervals, on the other hand, make a statement about the actual parameter of interest. That is, an estimate of the likely value of the population parameter is produced along with an idea of the precision with which this estimate was made. If the confidence interval is narrow, the estimate was based on a sizeable number of subjects and precision is good; conversely, if the confidence interval is wide, the estimate was based on fewer subjects and precision is not as good.
Parametric & Nonparametric Statistical Tests & When to Use Them
A parameter is a characteristic of a population. One of the parameters that we are often interested in is the population mean, μ. Parameters are usually denoted by Greek letters, whereas characteristics of samples are usually denoted by Roman letters (eg, a sample mean is denoted as X). Parametric tests evaluate specific differences among population parameters, such as population means or variances, but nonparametric tests evaluate hypotheses for entire population distributions.
Parametric tests are used with quantitative data and require assumptions about the precise form of the population distribution, eg, normality and equal variance (also known as homogeneity of variance). Nonparametric tests are used with quantitative, ranked, or qualitative data and require no assumptions about the precise form of the population distribution. These “distribution-free” tests can be used with quantitative data that have nonnormal distributions and when variances of the groups are not equal, but they must be used with ranked or qualitative data.
When it is believed that the normality and homogeneity assumptions are met, parametric tests are more powerful than nonparametric tests. However, when sample size is small (say, less than 10), there is a very good possibility that the assumption of normality has been violated; furthermore, when sample sizes are small and unequal, there is a very good possibility that the assumption of equal variance has been violated. Under these circumstances, the nonparametric tests should probably be used. We have already discussed an example of nonnormality with the VAS scores. It was suggested that perhaps the scores should be categorized into levels of pain and submitted to nonparametric tests (as will be discussed in the section on Nonparametric Tests).
Figure 83–6. Several 95% confidence intervals arising from the sampling distribution of the mean.
Data Transformations
Raw data may need to be transformed in order to meet the assumptions of the parametric statistical tests. As described throughout this section, data must come from a population with normally distributed values. Thus data that are inconsistent with this assumption should be transformed prior to conducting a statistical test. It is convenient that transforming to normality can reduce the influence of outliers (atypical values) on the analysis, thus a nonparametric test may become unnecessary. Another assumption of many parametric tests is that different groups have the same variance; hence, violations to this assumption may require data transformation. A third assumption applies to regression (and will be covered in Parametric Tests). It states that the model must be a linear combination of variables; hence, transformation must be considered if regression coefficients or raw data suggest a violation. It is indeed fortunate that the same transformation often helps to meet the first two assumptions (and sometimes even the third), rather than dealing with one assumption at the expense of the other two.
The only transformation that will be described here is the log transformation as it is by far the most frequently used. Simply, the logarithm of each raw value is computed and used in the inferential statistical analysis. However, results of the analysis are presented in the original scale of measurement. Other transformations require that the square root, reciprocal, square, or even the arcsine of the raw data are obtained prior to analysis. Statistical advice is necessary when using transformations, as certain conditions apply. For instance, a statistician may note that variance increases as the mean of the groups increases (producing a rather funnel-shaped scatterplot) and is likely to suggest the log transformation. However, the statistician will also know that this transformation can be used only if the outcome variable takes no negative values.
PARAMETRIC TESTS
Our foray into inferential statistics begins with the z test for a single population mean, the most fundamental of the inferential tests. This test is accurate only when the population is normally distributed (or the sample size is large enough to satisfy the central limit theorem), and the population standard deviation, Σ, is known. For instance, we wish to know whether the mean composite MCAT scores for college students in New York equals the 2005 national average of 24.5. Here, the population standard deviation is known (± 6.5).
Recall that and provides a scores address on the normal curve. It follows that the z score for a sample mean is merely an extension that provides the sample mean s address on the normal curve. So, instead of frequencies of scores, the distribution now consists of frequencies of all sample means
where
If the mean composite MCAT score from a sample of 30 New York students is 27,
, which is significant at p = .018. Therefore, we reject H0 that New York students are no different from the national average (in fact, they score higher on the composite MCAT than the national average).
Unfortunately, in regional anesthesia and in other areas of medicine, we rarely know Σ, so the z statistic is not frequently used. Rather, it was included here because it is instructive to note the difference between the z and t statistics. Unlike the z statistic, which has a known population standard deviation and thus has a constant denominator, the t statistic has a variable denominator that depends on the size of the sample.
This is because there is a whole family of t curves, one curve for each sample size. As shown in Figure 83-7, although each curve is bell-shaped, the area of the curve in the tails increases as sample size decreases. Thus the z score address on a curve with few degrees of freedom must be higher (in the upper tail) and lower (in the lower tail) than the address on a curve with many degrees of freedom. This is to accommodate the former’s thicker tails. For the curves shown in the figure, the z scores are ± 2.776 and ± 2.228 with 4 and 10 df, respectively, for 2.5% in each tail.
Figure 83–7.A couple of f distributions.
The difference between the t and standard normal distributions is greatest for small sample size (usually <30). As sample size increases, the curves converge to that of the standard normal curve because the sample variance (s2) becomes less variable, and s2 is better able to approximate the population variance (Σ2). In fact, tables of critical values for the t statistic rarely include degrees of freedom beyond 120, as critical values beyond 120 are virtually the same as those in the table for the z statistic (in which degrees of freedom are infinite).
It should also be noted that the sample variance, s2, is now being denoted with a lower case s, as opposed to the upper case S used in the descriptive statistics section of this chapter. Deviations of scores from their sample mean, X, tend to be smaller than deviations from other values, such as the population mean, μ. Thus for inferential statistics, a better estimate of the population variance, Σ2, is necessary. In order to offset a sum of squared deviations that is too small, the number of scores in the denominator of s2 is reduced by 1. The sample variance, s2, calculated with η — 1 in the denominator, is denoted by the lower case 5 in order to distinguish it from the sample variance, S2, used in descriptive statistics (which has n in the denominator).
For our example of the t test for a single sample, we wish to know whether the heart rate of patients undergoing interscalene brachial plexus block differs from the adult norm of 72. Heart rate is recorded on 18 patients (mean 75 bpm ±8.8). We calculate , which is not significant at p > .05. Thus heart rate in our sample of patients undergoing interscalene brachial plexus block does not differ from the adult norm of 72 bpm. Note that the test is with 17 df because a degree of freedom was lost estimating sx-.
Student’s f Test
The Student’s t test compares two independent groups with respect to some continuous variable. The test was named for William S. Gosset, a British chemist and statistician (18761937) who worked out the mathematics for the family of t distributions and wrote under the penname of Student. The groups must be independent, so a patient cannot contribute more than one value to a group nor can a patient contribute values to both groups. The formula to test is basically the same as that for the one sample case, in which the sample mean has been replaced by the mean difference, , the population mean by the population mean difference, (μ, —μ2), and the sample standard deviation by the standard error of the mean difference,
. It should be noted that (μι —μ2) is regarded as 0 whenever the null hypothesis posits no difference between the groups. It should also be noted that the standard error is essentially a standard deviation; the term error is often used to describe variability of computed measures, such as a sample mean.
For our example, we wish to know whether postoperative heart rate measured in the recovery room differs between patients who receive general and those who receive spinal anesthesia. Postoperative heart rate on 10 patients who received general anesthesia (87.7 ± 8.8) is compared with that of 10 patients who received spinal anesthesia (72.7 ± 7.1), idf = is = 4.2, p = .001. Thus postoperative heart rate differs by anesthetic technique; it is higher in patients who receive general anesthesia. Note that 2 df were lost estimating Si and s2.
Since
Dependent (Paired) f Test
Groups are not independent if subjects are matched on some variable or the same subjects are tested before and then after some intervention. For our example, we wish to know whether heart rate differs between patients who received epidural anesthesia at the T1 level before and after a bolus of 15 mL of 2% lidocaine. Heart rate prior to bolus (78.7 ± 7.4) is compared with heart rate subsequent to bolus administration (69.6 ± 6.7) in the same patients, idf =9 = —10.4, p < .001. Heart rate before bolus differs from heart rate after bolus; it is lower following bolus administration. Note the similarity with the Student’s t formula: The sample mean difference, (Xi —X2), has been replaced by the mean difference between scores, Ď, the population mean difference, (μ1 —μ2), has been replaced by the population difference, μ0, and the standard error of the mean difference, Sx.-x,, by ??. It should also be noted that μ0 is again regarded as 0 because the null hypothesis posits no difference in heart rate before and after boluses of lidocaine.
One-Way Analysis of Variance
One-way analysis of variance (ANOVA) extends the Student’s itest of two population means to three (or more) population means. Whereas the difference in postoperative heart rate between patients randomly assigned to receive one of two different anesthetic techniques might be tested with the Student’s t test, one-way ANOVA could be used to test differences in postoperative heart rate between patients randomly assigned to receive one of three (or more) different anesthetic techniques. The assumptions in ANOVA are the same as those for Student’s t, ie, all underlying populations are normally distributed with equal variance.
Deviations reflecting random error and the effect of treatment are calculated and compared as a ratio of two variances, known as the F ratio in honor of Fisher. When the null hypothesis is true and there is no treatment effect, both the numerator and denominator of the ratio will merely reflect deviations caused by random error. As these will tend to be similar, the F ratio will vary about a value of 1.0. Conversely, when the null hypothesis is false and there is a treatment effect, the groups will differ from each other. The deviations that are due to treatment enlarge the numerator sums of squares, and the F ratio will become greater than 1.0. In order to determine whether the F ratio has become large enough to conclude that the groups actually do differ beyond chance fluctuation, its address is located on the F distribution (a sampling distribution of variance ratios). If its address falls beyond the critical value into the improbable region, the null hypothesis of no effect is rejected. That is, the anesthetic techniques do differ in how they affect postoperative heart rate. Conversely, if the F ratio’s address falls in the probable region, the null hypothesis is retained (ie, the anesthetic techniques do not differ in how they affect postoperative heart rate). Like the t distributions, there is a whole family of F distributions, and the critical values are looked up by the appropriate number of degrees of freedom in the numerator and denominator or are provided by the statistical program.
For our example, we wish to know whether postoperative heart rate differs among patients who received general, spinal, or PNB for knee arthroplasty surgery. Postoperative heart rate is studied in patients who are randomly assigned to receive one of the three anesthetic techniques (20 patients per group). We find from the ANOVA that postoperative heart rate differs significantly by anesthetic technique (the critical value for F with 2 and 57 df is approximately 5.0 for p = .01 and is exceeded by the obtained F ratio of 6.5). Subsequent pairwise comparisons reveal that postoperative heart rate is lower in patients given spinal anesthesia (72 bpm) than in those given general anesthesia (88 bpm) but does not differ from that of patients given PNB (78 bpm).
ANOVA Table
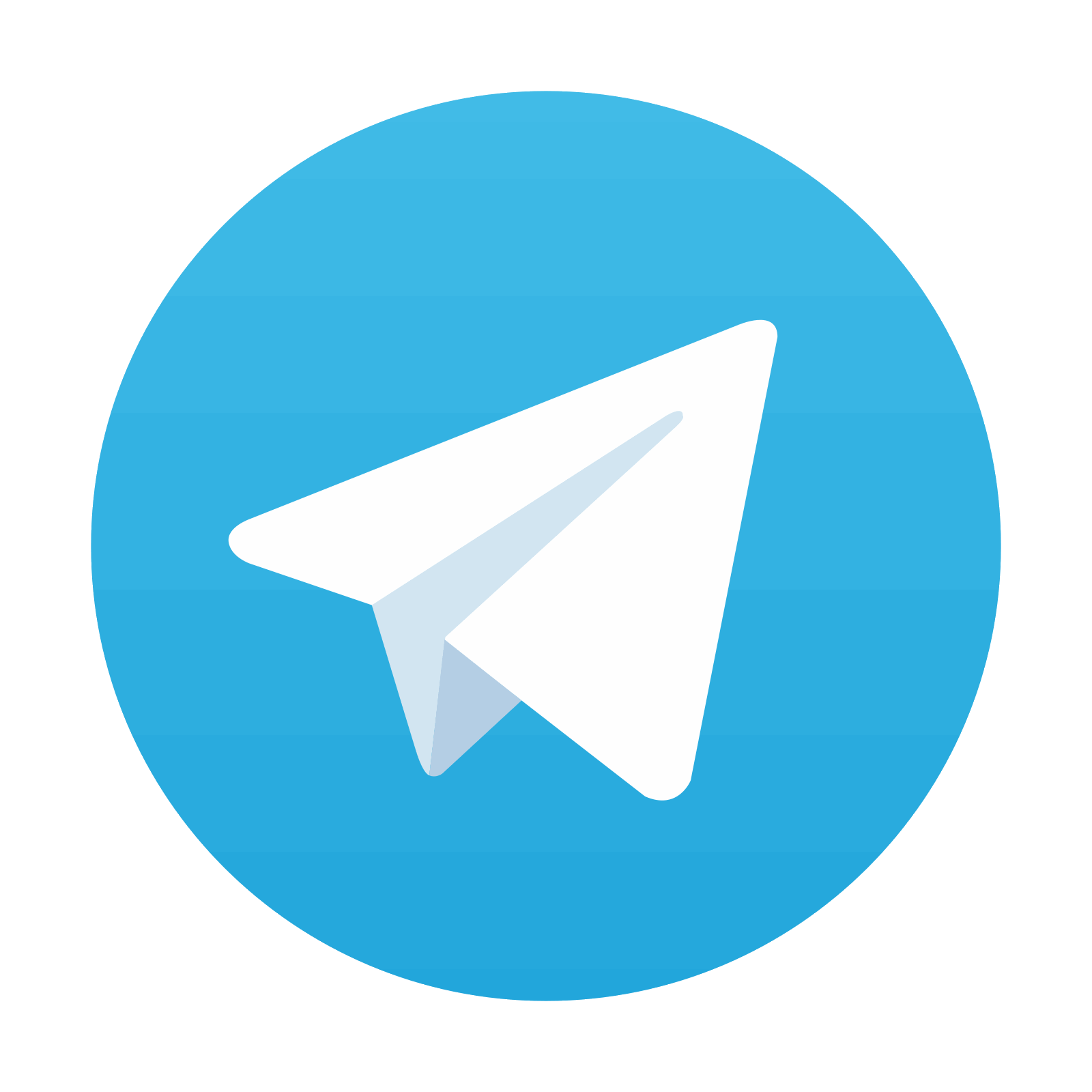
Stay updated, free articles. Join our Telegram channel

Full access? Get Clinical Tree
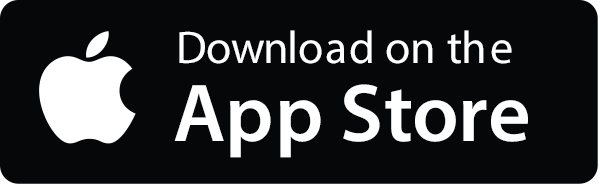
