Pearls
- •
Physiologic instability is a key factor in the prediction of short-term outcomes in critically ill patients.
- •
Prediction tools are central to controlling for severity of illness in studies and unit-based quality assessments for both internal and external benchmarking.
- •
Regression analysis is typically the central technique for constructing outcomes prediction tools.
- •
Assessment of the validity of prediction tools centers on two statistical measures: discrimination and calibration.
- •
Although mortality has historically been the outcome of interest, prediction tools for morbidity have recently been developed, as well as for clinical outcomes such as length of stay and reintubation.
Ongoing efforts to provide high-quality, error-free care require both the evaluation of complex systems and an assessment of the quality of care. Outcomes research is an important aspect of both requirements. Scoring systems add objectivity to these assessments, especially in critical care units. Controlling for population differences, such as differences in severity of illness, enables both the inclusion of different healthcare systems in a single investigative effort and contrasting individual healthcare systems in quality of care assessments. Measuring mortality adjusted for physiologic status and other case mix factors has been the core methodology of adult, pediatric, and neonatal intensive care assessments for decades for both internal and external benchmarking.
However, mortality rates in most pediatric intensive care units (PICUs) have decreased since these methods were developed. Medical therapies increasingly focus on reducing morbidity in survivors. Unfortunately, most quantitative outcome assessment methods continue to focus on the dichotomous outcomes of survival and death. Recently, there has been a new appreciation of the importance of other patient outcomes, such as discharge functional status, and better understanding of their determinants. The future will most likely see a diversity of patient outcomes of interest, methods to associate risk factors with these outcomes, and use of these risk factors for outcome prediction.
Historical perspective
The “modern” history of intensive care unit (ICU) scoring systems started with the Clinical Classification Scoring (CCS) system and the Therapeutic Intervention Scoring System (TISS). Although simple, the CSS system established the basis of severity of illness as a concept related to both physiologic instability and amount and intensity of therapy, ranging from routine inpatient care to the need for frequent physician and nursing assessments and/or therapeutic interventions. The TISS was based on the concept that sicker patients receive more therapy, such as mechanical ventilation or vasoactive agent infusions; thus, the number and sophistication of therapies serves as a proxy for severity of illness. Initially, 76 therapies and monitoring techniques were graded from 1 to 4 on the basis of complexity, skill, and cost. The TISS score still exists today, although the number of therapies has been reduced and objectivity has been added to the score.
The concepts of sequential or multiple organ system failures (MOSFs) were also important in the development of the concepts of severity of illness. Mortality rates increased as the number of failed organ systems increased. The MOSF syndrome was initially described in children in 1986. Although there have been numerous minor adjustments to the definition of an organ system failure, it continues to be based on the initial concepts of failure defined as extreme physiologic dysfunction or use of a therapy preventing that dysfunction.
Organ system failures have also been proposed as an outcome measure; since death is uncommon in PICUs, it is appealing to postulate that the number of organ failures or the temporal resolution of these organ failures could be a practical outcome. New or progressive multiple-organ dysfunction has been used as an outcome measure for large recently completed and ongoing studies. , Additionally, recent studies have examined the relationship between the number of dysfunctional organ systems and patient outcomes, including in general pediatric critical care patients as well as subgroups of patients with severe sepsis or bone marrow transplants.
Physiologic status is the underlying foundational concept for MOSF and the TISS score. Conceptually, severity of illness may be considered a continuous variable with extremes of outcomes (survival, death) occurring at low and high values. The threshold value determining survival or death is unknown and may vary from patient to patient. Physiologic instability has been an exceptionally productive concept expressed in multiple scoring systems in pediatric, neonatal, and adult intensive care with systems such as the Pediatric Risk of Mortality (PRISM) score, Score for Neonatal Acute Physiology (SNAP), Acute Physiology and Chronic Health Evaluation (APACHE), and many others.
Recently, the development of new morbidity during critical illness has also been related to physiologic instability, with the morbidity risk rising as the instability increases until, at higher states of instability, high morbidity risk transitions to mortality risk. Interest in and investigation of morbidity have been hindered by the lack of measurement methods that are reliable, relevant, and practical for large studies. The development of the Functional Status Scale and its use in a national study of more than 10,000 critically ill children hold promise that morbidity will be a more important and relevant outcome in critical care assessments. Since its publication, the Functional Status Scale has been used to measure outcomes in general PICU patients as well as subgroups of children with traumatic brain injury and other traumatic injuries, those undergoing stem cell transplantation, and those requiring extracorporeal membrane oxygenation.
Methods
Conceptual framework
When possible, the severity method should include variables fundamental to the issues being assessed. The fundamental role of pediatric critical care has been to monitor and treat physiologic instability. The development of severity measures has mirrored this role, first as descriptive categories, then as quantification of therapy designed to treat physiologic instability, and, finally, with physiologic instability itself as the foundational concept. Databases have become larger, and the availability of descriptive, categorical, and diagnostic data that they contain has increased. These data can also be associated with severity of illness and are being used for quality measures such as standardized mortality ratios and measures of severity of illness in academic studies. However, variables such as diagnosis and operative status are proxy variables whose risk estimation is, at least in part, one or more steps removed from physiologic status. Therefore, they are only indirect measures of severity that are vulnerable to “gaming” to alter an individual site’s results. Methods based on primarily categorical data often do not perform well across variable critical care environments.
Statistical issues
Regression analysis is typically the central technique for constructing outcome prediction tools. The type of outcome variable (e.g., continuous, dichotomous) is one determinant of the type of regression analysis used. Multiple linear regression analysis is most often used for models that seek to predict outcomes that are continuous variables (e.g., length of stay). Logistic regression analysis is most often used for models that seek to predict outcomes that are categorical variables (e.g., survival/death).
As data science applications in medicine have become more sophisticated and datasets have become larger, many areas of analysis have incorporated the use of machine-learning models to understand and predict patient outcomes. These can generally be thought of as a continuum of methods to approach data with different strengths and weaknesses. Traditional statistical analysis typically attempts to assign a relationship between a set of variables within a sample, while machine learning attempts to generate a function or pattern that can be generalized for prediction. In general, the data characteristics assumed in a machine-learning approach will be less restrictive than those for traditional statistical modeling. Finally, machine-learning approaches are especially well suited to large datasets, while traditional statistical modeling becomes more unwieldy with more complex inputs. However, as with traditional statistical tests, machine-learning algorithms each have unique characteristics that impact overall performance.
Regardless of how a prediction tool is created, the assessment of its validity centers on two statistical measures: discrimination and calibration. Discrimination is the accuracy of a model in differentiating outcome groups and is most often assessed by the area under the receiver operating characteristic curve (AUC), which is equivalent to the C statistic. Broadly, this represents the average sensitivity of the test when modeled over all possible specificities. An AUC = 1 represents a model with perfect accuracy; an AUC = 0.5 represents a model with no apparent accuracy. A rough guide for model discriminatory performance is as follows: AUC = 0.9–1.0 (excellent), 0.8–0.9 (good), 0.7–0.8 (fair), 0.6–0.7 (poor), and 0.5–0.6 (unacceptable).
Calibration refers to the ability of a model to assign the correct probability of outcome to patients over the entire range of risk prediction. In practical terms for an outcome such as mortality, calibration assesses whether the model-estimated probability of mortality for patients with a particular covariate pattern agrees with the actual observed mortality rate. The most accepted method for measuring calibration is the Hosmer-Lemeshow goodness-of-fit test. Although the AUC is helpful in determining overall characteristics of the test, it does not allow for comparison between the individual specificity or sensitivity of the test. Additionally, as researchers use large datasets more commonly, an artificial increase in the AUC may be seen due to the large sample sizes, particularly when the model is overfit. The use of positive predictive values, which incorporates the prevalence of the queried condition, may better represent the performance of predictive models. Finally, the AUC may be not fully representative of unbalanced patient samples. This is a particular concern with outcomes such as mortality in pediatric critical care, which occur relatively rarely. It remains important to consider a variety of test characteristics when assessing the suitability of a specific test or model.
An important issue in developing and evaluating severity models is the population used to derive and validate the method. The models are based on the populations used to develop them. For example, the Vermont Oxford Neonatal outcome predictor was developed in a large population from inborn nurseries and has been criticized for its lack of applicability to referral centers. The Paediatric Index of Mortality (PIM) and its subsequent updates (PIM2 and PIM3) were developed in predominantly Australian and European populations where the relationship of categorical and physiologic variables to outcome may be different than in the United States or developing countries.
Current prediction tools for assessment of mortality risk
Neonatal intensive care unit prediction methods
Three well-established prediction methods are used for the assessment of severity of illness and mortality risk in neonates: the Clinical Risk Index for Babies II (CRIB II), SNAP-II, and the Vermont Oxford Network risk adjustment. All scores can be calculated during the first 12 hours of life.
CRIB II is the second generation of CRIB, which was developed in the United Kingdom from 812 neonates born at less than 31 weeks’ gestation or weighing less than 1500 g. CRIB II is a simplified version of CRIB, validated on 3027 neonates born at 32 weeks’ gestation or less. It is a five-item score composed of sex, gestation, birth weight, admission temperature, and worst base excess in first 12 hours of life.
SNAP-II is the second generation of SNAP, which was a physiology-based severity of illness score with 34 variables for babies of all birth weights from the United States and Canada. SNAP-II simplified SNAP to six physiologic variables: mean blood pressure, lowest temperature, Pa o 2 /F io 2 ratio, lowest serum pH, seizure activity, and urine output. In an effort to improve the predictive capabilities of SNAP-II for mortality, three additional variables were added: birth weight, small for gestation age, and Apgar (appearance, pulse, grimace, activity, and respiration) score below 7 at 5 minutes. The resulting nine-variable score for prediction of mortality risk was named Score for Neonatal Acute Physiology with Perinatal Extension (SNAPPE-II).
The Vermont Oxford Network is a network of more than 800 institutions worldwide that maintains databases on interventions and outcomes for infants cared for at member institutions. The basic Vermont Oxford Network risk adjustment model includes variables for gestational age, race, sex, location of birth, multiple birth, 1-minute Apgar score, small for gestational age, major birth defect, and mode of delivery, with additional features included in prediction models for very- and extremely-low-birth-weight infants, those with chronic lung disease, or those with birth defects.
Revalidation efforts of these tools employing a variety of data sources have demonstrated largely similar discriminatory abilities among the tools. Using data from the Vermont Oxford Network, Zupancic et al. validated SNAPPE-II on nearly 10,000 infants with similar performance to the Vermont Oxford Network risk adjustment. Within this study cohort, the addition of congenital anomalies to SNAPPE-II improved discrimination significantly. Reid et al. compared CRIB-II and SNAPPE-II in a cohort of Australian preterm infants and found similar performance between the tools and good overall discriminatory ability.
Pediatric intensive care unit prediction tools
The prediction of mortality in the PICU has centered primarily on the use of two different acuity scoring systems, the PRISM score and the PIM3. Historically, these systems have been thought to be quite effective in discrimination but to lack robust calibration.
PRISM is a fourth-generation physiology-based score for quantifying physiologic status and mortality prediction ( Table 12.1 ). The original tool was developed on 11,165 patients from 32 different PICUs in the United States and includes 21 physiologic variables. The mortality predictions are routinely updated, the last update being completed on 19,000 patients. Among PRISM’s strengths are its flexibility to extend beyond mortality prediction to provide risk-adjusted PICU length-of-stay estimates. , Historically, PRISM mortality risk assessments were made using physiologic data from the initial 12 hours of PICU care. Notably, PRISM quantifies physiologic status and uses categorical variables to facilitate accurate estimation of mortality risk.
For computation of mortality and morbidity risk, physiologic variables are measured only in the first 4 hours of pediatric intensive care unit (PICU) care and laboratory variables are measured in the time period from 2 hours before PICU admission through the first 4 hours. See references for the appropriate time periods to assess cardiovascular surgical patients younger than 3 months of age. The neurologic PRISM IV consists of the mental status and pupillary reflex parameters. Only the first PICU admission is scored. Check publications for the most up-to-date prediction algorithms. | ||
Measurement | Score | |
CARDIOVASCULAR AND NEUROLOGIC VITAL SIGNS | ||
Systolic blood pressure (mm Hg) | ||
| 40–55 | 3 |
<40 | 7 | |
| 45–65 | 3 |
<45 | 7 | |
| 55–75 | 3 |
<55 | 7 | |
| 65–85 | 3 |
<65 | 7 | |
Temperature | <33°C or >40°C | 3 |
Mental status | Stupor/coma or GCS <8 | 5 |
Heart rate (beats/min) | ||
| 215–225 | 3 |
>225 | 4 | |
| 215–225 | 3 |
>225 | 4 | |
| 185–205 | 3 |
>205 | 4 | |
| 145–155 | 3 |
>155 | 4 | |
Pupillary reflexes | One fixed | 7 |
Both fixed | 11 | |
ACID-BASE, BLOOD GASES | ||
Acidosis (pH or total CO 2 ) | ||
| >7.55 | 2 |
7.48–7.55 | 3 | |
7.0–7.28 | 2 | |
<7.0 | 6 | |
| 5.0–16.9 | 2 |
<5 | 6 | |
P co 2 (mm Hg) | 50–75 | 1 |
>75 | 3 | |
Total CO 2 (mmol/L) | >34 | 4 |
Pa o 2 (mm Hg) | 42–49.9 | 3 |
<42 | 6 | |
CHEMISTRY TESTS | ||
Glucose | >200 mg/dL or >11 mmol/L | 2 |
Potassium (mmol/L) | >6.9 | 3 |
Blood urea nitrogen | ||
| >11.9 mg/dL or >4.3 mmol/L | 3 |
| >14.9 mg/dL or >5.4 mmol/L | 3 |
Creatinine | ||
| >0.85 mg/dL or >75 μmol/L | 2 |
| >0.90 mg/dL or >80 μmol/L | 2 |
| >0.90 mg/dL or >80 μmol/L | 2 |
| >1.30 mg/dL or >115 μmol/L | 2 |
HEMATOLOGY TESTS | ||
White blood cell count (cells/mm ) | <3000 | 4 |
Platelet count (×10 3 cells/mm ) | 100–200 | 2 |
50–99 | 4 | |
<50 | 5 | |
PT or PTT (sec) | ||
| PT >22 or PTT >85 | 3 |
| PT >22 or PTT >57 | 3 |
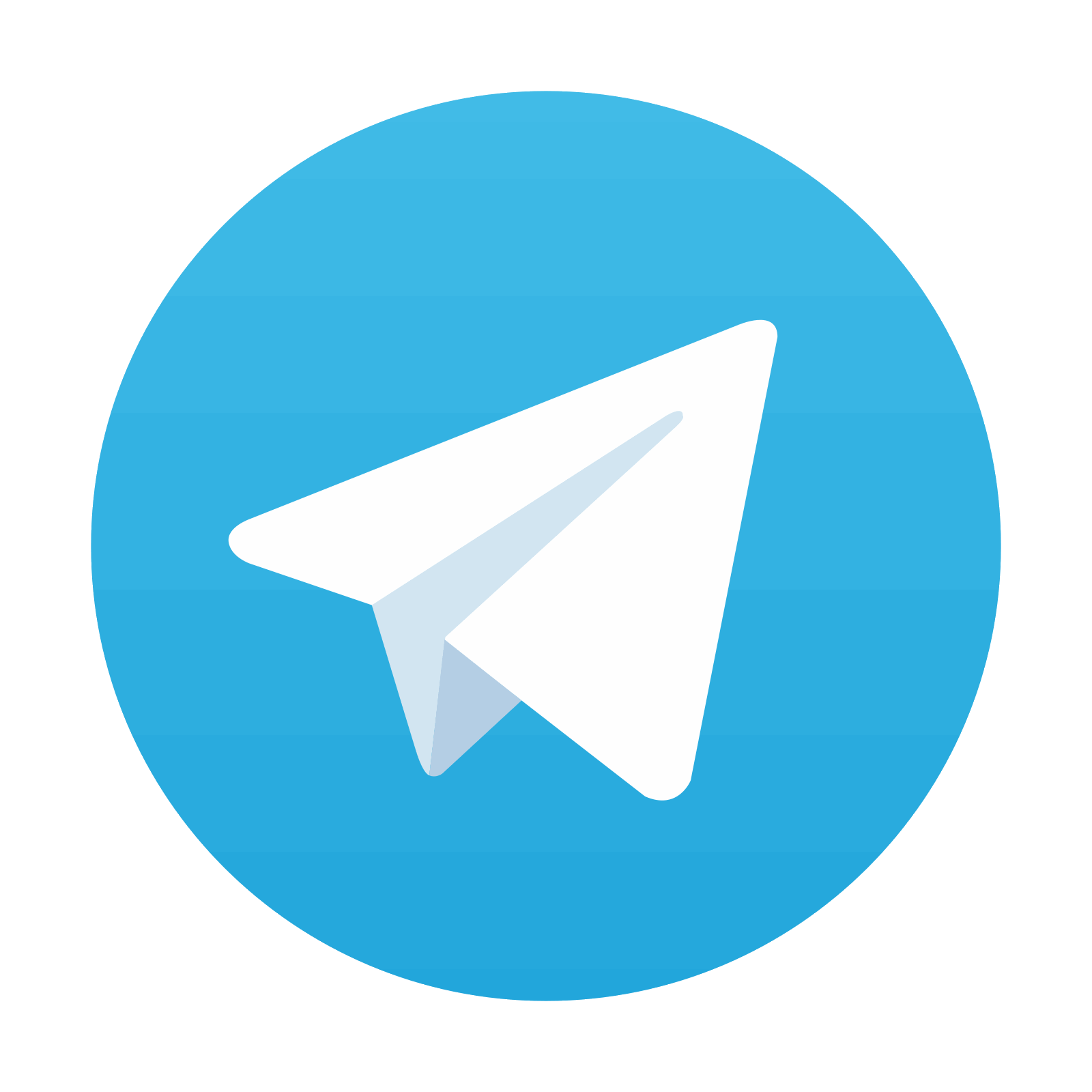
Stay updated, free articles. Join our Telegram channel

Full access? Get Clinical Tree
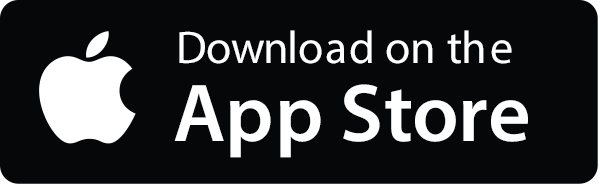

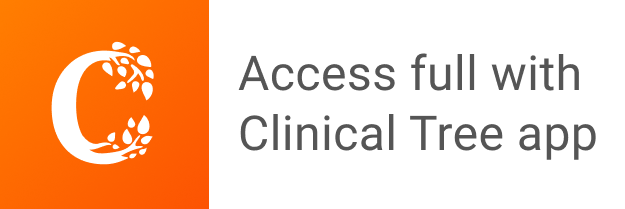