Modeling is the technique of defining a mathematical equation that fits a data set as accurately as possible. Linear regression could be considered as a rudimentary form of modeling. A linear regression analysis of a data set determines the best-fitting linear correlation between data couples by minimizing the sum of squares of all perpendicular distances from each observation to the proposed line (least-squares principle). As such, the optimal value for parameters a and b in an equation of the form y = a ⋅ x + b can be determined from an observational data set. The resulting linear equation describes the correlation of the data couples within the population with the least possible scatter (or residual error). Consequently, the resulting equation can be used to predict prospectively which y can be expected for every known x, with the smallest possible error.
In many pharmacologic processes, the equations that best describe the data are nonlinear in nature. For example, the classical dose- or concentration-response curve is generally described as a sigmoidal Emax curve, also called the Hill equation. This equation includes 4 parameters that need to be estimated from data: baseline value of the effect variable (E0), maximal possible effect obtained at high doses (Emax), the effective dose or concentration related to 50% of Emax (ED50 or EC50), and the slope of the dose or concentration versus effect curve (γ). The ED50 or EC50 represents potency of the drug, and Emax reflects efficacy of the drug.
In order to fit nonlinear equations (also called structural models) on a data set, a statistical approach called nonlinear mixed effects modeling (as performed by the software package NONMEM (ICON Development Solutions, Hanover, MD) has become the standard methodology. Several structural models have been applied on anesthesia interaction data. We will review the major differences between structural models later.
In NONMEM, the optimal structural model is selected when it results in the lowest objective function value (OFV), that is, the minimum value of the “–2 log likelihood” function. A low OFV reflects the ability of the model to describe the data with the least amount of scatter. Mixed effects refers to the combination of fixed effects and random effects. Fixed effects are sources of variability in the observation that are the result of covariates that we can measure. For example, we can categorize the studied subjects into relevant groups according to differences in fixed effects (eg, age, weight). If the inclusion of a fixed effect in the structural model improves the fit on the data set significantly, this covariate is considered a relevant improvement for the predictive performance of the model. On the other hand, even after having defined a number of significant fixed effects in the structural model, a residual amount of error will always remain. This is caused by random effects—for example, interindividual variability and residual error. One can only speculate about the causes of these random effects. They are the result of all mechanisms (biological or technical in nature) that evoke the biological variability in individual patients and individual observation. The random effects can therefore never be fully controlled, but they can be quantified as a level of uncertainty in the predictions of the model.
When deciding on which methodology to use for quantifying interactions of drugs on a clinical effect, several well-known sources of variability should be considered (and possibly controlled) in advance. First, a careful definition of the relevant end points of effect needs to be decided. Secondly, maximal reproducibility of the pharmacologic condition during the observations is mandatory.
The therapeutic drug effects from the various components of anesthesia (hypnosis, immobility, analgesia) are commonly measured and expressed as dichotomous clinical end points in the individual patient, or alternatively as a “probability of response” in the population. The dichotomous approach describes the presence or absence of a cognitive, motor, or autonomic response to a verbal, tactile, or painful stimulus. Examples of commonly used stimuli to test responsiveness are loud or repeated name calling, eye lash reflex, shake and shout, a movement to a painful stimulus such as trapezius squeeze, tetanic electrical stimulus or tibial pressure, introduction of a laryngeal mask, laryngoscopy, tracheal intubation, and surgical incision. With these end points, no gradual change in effect can be observed. A response can only be either present or absent. To bypass this shortcoming, stimuli with progressively intensified arousal capacity are applied in consecutive steps, in order to obtain a gradual impression of the clinical onset of anesthetic effect in the individual patient. The observers assessment of alertness and sedation scale (OAAS) is an example of such a gradual intensifying stimulation test.1,2 A modified version of this scale (see Table 3–2) is commonly used as a reference of clinical effect of anesthesia.
By means of logistic regression, dichotomous individual observations can be translated into a probability of response within the population. The minimal alveolar concentration (MAC) is a well-known example of a population-based measure of drug effect. One MAC of an inhaled anesthetic represents the minimal alveolar concentration needed to evoke a 50% probability of immobility in a population of patients after applying a “standardized” incision.3
Continuous measures can also be used to describe anesthetic drug effects. For example, cerebral hypnotic drug effects can be quantified by electroencephalographic-derived indices (such as Bispectral Index [Covidien], M-Entropy [Datex-Ohmeda]).1,4 Monitoring the cortical activity during anesthesia has contributed largely to a more detailed understanding of the neurophysiologic processes involved in anesthesia and even has triggered a new functional definition of anesthesia.5
In contrast to hypnotic effects, the continuous quantification of the balance between nociception and antinociception remains a challenge. A search for new measurements of this complex concept is still ongoing.6,7 By evaluating the relation between interacting drug combinations and the output of alleged monitors of nociception-antinociception may help differentiating their usefulness for clinical use. Such studies should deal with the fundamental issue concerning how the dose-response curve between interacting drugs and the continuous measures of hypnotic or analgesic effect are influenced by the balance between opioids and hypnotics.
The observations of anesthetic effect can be performed either in pharmacologic non–steady-state or, preferably, in steady-state conditions. The pharmacologic conditions obtained at the biophase should be as reproducible as possible in order to define interaction models that have direct clinical applicability. Therefore, all methodology developed for studying anesthetic interactions should include a precise description of the method of drug delivery. Currently, modern vaporizers and target-controlled infusion pumps provide excellent tools for the clinician to titrate drugs toward the desired effect-site concentration in a reproducible way.
The end-tidal concentration of an inhaled anesthetic is closely related to the individual plasma concentration of the drug. When kept constant over a sufficiently long equilibration time, a steady-state concentration at the effect site can be assumed. For intravenous anesthetic drugs, there exists no direct individual measurement of plasma or biophase concentration. Therefore, population derived pharmacokinetic-pharmacodynamic (PKPD) models are used to predict the plasma-concentration and effect-site concentration with the least possible error. Much progress has been made in the clinical applicability of contemporary PKPD models, but the use of population estimations—by definition—remains open for prediction errors in the individual patient8. Still, as many of the population PKPD models used in interaction studies are commercially available, any anesthesiologist should be able to reproduce the effective drug concentrations described in the model estimations. When studying interactions, computer-controlled drug administration for total intravenous anesthesia is strongly recommended. It standardizes the speed of drug infusion, the timing and magnitude of bolus and maintenance infusions in such a way that the resulting effects reach a higher reproducibility compared to manually administered drug titrations.
Studying drug–drug interactions requires a specific study design for optimal data collection. Short et al performed simulations for several study designs in order to determine the most efficient inclusion methodology.9 The researchers showed that the optimal design for an interaction study (with minimum number of patients and maximal accuracy in prediction of the observed responses) is a so-called “criss-cross” design. In this setting, the study population is randomized to 2 groups. Group 1 receives drug A as a constant pseudo–steady-state effect-site concentration at several predefined targets, while drug B is administered in brief zero-order step-up infusions. Group 2 receives drug B in a constant amount, while drug A varies to maximal effect. This method appears to provide sufficient spread in the data for accurate model building while only including a limited number of patients for the study. However, the exact number of patients as concluded by the study of Short remains open for debate as this number is only valid when all assumptions made by Short are met.9
Several mathematical approaches have been proposed to model interaction. A short review of the advantages and disadvantages of currently proposed mathematical equations is therefore discussed here.
Isoboles are lines that represent conditions of equal effect in relation to the doses (or concentrations) of 2 interacting drugs, respectively, plotted on the x- and y-axes (Figure A3–1). The isobole is the line that connects all drug combinations that evoke an equal effect of interest (eg, the 50% probability of response to incision), including the dose of a solitary administration of drug A or B. Many methods have been proposed to fit isoboles on clinical data sets ranging from drawing by hand to methods that add more statistical rigor. For a thorough review of all these methods, we refer the reader to other publications.10 In general, a major advantage of the isobolographic approach is the relative simplicity of the statistical method and the availability of software to do so. However, 1 isobole does not contain information on the total spectrum of desired anesthesia effects. For example, a strong synergism between drugs A and B, observed at the 50% probability of response level, does not guarantee a comparable intense synergism at the 95% probability of response. If only the 50% probability of response is targeted during the study, information on more intense levels of drug effect might not be extractable from the data set.
Figure A3–1
Comparison of estimations from 4 interaction models using isoboles for 90% probability (P = 0.90) of tolerance to laryngeal mask airway placement (TMLA). The thin dashed line represents the reduced Greco model, the thin solid line represents the Minto model, the dotted line represents the scaled C50O hierarchical model, and the thick solid line represents the fixed C50O hierarchical model.

For a single drug, the natural logarithm of the odds ratio of drug effect (called the logit) can be expressed in terms of drug concentrations.11

where P is probability of response, β0 and β1 are estimated parameters, and C is the concentration of the drug. For multiple drugs, this can be expanded by using a linear function of the concentrations of both drugs as shown in Eqn. 2.
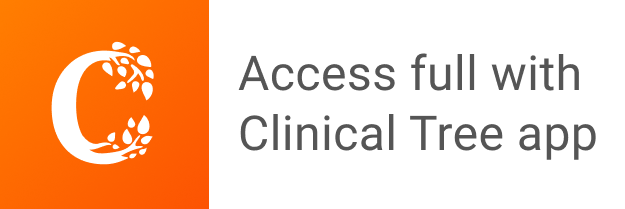