div class=”ChapterContextInformation”>
19. Learning Healthcare System Principles to Facilitate Spread of DCR
Keywords
Learning healthcare systemEmpowering cliniciansAdaptationData-driven decision-makingManaging fearQuality improvementSpread of innovationsTests of changeLeadershipMeasurement and data systemsIntroduction
The fields of healthcare and public health regularly generate new innovations and evidence-based practices designed to alleviate suffering, improve quality of care and help the system function more efficiently. But while there is no shortage of well-established ideas about how to improve performance, disseminating what works remains a challenging and, at times, elusive enterprise [1–4].
The medical community and the reader alike now know what Damage Control Resuscitation (DCR) is and how vital it can be when used “with the right patient, in the right place, at the right time” [5, 6]. However, many brilliant and powerful healthcare interventions like DCR are not implemented broadly or reliably, particularly when the added complexity of cooperation across disciplines, environments, and specialty domains are introduced. As such, those seeking to introduce DCR into their system must think critically about the ways by which they, along with their teams, will introduce and embed the intervention within and throughout their system, ultimately creating a sustainable process. There is a clear intrinsic motivation within all providers for delivering innovations like DCR—indeed, any interruption in delivery of this approach risks less than optimal outcomes and ultimately the loss of human life or a reduction in the quality of life.
Adopting the principles of a learning healthcare system (LHS) can create an engine for embedding research-based and evidence-supported interventions and practices like DCR into clinical care. This chapter will provide an overview of an LHS, describe how it constantly learns, and offer an example from Intermountain Healthcare. Following these, a description of how an LHS can ideally implement and improve the practice of DCR will be discussed. An important facet of DCR is the need to bring DCR to the patients most in need and at an early interval after life-threatening injury and exsanguination. Thus, we will address how different components of the trauma LHS (prehospital, en route, and in-hospital care) must integrate data, experience, and report outcomes to continuously learn, identify gaps, and direct further research and improvement. Prior to concluding, the chapter will briefly look at what it might take to introduce DCR at national scale.
An Introduction to Learning Healthcare Systems
The incorrect belief that simply publishing new innovation is sufficient to lead to its adoption [1]
The sheer volume of new information (publications, studies, protocols) that most healthcare professionals in the healthcare system have to process
Management incentives, such as ranking by individual performance rather than by team performance, that create fear and limit learning
The logistical and operational challenges of introducing innovations into new settings and contexts, with all of their attendant differences
The fundamental imbalance in access to care, quality of care, and care outcomes experienced in particular geographies and by different groups (e.g., people of color) and cultures in the American healthcare system [9, 10]. These inequities are sometimes replicated with relation to acquisition of new knowledge and practice, thereby hindering learning and spread and heightening maldistribution of outcomes [9, 11, 12]
Equity here is defined as achieving equally high outcomes for all patients removing the predictability of success or failure that in some areas of healthcare correlate with social or cultural factors; any learning system must address sources of inequity, including cultures that preference certain races, classes, and genders [13, 14].
Learning systems that effectively introduce new innovations operate in an exceptional way that proactively mitigates for these barriers. Specifically, LHSs understand the inherent patterns of behavior and cultural norms in the systems and organizations in which they operate; refine their interventions thoughtfully to meet the needs, requirements, and values of all adopting audiences; pursue many strategies to raise awareness and deepen will for change; and offer many forms of support for adult learning and implementation of new practice via a variety of adult learning methods [15, 16]. Lastly, LHSs have styles of operating that are highly tolerant of rapid iteration, adaptation, and learning [17].
Comparing typical with exceptional learning systems
Typical learning systems | Exceptional and transformational learning systems |
---|---|
The aim of the network is to facilitate general learning No sense of direction or urgency, so making progress is not the goal | The system-level aim of the network is crisp and quantifiable The aim focuses action and induces cooperation toward a shared vision of success |
The system is oriented to focus upward in the hierarchy; leadership receives data and reports The system’s capacity to learn is limited because the orientation is toward leadership rather than the front lines and the patient | The system is oriented to focus on the needs of the patient/customer; leadership removes barriers to progress in the field Frontline providers are empowered to deliver quality care based on their deep contextual wisdom |
The system catalogues explicit knowledge (libraries) Inadequately contextualized information, not designed to help practitioners change behavior | The system facilitates the exchange of tacit knowledge Focused on sharing the emergent tricks of the trade and pragmatic how-to’s |
The system furnishes aggregate information Bright spots and areas for improvement are combined, so opportunities for learning are lost | The system measures site-level performance and disaggregates data by race and class wherever possible Allows for learning about local adaptations, and helps motivated teams improve |
A large investment is made in teaching and training Fosters passive learning | Experimentation and improvisation are encouraged or required; there are high expectations for testing and adjustment Providers constantly apply new knowledge and learning to meet outcome goals for patients |
Consensus is the primary approach to decision-making While important under certain circumstances, consensus can be time consuming | Agility is valued, and a variety of decision-making approaches are used depending on the stakes/risk involved Allow stakeholders and caregivers to have the license to explore different approaches toward shared aims |
New networks are created Work intensive and potentially inefficient | Existing networks are harnessed Other communities are likely working on the same problem. Collaborating is mutually beneficial |
The system is centrally managed Can be an impediment to rapid learning | System management is distributed; losing control is viewed as success Frontlines are laboratories for learning |
Leadership
In order to launch and maintain an effective DCR program using the principles described above, leadership at every level must support the constant improvement of knowledge, experience, and study of DCR with all stakeholders throughout the delivery system. There are many existing definitions of leadership, but when it comes to establishing and maintaining learning health systems, we appreciate Marshall Ganz’s definition: “Leadership is accepting responsibility to create conditions to enable others to achieve shared purpose in the face of uncertainty” [21]. The function of leadership in a transformational LHS is to create an ongoing practice of identifying, recruiting, and developing leaders at all levels and supporting one another’s personal growth and professional development [17, 22, 23]. A workplace or community with more leaders of this type will be more effective and interconnected than those with a more traditional linear reporting structure.
Looking to Ganz’s definition and the literature on leadership and leadership in healthcare, what might it look like to create conditions to enable others to achieve? We propose that leaders must take a hard look at existing policies, standard procedures, and incentive systems to understand what behaviors and attitudes are being reinforced [21, 23]. There is no such thing as a neutral system—the structure of any organization and community expectations for performance are always buttressed by deeper intersubjective beliefs. Methods like design thinking’s empathy interviews, colleague shadowing, and 360 feedback processes can be powerful experiences to gain an understanding of those deeper beliefs.
Measurement and Data Systems
Any attempt at systemic quality improvement will need to create and tend to multidisciplinary measurement to ensure that teams are making progress toward prioritized goals. Effective measurement systems are designed to give frontline clinicians expedient access to formative data and empower those frontline clinicians to use data to inform their delivery of care. Less effective measurement systems, on the other hand, tend to provide summative data oriented toward leadership, which are used for clinician evaluation and even punishment based on outcomes. These ineffective measurement systems are not designed to aid frontline learning, which makes them less accessible in the critical moments of delivery of care.
In the highest performing LHSs, data systems are structured from the bottom-up rather than the top-down. Provider-level data counters inertia with a sense of urgency and can motivate staff into action [21, 24, p., 278]. Aggregate data showing exclusively high-level system performance hides trends and reduces the likelihood of learning. This approach of acting and reflecting at the team and individual provider data reinforces the clinical gaps, reveals areas for improvement, and at times, demonstrates how to promote learning. Using patient data to generate clinical evidence is extremely important to an LHS. Registries, which allow for timely analysis of and quick access to patient data, are also enormously useful [18, 24].
This process starts with gathering data while treating patients and developing information that leads to actions to be incorporated into future practice. Quality improvement gradually becomes a routine and perpetual process, helping all stakeholders optimize outcomes with and for patients by using evidence-based practices and interventions.
What a Learning Healthcare System Can Look Like: Intermountain Healthcare’s LHS

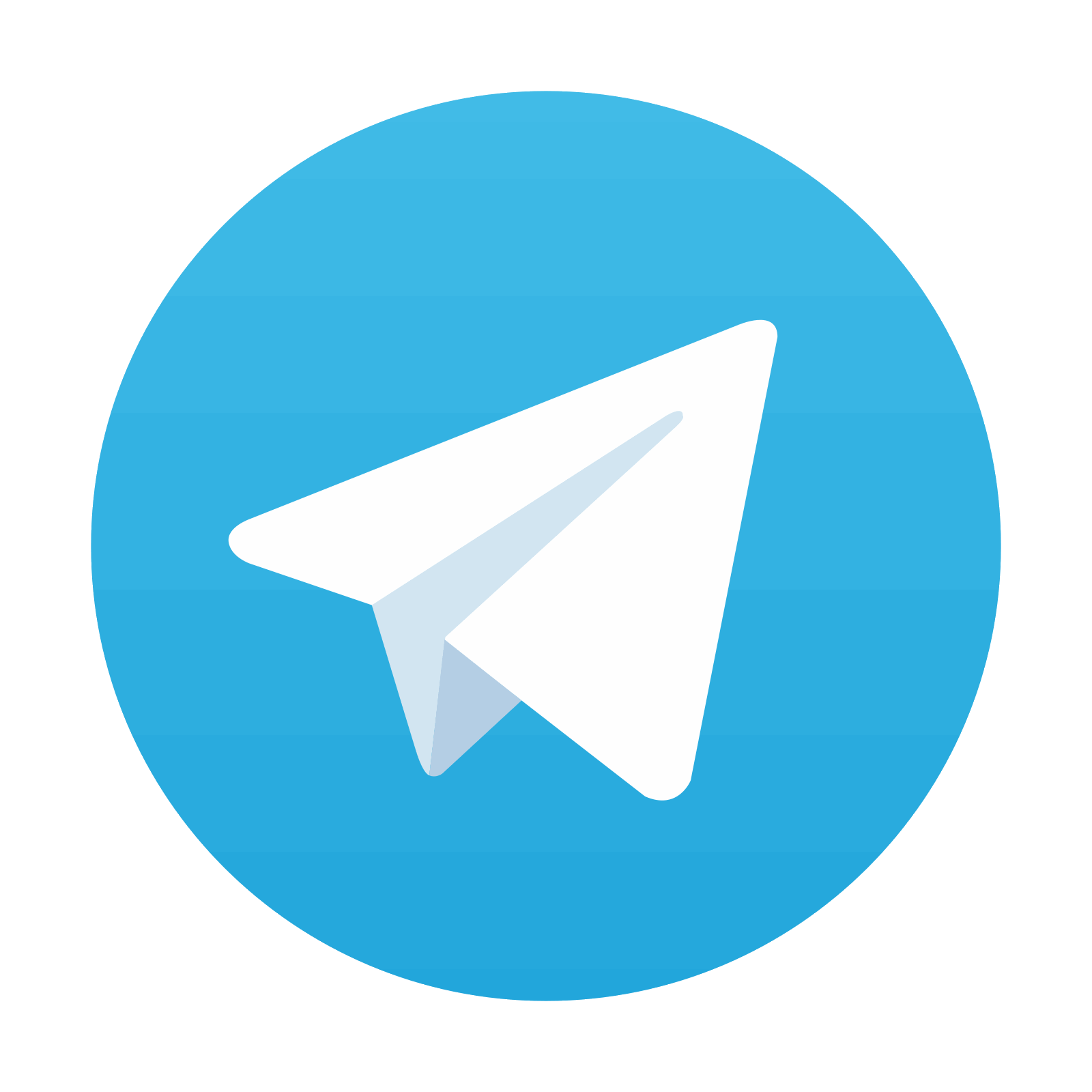
Stay updated, free articles. Join our Telegram channel

Full access? Get Clinical Tree
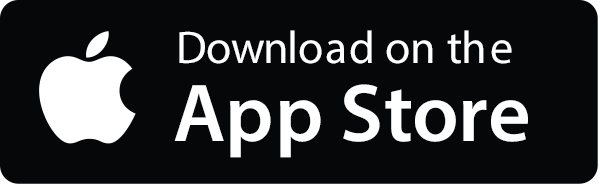
