The technical term geographic information system (GIS) describes a relational computer system that captures, stores, manages, retrieves, analyzes, and displays geographic data. Practically understood, a GIS allows users to understand the “where” dimensions of their work and to relate place to information about that place, especially information about population. Everything happens in space and time is a fundamental principle of disaster response and of epidemiology, the population-based science that is part of public health practices. GIS provides a way to visualize how populations are dispersed, how they migrate, how they are affected by their environment, how they affect their environment, and their relationship to all manner of critical variables, outcomes, and risk factors.
At its core, GIS consists of hardware, software, and management systems that rely on an array of computing technologies for capturing and processing geographic data. As such, it is a valuable mapping and analysis system. Geospatial technologies that capture data include global positioning systems (GPSs), remote sensing, and map scanning and digitization. Most people are familiar with the portable GPS receivers that capture locations of latitude and longitude from orbiting satellites and Earth-bound satellite sensors. Remote sensing refers to data gathered away from the Earth’s surface. Remote sensing involves sensors linked to platforms and includes modalities such as aerial photography (cameras on aircraft or unmanned aerial vehicles) and satellite imaging with digital image processing (cameras, digital scanners, light detection and ranging [LIDAR]). Optical lasers and other reading devices can also scan maps into digital formats or convert map features into geospatial data.
Geospatial data by definition are observations and measurements linked to locations on the surface of the Earth and are graphically represented as two models: the raster model, which is represented as pixels or cells; and the vector model, which include points, lines, networks, and polygons (areas with vectored boundaries). The former contains continuous data with values that convey units of space on the Earth’s surface (such as land use, elevation, or population) and can vary in size, determining the degree of resolution; the latter has a single class of objects with similar dimensions. The vector model tends to be more geographically accurate and lends itself to common mapping and operational graphing queries for items such as transportation lines, area boundaries, points of interest, etc. Spatial features also include attributes, additional information about a data feature in tabular form. For example, a field hospital is a spatial feature, identified by a spatial locator (coordinate) represented by a point (in a vector layer), with tabulated attributable information items such as number of personnel, types of services and specialties, number of beds, among others.
Once digitalized spatial data are acquired, computer graphics software can create maps with an array of attributes and variables. Exploring geography through digital means enables mapping, and mapping provides a visual tool for targeted interventions and efficient response: for disaster managers and responders, a map of “who is doing what and where” is an invaluable tool. With current graphic technologies, mapping is interactive and functional, especially as conditions on the ground rapidly change and the dynamics of the response efforts need to be plotted in real time.
Most importantly, GIS is a relational database. Geographical data are referenced to places on the Earth’s surface as coordinates of latitude, longitude, and elevation; it is these coordinates that link a variety of raster and vector data layers together. Any place on Earth, as long as it has a geo-reference, can be spatially represented. Thus any variable that can be geo-located with these relational spatial coordinates—health facilities, population demographics, transit lines, power stations, socioeconomic metrics, phone calls and communications networks, Earth features such as topography, climate, vegetation, soils, variables linked to natural hazards, among many other examples—can be linked spatially and often temporally, not only providing a graphic visualization of place and links to attributes, but also opening up possibilities for spatial analytics.
Spatial analysis, a toolkit afforded to GIS software (ArcGIS and QuantumGIS), allows one to investigate geographic patterns in spatial data and the relationships between features and, if needed, to apply inferential statistics to determine the relevance of spatial relationships, trends, and patterns; to see if “what is next to what” and “what is connected to what” have significance. The concepts of “nearness” and “relatedness” have at their core geographic significance, and the relationships among things that are near can generate complex spatiotemporal phenomena. Yet nearness does not necessarily imply causation. Spatial analytics affords the opportunity to take spatial characteristics into account to make those determinations. The concept of spatial autocorrelation is the name given to the degree to which one object is similar to other near objects and helps determine if objects are spatially independent from each other; this correlative statistic may be positive (similar values cluster together spatially, such as an emerging epidemic), negative (dissimilar objects cluster together spatially), or something in between. Flow-through networks to maximize time and efficiency, impacts of distances on health, impacts of typology on health services delivery, potential environmental hazards, and risk of death or injury are all examples in which spatial features are the critical element of analysis. In multivariate health outcomes studies, spatial features may need to be included in the regression if one is, for example, to fully appreciate the geographic variation of health outcomes in exposed and unexposed groups. Spatial statistics help see spatial variation in the relationships between factors contributing to disease and health outcomes. Once spatial effects are established, they can be used in risk modeling ( Box 49-1 ).
Space refers to position or location. It describes the geographic distributions of constraints and opportunities that influence and result from human activities and interactions. In contrast, place is a relational concept that addresses the human meanings and experiences associated with particular locations. Social relations and the physical sites of everyday life are intertwined in the concept of place.
Historical perspective
Intuitively, geography has always played a role in disaster response, even if originally used in the form of stagnant logistical tools to inform organizations about where to target programs and how to shuttle resources. With the incorporation of epidemiological methods and the evolution of computer and communication systems, GIS has progressed beyond the use of often incomplete, outdated maps to a complex and interdisciplinary field that includes advanced mapping and data collection techniques, crowd-sourcing, participatory mapping and needs assessment, hazard modeling, operations research, and programmatic decision support, all of which inform the many phases of disaster management and subsequently disaster medicine.
Aerial Photography, Satellite Imaging, and Remote Sensing
After centuries of parchment and compasses, cartography transformed significantly during the twentieth century with the advent of aerial photography, satellite imaging, remote sensing, and advanced mapping software. But not until the 1990s did this advancement revolutionize the field of disaster management. In response to the Japanese earthquakes in Hokkaido and Kobe that took place in 1993 and 1995, respectively, along with the Northridge California Earthquake in 1994, governmental organizations used aerial photography and satellite imaging to delineate damage zones and characterize infrastructural casualties. In 2001, after the attacks on the World Trade Center, light detection and ranging imagery (LIDAR), satellite imagery, and handheld GIS-linked devices were used to support rescue and recovery operations.
As humanitarian programmatic design evolved, operatives needed more than geographic information to advance disaster response initiatives, so GIS platforms began to incorporate thematic overlays into mapping. In response to the 2004 Indian Ocean Tsunami, the United Nations (UN) Environmental Programme reported critical gaps in geographic, infrastructural, and disaster impact information. Preexisting maps maintained by the Indonesian national mapping agency were lacking accurate and timely data regarding infrastructure; there was a lack of vulnerability and risk assessment mapping and environmental baseline data, and minimal field assessments hindered any appropriate response. Acknowledging this dearth, the UN Humanitarian Information Center, using ArcGIS, augmented old topographic maps with satellite imagery and then applied thematic layers to indicate damage assessments, displaced populations, injured peoples, and organizational distribution. During this campaign, GIS was used to create potable water, food, and medical supply chains; assess village mapping and inform community planning; determine field hospital and mobile health clinic locales, and monitor for communicable diseases.
Expanding Agency: Crowd-Sourcing, Participatory Mapping, and Collective Intelligence
Conventionally, humanitarian programs and their GIS counterparts have been led by centralized, top-down teams that grapple with vast amounts of information and disseminate culled data and subsequent analyses with a significant amount of discrimination in terms of what is graphically presented. Recent developments in web-mapping, mobile technologies, and open platforms have led to “a kind of collective intelligence” that decentralizes disaster response, empowers affected communities, and capitalizes upon the vast capacities of a technologically savvy, cosmopolitan population. Forays into open participation occurred as early as 2001, when volunteers were deployed from the Emergency Mapping and Data Center in Manhattan to gather much of the early GIS data after the 9/11 attacks, and in 2004, with the creation of the Chuetsu Earthquake disaster and recovery GIS open website project. Amidst the aftermath of the violence following the 2007 Kenyan elections, Ushahidi launched a crowd-sourced, open-platform initiative that collected eyewitness reports of violence via E-mail and Short Message Service (SMS) texts and overlaid them onto Google Maps.
But in 2010, following the Haitian earthquake, community-sourced information and open participation truly came to the fore. Volunteer and technical communities (V&TCs) emerged, utilizing the input of affected populations to provide real-time, ground-level data. The voice of the community, empowered by more than 3 million Haitian cell phone users, was incorporated to rebuild maps through OpenStreetMap, identify health facilities, and, via SMS texting, to augment needs assessments surveys. Internet collaborations between V&TCs emerged for aggregation and analysis of this overwhelming amount of data. Since that time, open participation in mapping, needs assessment, and data analysis continues to blossom, with disaster response efforts ranging from the Ushahidi Missouri River Floods project in 2011 to Crisis-Mapping of the 2010 Chilean earthquake and the employment of OpenStreet map by the UN High Commissioner for Refugees (UNHCR) in the Zaatari refugee camp in Jordan following the Syrian conflict.
Hazard Modeling, Operations Research, and Geographic Information System for Decision Support
One of the first uses of GIS for hazard modeling and disaster preparedness was the Sea, Lake and Overland Surges from Hurricanes (SLOSH) program. First developed in the late 1960s, SLOSH was originally created for the purpose of forecasting weather, but it soon became apparent that SLOSH’s hydrologic modeling could be used as hazard analysis to delineate communities susceptible to surge flooding and to design preparedness programs. In 2005, after decades of permutation, these data, initially disseminated to organizations via print maps, were now presented in graphical, often animated form via the SLOSH Display Program to the Federal Emergency Management Agency (FEMA), the Army Corps of Engineers, and state and local emergency managers during Hurricane Katrina. Similar hazard models have been developed for identifying populations vulnerable to natural disasters such as earthquakes, floods, wildfires, and landslides and have been integral to international disaster risk assessments, as illustrated through the Natural Disaster Hotspots project and the UN Development Programme (UNDP)’s Disaster Risk Index. Incorporation of preexisting community health demographics into hazard modeling has led to a richer understanding of the postdisaster medical needs of an affected community—invaluable knowledge that undoubtedly fosters a more comprehensive and competent disaster preparedness plan. Further interdisciplinary efforts wedding hazard modeling and operational research (OR) have bred powerful decision support tools that address evacuation routing, optimal resource allocation to emergency shelters and clinics, and supply chain and distribution schema.
Notwithstanding these exponential advancements made in the field of GIS and its incorporation into disaster response, there continue to be challenges surrounding uptake, interoperability, data-verification and security, residual logistical obstacles, and information sharing. While these pitfalls are indeed formidable, as discussed in the following, technology does nothing but evolve, and its role in disaster response will undoubtedly continue to burgeon.
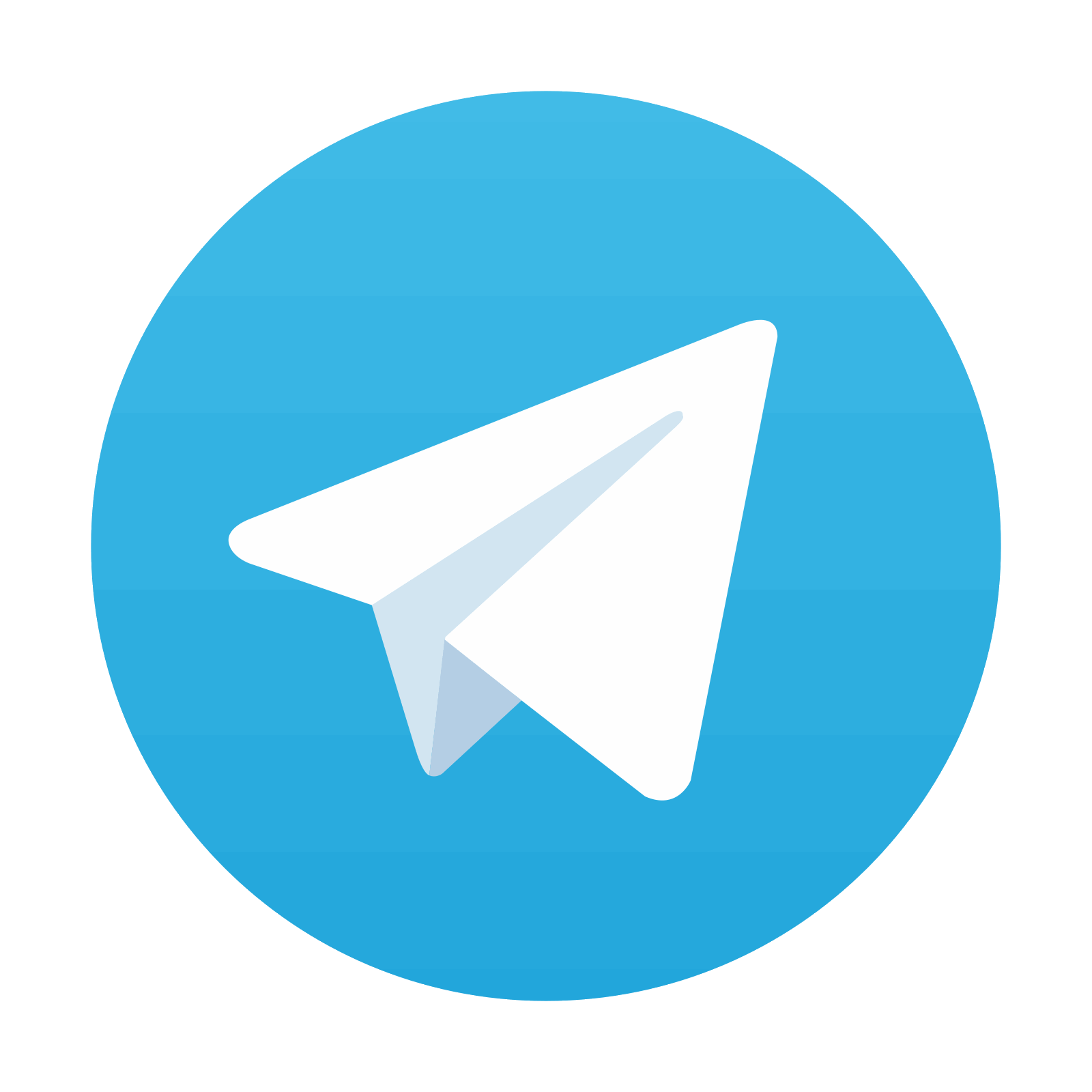
Stay updated, free articles. Join our Telegram channel

Full access? Get Clinical Tree
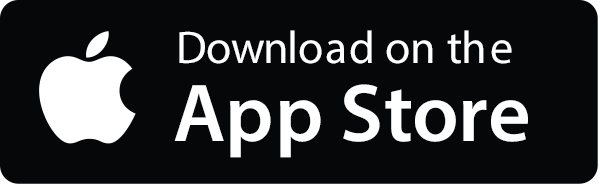
