Pearls
- •
Clinical trials require appropriate design, conduct, and analysis in order to provide valid, unbiased, and reliable results that will be useful to clinicians.
- •
Trial design is more important than analysis; while statistical analysis can be adjusted at any time, study design flaws that introduce bias cannot always be corrected after a study is complete.
- •
Critical design elements include selecting the appropriate population, determining the necessary sample size, and selecting a clinically meaningful, readily interpreted outcome to study.
- •
Randomization, blinding, and intention-to-treat analysis reduce bias in study results.
- •
Statistical analysis aims to estimate the treatment effect, calculate uncertainty around that estimate, and calculate the likelihood that the effect was identified by chance.
“You can best learn statistical methods by applying them to data which interest you.” —PETO, PIKE, ARMITAGE, ET AL.
Purpose of a clinical trial
Before recommending an intervention (e.g., a medication, therapy, or practice) to a patient, clinicians need to know whether it is effective. When asking whether a medication is more effective than placebo, or whether it is superior to another medication, or whether a new screening practice improves outcome, a trial creates a standardized setting in which researchers can determine whether that intervention has the desired effect.
The ability of a clinical trial to demonstrate the effect of an intervention reliably rests on its design. Trials that answer a clinically relevant question, adequately control bias, and reduce errors due to chance with strong external validity will most inform clinical practice. The gold standard in assessing the efficacy of a therapy remains the randomized controlled trial (RCT). RCTs provide the bulk of evidence about interventions of interest to the medical community and are the focus of this chapter. RCTs require careful design, conduct, analysis, reporting, and interpretation. By understanding these fundamental aspects of clinical trials, clinicians can better interpret the results of new medical research and better incorporate them into practice.
Clinical trial design
Getting started: Question and hypothesis
The first step in clinical trial design is identifying a clinical question for which a randomized trial is feasible. Feasibility requires that the investigator be able to control the treatment exposure, that equipoise exists about whether it works, and that the outcome of interest happens commonly enough and soon enough for it to be observed in a study. Randomized trials cannot answer all questions; questions about the epidemiology, risk factors, and natural history of an illness require observational studies.
If the treatment and outcome support conducting a clinical trial, researchers must next demonstrate equipoise about the treatment. Individual equipoise refers to a clinician having no preference between two treatment options. Collective equipoise refers to uncertainty or disagreement among clinicians as a community about the superiority of one treatment. Ethical oversight committees require researchers to demonstrate that sufficient collective equipoise exists to justify subjecting patients to the potential risks of a study. ,
The clinical question must be worded in a testable format, clearly specifying the population, intervention, comparison, and outcome (PICO criteria) of interest. For interventional trials, researchers state a null hypothesis, usually that the intervention will have no effect, against which the results will be judged. The null hypothesis states what the researcher is trying to disprove . For example, if a researcher wants to examine whether surfactant improves mortality in children with pediatric acute respiratory distress syndrome (PARDS), the null hypothesis might state: “Mortality in children with PARDS who receive surfactant is equal to the mortality of children with PARDS who do not receive surfactant.” Statistical analysis then allows comparison of the observed outcome to the null hypothesis, including mathematical estimates of uncertainty and calculations of the probability that the observed effect is due to chance.
Target population: Minimizing variation versus generalizability
Another challenge in study design is identifying the optimal study population. A more homogeneous population may help the study identify an effect of an intervention at the expense of limiting the generalizability of the study results and potentially slowing enrollment. Conversely, in a broader, more generalizable population, the effect may be diluted—or even lost—if the intervention is only efficacious in a subset of study subjects. Enrolling a truly representative population for the clinical question reduces selection bias. For example, in the theoretical PARDS study, selection bias may occur if clinicians only refer patients with mild PARDS for enrollment. The results of this study may not accurately describe the risks and benefits of surfactant therapy among patients with severe PARDS; therefore, the results may not be generalizable to the actual patient population of interest.
Power and sample size
A study needs to enroll a sufficient number of patients to obtain an accurate estimate of the true treatment effect; the ideal sample size is a consequence of several factors. A smaller magnitude of intervention effect and a lower baseline outcome frequency will both increase the sample size needed to achieve a given power. For example, if a disease typically causes 30% mortality, which will be seen in the control arm, and a treatment is expected to reduce mortality to 10%, the estimate of effect is a 20% absolute risk reduction (and a 67% relative risk reduction ) in mortality. This will be easier to identify and will therefore need a smaller sample size than a study of a treatment expected to reduce mortality to only 25% (a 5% absolute risk reduction and 17% relative risk reduction). If the baseline mortality is only 10% to begin with, then observing enough events to see a difference between the control and treatment groups will also require a larger sample size.
The next step is setting an acceptable threshold of observing a given treatment effect by chance alone, when no effect actually exists. This is referred to as type I error , represented by α. Larger sample sizes are needed to achieve smaller type I error. The P value is the probability that the observed difference (or one greater) in a study would have been found by chance or coincidence if no effect were present. If the mortality in a study’s intervention arm is 10% versus 30% in the control arm, with a P value of .01, then in only 1 out of 100 trials would we find this 20% (or greater) absolute difference in mortality if no effect actually existed (if the null hypothesis were true). This seems unlikely, although not impossible. Conventionally, P values that are below an α threshold of .05, indicating a less than 1 in 20 probability that the study results are due to chance, are considered statistically significant. However, because the P value is a probability, even a significant P value of .04 means that 1 out of 25 times, investigators will find a difference where none actually exists. The appropriate P value threshold for a study depends on what is at stake with the clinical question.
The sample size calculation also needs to be sensitive to the desired power for a study. It is possible for researchers not to reject the null hypothesis when they should (incorrectly concluding that there is no difference when one actually exists). This is termed type II error , denoted by β. Power refers to the likelihood of avoiding a type II error, equal to 1 − β. A study with 80% power to detect a difference between treatment groups will incorrectly reject the null hypothesis (i.e., observe no difference when one truly exists) 20% of the time, or in 1 in 5 studies. Depending on the question under consideration, this conventional threshold may be too high, but designing a higher-powered study will require enrolling more patients.
Sample size calculations identify how many patients must be enrolled to estimate the treatment effect with appropriate α and β boundaries. Calculations are based on the expected outcome frequency in the control group, what difference in outcome the treatment group should experience, and what type I and type II errors the investigators find acceptable. eFig. 11.1 provides an example of how sample size, effect size, number, and α thresholds influence study power. Sometimes, external constraints—such as cost, time, number of eligible patients, and consent rates—may limit the pool of available study subjects, leading investigators to modify study assumptions, such as increasing the expected difference in outcome in the treatment group. However, such modifications make it harder to identify a smaller, but important, treatment effect. Clinicians need to know when negative results are due to an underpowered study, as such studies are not necessarily evidence that a treatment has no effect.

Randomization
Randomization is an essential feature of clinical trial design. The outcome of a study subject is affected by factors other than the study intervention, which can obscure the effect of the intervention on the outcome. Confounding classically refers to factors associated both with the exposure and the outcome outside of the causal pathway. For example, one might observe that duration of antibiotic therapy is associated with longer pediatric intensive care unit (PICU) length of stay (LOS). However, both are affected by the presence of a positive blood culture. By adjusting for presence of a positive blood culture, a less biased estimate of the true association between antibiotic duration and PICU LOS can be calculated. Statistical analyses, such as stratification or multivariable modeling, can adjust for known confounding factors after a nonrandomized trial, but nothing can be done about unknown confounding factors. Instead, if subjects are assigned to treatment groups by chance alone, both known and unknown confounding factors should be balanced across groups. These factors can no longer be associated with the treatment group assignment (which was made randomly) and will therefore no longer confound the results.
In a randomized trial it is essential that study staff be unable to determine or guess which treatment group a particular patient might be enrolled into. For example, if a provider believes that an intervention is likely to be effective and is able to determine treatment group assignment in advance, that provider may try to enroll a sicker patient when the sicker patient is likely to be assigned to the treatment group. This selection bias will affect the study results because severity of illness will now be unbalanced between groups. Methods of randomization range from simple (flipping a coin) to complex (centralized, computer-generated randomized lists). Block randomization restricts the randomization process so that an equal number of group allocations is ensured within “blocks,” or small groups of serially enrolled patients, such as those at a study site in a multicenter trial. This ensures balanced numbers of patients in each treatment arm within each block.
Despite randomization, a study could still have imbalance in crucial factors between study groups by chance. Stratification at randomization can help control unwanted variation, which is particularly important in smaller studies. In the PARDS example, if children under 2 years old with PARDS have twice the mortality of children over the age of 2 years, then the investigators would need to ensure that an equal number of children under age 2 are enrolled in each study arm. To accomplish this, investigators can randomize within strata of age so that equal numbers of patients within each age group are randomized to the treatment versus control groups. A combination of these two practices—stratified block randomization—is the most common randomization practice in RCTs.
Blinding
Blinding indicates that individuals involved in a trial—clinical providers, investigators, and enrolled patients—cannot determine which treatment group study subjects are in. Blinding minimizes the chance that the trial results will be influenced by a change in behavior by providers or subjects based on their knowledge (and opinions) of the intervention. Single-blind studies are composed of subjects who do not know their treatment group assignment. Double-blind studies conceal treatment group assignment from both enrolled patients and those caring for them. It is crucial to blind study staff who determine any subjective outcomes of subjects in order to reduce observer bias, also known as ascertainment bias . Concealing treatment group assignment may not always be feasible, such as in trials of extracorporeal life support. If an intervention is highly effective, it is also sometimes possible for clinicians to guess which patients are receiving the intervention. Some efforts to blind participants to treatment group allocation, such as using sham surgical procedures to compare to a surgical treatment arm, may subject patients to additional risk. Special ethical considerations apply in these cases. ,
Outcome selection
Multiple aspects of study design, including sample size calculations, the timing of study observations, and statistical analysis, are dependent on the choice of the primary outcome. Since it is so influential in study design, a study should have only one primary outcome, although many studies also evaluate additional (secondary) outcomes that are observed alongside the primary outcome but do not influence study design. Each outcome measure has particular strengths and weaknesses; which measure is most appropriate for a particular study depends on the question being asked.
Mortality
Reporting death is accurate, with indisputable clinical relevance. However, using mortality as a primary outcome is not entirely straightforward. Many studies attempt to define deaths as related or unrelated to the critical illness, but ascribing death to a particular illness can be highly subjective. Mortality is sometimes too infrequent, as it is in critically ill children, to be a feasible outcome for RCTs.
Two traditional measures of mortality are hospital discharge status and mortality at a fixed time point (e.g., day 28). Status at hospital discharge can be difficult to interpret, as local practice at a study site (e.g., a preference for early discharge to a nearby rehabilitation hospital) may influence discharge timing and in-hospital mortality. Mortality at a fixed time point avoids this difficulty but may fail to capture the entire risk period of the illness. For example, a patient with severe sepsis may remain critically ill on life support at day 28. This patient’s outcome for study purposes is survival, although this is meaningless if the patient dies shortly thereafter. The risk of mortality can persist for months and remains high for up to 3 years among children following critical illness. Failure to capture delayed illness-associated mortality may falsely support therapies that delay, but do not prevent, death.
Morbidity
Intensive care may save a life only to produce a survivor wracked by infirmity, with low quality of life (QOL) and high reliance on healthcare. Morbidity can be considered in terms of physiologic change in organ function, effects on resource use (e.g., hospital costs, hospital readmission, cost of home nursing), functional status, or quality of life. , ,
Organ dysfunction
Organ dysfunction (or organ failure) scores are relatively easily quantified. These scores can describe the severity of a patient’s acute illness, indicate the need for hospital resources, and predict risk of in-hospital death. Many organ dysfunction scoring systems exist. , , The pediatric logistic organ dysfunction (PeLOD) score was the first score developed and validated to predict hospital mortality in critically ill children. Subsequently, multiple organ dysfunction syndrome (MODS), pediatric MODS, and pediatric sequential organ failure assessment (p-SOFA) scores have also been associated with in-hospital outcomes for critically ill children. , However, the extent to which timing, severity, and the differential effects of different organs failing influence long-term outcome remains poorly understood.
Resource use
Resource use, including measures such as duration of mechanical ventilation and ICU LOS, captures information related to the severity of organ dysfunction and critical illness. Analysis of studies with resource use as the primary outcome require special handling of mortality and other competing risks to ongoing resource use, as patients who die during a study use no additional resources and accrue no additional costs, but this can hardly be considered a positive outcome.
Functional status
Research tools that quantify functional status have supported the expansion of critical care outcomes research beyond mortality and the hospital setting. The most commonly employed tools are well validated, easily administered, and suitable for retrospective collection in large populations. Examples include the Pediatric Overall Performance Category, the Pediatric Cerebral Performance Category, and the Functional Status Scale. On a large scale, approximately 5% of children surviving critical illness experience new functional morbidity, although this risk varies by age, comorbidity, and diagnosis.
Quality of life
QOL blends survival with the perceived value of survival. Along with functional status, QOL ranks highest on patient-nominated outcomes of interest. QOL measures are being increasingly used in studies of critical illness, , including two large pediatric critical care RCTs in progress (Stress Hydrocortisone in Pediatric Septic Shock #NCT03401398; Prone and Oscillation Pediatric Clinical Trial #NCT03896763; both at clinicaltrials.gov). It can be difficult to ascertain whether a decrease in QOL is due to critical illness as opposed to being the result of an underlying disease (although paired assessments in relation to a baseline address this challenge), and QOL can be measured only in survivors. However, large RCTs incorporating QOL outcomes can be quite powerful, as underlying disease is usually balanced between treatment groups. Well-validated tools allow comparison with healthy population norms and with other ill populations.
Composite end points
Composite end points combine multiple different clinical outcomes into one outcome measure. This increases the event rate, which can reduce the sample size needed to see an effect. However, careful selection is needed to ensure that the combined end point is meaningful. Combined outcomes can be difficult to interpret, as they may combine differently valued outcomes (e.g., combined death and neurologic disability at 28 days) that individually occur with different frequency across the study groups. The least important outcomes usually have the highest event rate; unfortunately, such studies may be underpowered to identify a difference in the individual outcomes considered most important by patients, families, and clinicians.
Outcome-free time
To address difficulties in interpreting duration-based clinical outcomes in which mortality does not contribute additional days but is clearly undesirable, many investigators have turned to a composite outcome of outcome-free time. Unfortunately, very different individual outcomes can yield identical outcome-free time. For example, a study group with 10% mortality and median 14 days ventilation among survivors and a study group with 40% mortality and median 5 days ventilation among survivors both have a median of 10 ventilator-free days within a 28-day period. If these were trial results, we would conclude that there was no difference between groups, although patients and providers would likely disagree that these outcomes are the same. At a minimum, simple statistical tests are inadequate to evaluate this type of data; more sophisticated methods are required.
Surrogate end points
A surrogate outcome is an alternative outcome, often a biomarker, used to stand in for a clinical primary outcome of interest. The word biomarker was first used in research in 1973 to describe extraterrestrial biological markers , ; medical research adopted this term over time. The US Food and Drug Administration (FDA) began accepting biomarkers as surrogate primary end points in clinical trials in 1992, primarily to speed development of antiretrovirals to combat HIV/AIDS. Surrogate outcomes are attractive in medical research, as they are often more easily measured, cost less, or occur sooner than the ideal clinical outcome.
However, concerns persist about the validity and interpretation of surrogate end points. To be valid, surrogates must have a well-established, strong, independent association with the relevant clinical outcome in observational studies, with a plausible biological relationship. Then an intervention with an effect on the surrogate marker needs to be consistently associated with a simultaneous effect on the relevant clinical outcome in clinical trials. Difficulties with interpretation stem from the leap that clinicians must make between an effect on the surrogate to the relevant clinical outcomes. A statistically significant reduction in low-density lipoprotein serum cholesterol, for example, may not have any impact on clinical outcomes if the magnitude of reduction is too small or if the biomarker-based study did not last long enough to identify important side effects that would influence long-term adherence and safety. Studies using surrogate measures as the primary outcome must be considered in the context of medical knowledge surrounding that surrogate measure as it relates to actual patient outcomes.
Common trial designs
The simplest structure for an RCT involves a single intervention arm compared with a single control arm. However, investigators often wish to evaluate several possible interventions. A multiarmed study is possible but would require adding a study arm’s worth of patients for each intervention. The factorial trial design alleviates this problem. In a 2 × 2 factorial trial, an equal number of patients are allocated to four groups: control, intervention A, intervention B, and intervention A + B. This allows for patients in both the intervention A and A + B groups to be evaluated for the effect of intervention A, which can reduce total study size if there is no interaction between the effect of A and B. This design can also allow for evaluation of the interaction between A and B, although powering the study to evaluate for an interaction requires a larger sample size. ,
For some conditions and indications, it is challenging to recruit enough patients to carry out a standard randomized trial design while maintaining balance in other clinically important factors between study groups. A crossover trial may alleviate this problem. This within-subject study design exposes all patients to placebo and to intervention. Patients are randomized to the order in which they are exposed, with a washout period in between. Since all patients receive both treatment options, the number needed is reduced; all patients are assumed to function as their own controls. However, the washout period must be sufficiently long and the effect of the intervention must happen soon enough for it to be observed during the treatment period. The order of receiving treatment may have an effect on the outcome and should be included in the analysis. For example, if the intervention is effective but takes longer than anticipated, the effect could appear during the placebo period for the group that received the treatment first.
As new medications are developed and tested against previously approved medications, sometimes the question changes from “is the treatment better than the control?” to “is the treatment as good as the control?” This is especially important if the new treatment offers other benefits, such as lower cost, more favorable pharmacokinetics, or fewer side effects. Noninferiority trials approach study design with a different null hypothesis—namely, that the new treatment is worse than the old treatment (often referred to as an active control ). Rejecting the null hypothesis requires that the estimate of effect and the entire 95% confidence interval do not cross a predetermined threshold of “worse.” These studies must be powered sufficiently to achieve a narrow confidence interval to appropriately identify noninferiority when present. Additionally, a lower than anticipated outcome rate may make it inappropriately easy for a new treatment to be deemed noninferior. Noninferiority trials require a unique approach to statistical analysis and reporting.
Phases of clinical trials for new drug approval
When a drug, procedure, or treatment appears safe and effective in laboratory and preclinical experiments, investigators proceed with evaluation in human trials. Clinical trials for human drug development in the United States are classified into four phases by the FDA. In each progressive phase, the number of patients, complexity, duration, and costs of the trial increase. The complete process is long; the average interval from drug development to market is approximately 10 years. The longest portion of this interval is usually drug testing in clinical trials, which collectively occur over 5 to 7 years.
Phase I trials are dosing trials, in which a small number of human subjects receive several doses of the study drug to assess its pharmacokinetics, pharmacodynamics, and side effects in either healthy volunteers or patients with terminal conditions with few remaining treatment options. A phase II trial evaluates drug efficacy, usually in the patient population of ultimate interest, while continuing to monitor safety and side effects in more subjects over a longer period. Finally, phase III trials are rigorously designed RCTs, with strictly defined outcomes or clinical end points, enrolling hundreds to thousands of patients across multiple centers. Phase IV trials are postmarket trials conducted following drug approval by the FDA. These studies, in addition to consumer and clinician reporting of drug-associated safety concerns, allow for ongoing evaluation of rare adverse events associated with the drug and evaluation in new populations.
Statistical analysis and reporting
The results of research studies are judged by their reliability and validity. A trial is reliable if it is repeated under the same circumstances and the same results are achieved. A study has internal validity if the results are real and not due to bias, chance, or confounding; it has external validity if its results can be generalized to a broader population.
Whom to analyze?
All randomized studies should be analyzed based on original group assignment, or intention to treat . By including all patients randomized into each group—by the treatment that they were intended to receive—all consequences of the treatment and all benefits of balance achieved by randomization are preserved. It can be tempting to analyze based on actual receipt or completion of treatment, termed per-protocol analysis , which excludes patients who crossed over between treatment groups or excludes patients after randomization for other reasons. While these analyses will reduce dilution and possibly identify a greater treatment effect, they will also lose the benefits of randomization and will introduce selection bias in the result. However, the most appropriate approach to analysis depends on the trial. Pragmatic trials , which aim to test the real-world performance of an intervention in a broad population of patients in a clinical setting, differ from explanatory trials , which aim to identify the biological effect of an intervention in a more idealized setting. In pragmatic trials in which loss to follow-up and incomplete adherence are an expected part of the intervention, per-protocol analysis may help translate trial results to alternate clinical settings in which differences in adherence, demographics, and other factors may substantially influence the effect of the intervention.
Subgroup analyses focus on the effects of a treatment within a particular group of study participants, such as women, those within a given age strata, or among members of a specific race. These analyses are typically performed when there is a suspicion based on observational data or biology that the treatment effects may differ among groups, also known as effect modification or interaction . Since these analyses involve smaller numbers compared with the whole trial, they are typically underpowered to definitively identify treatment effects. Also, performing multiple additional statistical tests increases the likelihood of a type I error or identifying an effect by chance simply because so many tests were done. For these reasons, subgroup analyses should be prespecified and limited in number.
Hypothesis testing and determining the study result
Inference and estimate of effect
A clinical trial observes the effect of an intervention in a small sample of patients; however, researchers want to generalize these results to the entire (theoretical) population. Statistical analysis allows this generalization to be made. Based on the study design and distribution of study measurements, researchers choose an appropriate statistical test to compare the study results against the null hypothesis.
For binary outcome measures (e.g., mortality), the estimate of effect is usually expressed as a relative risk or a risk ratio : the proportion of subjects with the outcome in one group divided by the proportion of subjects with the outcome in the second group. Alternatively, relative odds or odds ratio may be reported. Odds are calculated as the ratio of number of events to nonevents, and the odds ratio is the odds of the event in one group divided by the odds of the event in the second group. For rare events, the odds ratio will approximate the relative risk. The odds ratio is amenable to mathematical operations and can be generated from logistic regression analyses, which can include adjustment for confounding factors in calculating the estimate of effect.
95% confidence interval
Next, the authors consider the certainty of the estimate of effect observed in the study. The 95% confidence interval (CI) describes the range of true effect values that would plausibly yield the observed effect in the study. If the estimate of effect in a study is a relative risk reduction of 50%, then a 95% CI of 40% to 60% indicates that it would reasonably have obtained this study result if the true effect was anywhere from 40% to 60%. A higher-powered study will usually achieve a narrower 95% CI, giving more certainty about the true magnitude of effect. When comparing binary outcomes, a CI that crosses 100% (or 1.0) for odds ratio or relative risk ratio translates to no statistically significant difference between groups. Similarly, for continuous outcomes, a CI that crosses 0 (i.e., no risk difference) translates to no statistically significant difference.
P values
Statistical testing assesses whether the results support rejecting the null hypothesis within some margin of error. Researchers calculate the probability that the observed difference (or one greater) would have occurred by chance, if the null hypothesis were true—the P value . A low probability indicates that the results are unlikely to have occurred by chance and would support rejecting the null hypothesis. Notably, even study results with an imprecise estimate of effect (e.g., those with a very wide 95% CI, or wide range of true values that could be consistent with the observed results) can meet statistical significance (i.e., P < .05).
The method for calculating the P value varies by study design and outcome measure. Parametric tests assume that the outcome measure has a normal distribution, indicating that it can be fully described by its mean and standard deviation. For this kind of outcome, a t-test is used to compare means from one or two samples; the analysis of variance test is used to compare means from more than two samples. Nonparametric tests do not depend on a normal distribution, but because they make fewer assumptions about the distribution of the data, they are typically less powerful. These can be more appropriate for data that are highly skewed (e.g., length of stay, which is typically right skewed, as some patients have very long lengths of stay) or otherwise expected to have a nonnormal distribution. Nonparametric tests include the Wilcoxon rank sum or Mann-Whitney U test (for unpaired comparison of the median in two groups) and the Kruskal-Wallis test (for comparison of medians across multiple groups).
Different tests are used for categorical data (e.g., ethnicity, gender, pediatric operational performance category score). The chi square test compares observed to expected values in a 2 × 2 table. Fisher’s exact test is similar but calculates a more accurate P value when small cell numbers are present. McNemar’s test is used for paired categorical data.
For time-to-event data, survival curves are often used to display data. This allows investigators to handle varying times of observation prior to events, as well as partial data from patients who are observed for some time but not observed to have an event during follow-up (censored data). A hazard ratio is typically reported for survival data, describing the ratio of “hazard” rate (moment-to-moment outcome or event rates) between groups.
Additional sources and mitigation of bias
Bias results in differences between study populations that are not due to chance. Some features of design that reduce or eliminate bias, including study population selection, randomization, and blinding, have already been discussed. Some additional features of study analysis can further reduce bias.
Bias due to loss of data occurs when data from subjects are eliminated from the final analyses. Protocol violations, postrandomization exclusion, or unequal dropout or loss of follow-up can all result in missing data. Data that are unequally missing between groups, or missing not at random, can introduce bias. For example, if the study treatment was poorly tolerated by a subgroup of the study population, then those patients might be more likely to drop out, and their data would be missing from the final study results. The total amount of missing data can also impact study results. One review of 71 major RCTs in top-tier medical journals identified that 13 of the trials (18%) were missing outcome data on 20% or more of enrolled subjects. Imputation, sensitivity analyses, and other advanced statistical methods can be used to explore how much the results might be affected by missing data.
Additional methods of exploring study results
A clear distinction should be made between relative risk reduction and absolute risk reduction in study results. A reduction in mortality from 60% to 20% and a reduction from 3% to 1% both represent a relative risk reduction—1 minus the risk ratio—of 66%. However, the absolute risk reduction, or the difference in risk between groups, is substantially different: 40% versus 2%. The number needed to treat (NNT) is a related statistic that describes what number of patients would have to be exposed to the intervention to result in one “saved” outcome, equal to 1 divided by absolute risk reduction. In the examples above, the NNT would be only 2.5 patients for the intervention that reduces mortality from 60% to 20% but would be 50 patients for the intervention that reduces mortality from 3% to 1%. The NNT can facilitate comparing results from different studies and consideration of side effects, costs, and other aspects of an intervention. It can also be adjusted for a particular patient’s baseline risk compared with the average risk of patients in the study; among patients with twice the baseline risk of the outcome, the NNT would be cut in half. These terms and additional common calculations are summarized in eTables 11.1 and 11.2 .
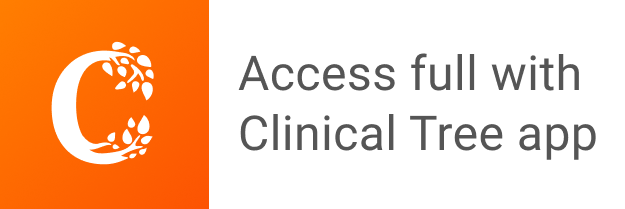