Abstract
Background
Recently, we used data from Epic’s Rover Transport application to analyze patient discharges from a phase I post-anesthesia care unit (PACU). We perform a retrospective cohort study to analyze how and when to use transporter log data to appropriately balance Type I and Type II error rates in the context of PACU transporter staffing decision-making.
Methods
The Rover Transport app was used to track all PACU transports from July 2022 through April 2024, totaling 22,846 across 461 workdays. The total hours of PACU transport follow a trapezoidal pattern, with load increasing through the morning (08:00–11:59), plateauing in the afternoon and evening (12:00–15:59 and 16:00–19:59), and then decreasing at night (20:00–23:59). Each transporter spends approximately one hour per four-hour period transporting. To inform transporter staffing decisions from these data, pairwise comparisons were generated between each workday’s “light” periods (08:00–11:59 vs 20:00–23:59) and “busy” periods (12:00–15:59 vs 16:00–19:59). The probability distribution of these pairwise comparisons were compared with normal distributions using Shapiro-Wilk tests and standardized normal probability plots. Then, for repeated statistical power analyses to guide PACU transporter staffing, Type II errors were considered at least as costly as Type I errors. Setting α = β = 0.05, we determined how many days of data were required to differentiate between the “light” periods (08:00–11:59 vs 20:00–23:59) and the “busy” periods (12:00–15:59 vs 16:00–19:59), using the minimum actionable difference of one hour per four-hour period.
Results
Both pairwise comparisons were normally distributed (Shapiro-Wilk W > 0.99). At α=β=0.05, proper differentiation of hours of PACU transport workload between four-hour periods required total transport data from at least 18 out of every 100 workdays for the “light” four-hour periods, or 44 out of every 100 workdays for the “busy” four-hour periods. Relaxing the combined error rate to 0.15 reduced the day requirements to 36 and 15. Restricting the combined error rate to 0.02 required 80 and 32 days of data for comparison between “busy” and “light” four-hour periods respectively.
Conclusions
The number of days of data needed for statistically powerful comparisons between four-hour period workloads are prohibitively large for manual collection. Therefore, hospitals not yet using the transport tracking capabilities in their electronic medical records for PACU transports will benefit from using them, even if only for the improved staffing decisions the data allows.
1
Introduction
Recently we studied a phase I post-anesthesia care unit (PACU) that implemented Epic’s Rover Transport application for its transporters. The availability of detailed data on transport times of PACU patient discharge allowed for improved decision-making about PACU transporter utilization and staffing. Transport metrics varied substantially by four-hour period of the day rather than weekday. In addition, prediction of transport workload using the count of transports for each combination of day and four-hour period multiplied by the overall mean time per transport differed substantively from actual total transport times per four-hour period per workday. This rendered the count of PACU discharges by time of day insufficient for high-quality transporter staffing decisions. As such, we previously recommended planning transporter staffing based on the mean and standard deviation of the workday’s total transport times during each four-hour period rather than the count of transports (i.e., PACU discharges). While the previous article considered how to choose a suitable number of transporters for each four-hour period, that presupposed a hospital had already implemented a digital transport tracking application such as Epic’s Rover Transport application for PACU-specific transports. In the current study, we evaluate the practicality of collecting transport time data intermittently (e.g., 1 day every 2 weeks) as a substitute for an always-on system able to automatically collect all transport data in its entirety.
PACU trends within a workday follow a consistent trapezoidal pattern with a steady increase in the morning, afternoon and evening plateau, and a steady decrease at night. Therefore, the middle of the day can be called “busy” whereas the morning and evening can be called “light” with a high degree of generalizability. Consider, for example, transporters working shifts in multiples of four hours. If a hospital has one transporter shift daily for four hours, then a decision is necessary. The transporter should work in one of the busiest two periods, likely either period 2 (12:00–15:59) or period 3 (16:00–19:59). If a hospital has two transporter shifts for four-hours (equivalent to one person working eight hours), no decision is necessary. Without analyses it is self-evident that there should be one assigned to each of the busiest two four-hour periods, likely one to period 2 (12:00–15:59) and one to period 3 (16:00–19:59). If a hospital has three transporters each working one four-hour shift, another decision is necessary. There should still be a transporter shift assigned to each of the busiest two periods, but then the third shift can be assigned to a “light” period, either period 1 (08:00–11:59) or period 4 (20:00–23:59). If a hospital has four transporter shifts, no decision analysis is necessary. One transporter shift should be assigned to each period. Yet, if a hospital has five transporters, one should be assigned to each period and then a second should be assigned to one of the busiest two periods, necessitating a decision analysis. This decision cycle repeats in order as successive shifts are added to the workday.
Based on the above logic, we focused our investigation on differentiating between the two periods that are the busiest (12:00–15:59 and 16:00–19:59), and the two periods likely to be the lightest (08:00–11:59 and 20:00–23:59). Our primary goal was to establish whether necessary sample sizes would be feasible for manual data collection (e.g., tracking transport data by hand one day every two weeks), or if the amount of data required would necessitate an automated system to record all transports. By doing so, we determine the economic value of using the electronic medical record system for transporters, if for no reason other than to collect the data needed to make well-informed staffing decisions.
2
Methods
This was a secondary study done on data extracted as part of previous research, which was determined by the University of Iowa Institutional Review Board to be a workflow assessment aimed at process improvement and so did not meet the regulatory definition of human subject research (#202311501, 28 November 2023). No patient data was involved. The manuscript was prepared using the Strengthening the Reporting of Observational Studies in Epidemiology (STROBE) checklist. All analyses were performed stepwise, using Stata 18 (Stata 18.0 BE, StataCorp LLC, College Station TX). All Stata code and associated outputs are in the Supplemental file, sequenced to match the Methods and Results sections. Interested readers would benefit from following along in the supplemental Stata output as they read the paper.
We extracted all PACU transport records between 1 July 2022 and 30 April 2024, when analysis began. That interval covered 670 calendar days, >4 times the period necessary for reliable PACU data. Epic’s self-service SlicerDicer tool was used for data extraction from the Rover Transport application system (Epic Systems, Verona WI). Detailed instructions on the extraction process (e.g., for other hospitals to use) are in the Supplemental Section E of Cover, Dexter, & El-Hattab (2025). The data flowchart with detailed consideration of the exclusions made can be found in Fig. 1 and Supplemental Section C of the earlier article. Briefly, system tests, transports that were cancelled or reassigned, transports longer than 60 min of travel time, and weekends were removed from the dataset. We studied 22,846 transport entries among 461 workdays of data.

Our previous work identified a large effect of four-hour period on transport workload, but no effect of weekday on transport workload. That finding matched our references, all of which found a similar impact of time of day. Therefore, we used the same four-hour period divisions as before. Each workday was divided into 4 successive four-hour periods: 08:00–11:59, 12:00–15:59, 16:00–19:59, and 20:00–23:59.
For each four-hour period of each workday, the total hours of transport time for all transports starting within those four hours were calculated. As explained in the Introduction, decision-making depends on the pairwise differences of hours of transport time among four-hour periods. The pairwise differences of total hours of transport time were generated for the two comparisons of interest (the “busy” periods of 12:00–15:59 vs 16:00–19:59, and the “light” periods of 08:00–11:59 vs 20:00–23:59). The Shapiro-Wilk test of normality was used to assess for normal distribution. Standardized normal probability plots were created to visualize the distributions of those two pairwise comparisons of interest (12:00–15:59 vs 16:00–19:59, and 08:00–11:59 vs 20:00–23:59). Because our sample size was large ( n = 461 workdays >> 50), we report the Shapiro-Wilk W-statistic as a unitless 0–1 measure of closeness to a normal distribution. With such a large sample size, the Shapiro-Wilk test of normality was statistically powerful, which allowed us to distinguish between W = 0.99 and W = 1.
Our primary goal was to evaluate whether sample size requirements would render the use of Epic’s Transport application or some similar tracking tool necessary for statistically sound staffing decisions. In performing a power analysis to determine sample size, the α, β, and minimum relevant difference must be decided beforehand. Because our earlier analysis determined that each PACU transporter spent roughly one hour per four-hour period on transports, a one-hour difference in total hours of transport load between four-hour periods would be enough to reasonably alter transporter staffing decisions. Thus, we used one hour as the minimum relevant difference for the analysis. The results of this power analysis matter economically because the one hour minimum relevant difference is considerably smaller than the 2.09 hour width of the 95 % prediction interval for the pairwise differences by combination of four-hour period and day between actual total transport times and transport times predicted from the number of PACU patient exits during the four-hour period; see Fig. 3 of our earlier article.
Statistical power analyses depends on balancing Type I (α) and Type II (β) error rates using values relevant to PACU operations management, a topic different from clinical trials. For purposes of PACU transporter staffing, a Type I error would be that we determine there is a staffing-relevant difference (≥1 hour of total transport time) between periods when one doesn’t exist. (The ≥1 hour of total transport time per four-hour period is from our earlier study of the data, showing this to be the average total transport time per transporter per four-hour period. ) Thus, the cost of a Type I error is the cost of the transporters’ wages that are “wasted” by incorrectly assigning them to a four-hour period where their labor is not effectively utilized. In keeping with statistical convention, we first set α = 0.05.
A Type II error would be that we find there is no staffing-relevant difference (≤ 1 hour) in periods when one does exist. Thus, a Type II error leads to a PACU that is constrained by transporter availability due to an inability to properly assign transporter coverage. The cost of a Type II error in this situation can be substantial from the resulting cost of the OR backups that accrue due to the improper assignment of transporter coverage. , , , As a result, a Type II error is at least as expensive as a Type I error. Thus, the combined error rate should be minimized rather than prioritizing either α or β. Proper weighing of these values requires precise knowledge about the costs of each type of error and their relative likelihoods. , However, when such specifics are unknown, setting α = β = (maximum tolerable error rate / 2) is sufficient. Therefore, consistent with past PACU operations management research, β = 0.05.
As a sensitivity analysis of our decision to set α = β = 0.05, we performed the same power analyses with α = 0.1 and β = 0.05, as well as with α = 0.05 and β = 0.1 To illustrate how the necessary sample sizes would change with even more restrictive errors, we performed one final set of power analyses with α = β = 0.01 (a combined error rate of 0.02).
The power analyses are for mean pairwise differences, and thus depend on the standard deviation of those pairwise differences. We used a finite sample, the most data that we could from the first consistent use of Epic’s Rover Transport application through when we started the project. To account for our finite sample size and thus the imprecision of the standard deviations used in the power analyses, we generated 95 % confidence intervals for the standard deviation of the pairwise differences. We then repeated the power analyses twice. The first repetition used the lower 95 % confidence limit of that standard deviation. The second repetition used the upper 95 % confidence limit of that standard deviation.
To determine what kind of differences could be detected using all available transport data (i.e., n = 100 days, as would only be possible with the implementation of an electronic transport system), we reran the analysis one final time. We set α = β = 0.05 throughout. Previous power analyses showed that a PACU should reevaluate staffing at least every 4 months. Ignoring weekends, this period (4 months) yields approximately 90–100 workdays of data. Thus, we fixed n = 100 (the maximum number of workdays in a four-month period). As in previous sections, the analysis was run in triplicate using the standard deviation point estimates and associated 95 % confidence intervals generated for the two pairwise differences of interest.
Each transport is ended in our data by the assigned PACU transporter pushing a “complete” button in their Rover Transport application when they return to the PACU. However, facilities that do not use Epic Rover Transport or a similar application, and instead try manual recording intermittently, may have documentation errors. Because the data collection would be identifiable by transporter, there may be a bias toward reporting longer transport times and thus each transporter appearing busier. Also, people often report times in rounded increments (e.g., more likely to enter 18:45 than 18:41). Therefore, as a simulation of human behavior, we rounded the total time of every transport in the dataset up to the nearest 15 min. We then reran the standard deviation calculations and associated power analyses.
3
Results
Both pairwise differences between hours worked in each four-hour period per day were normally distributed (both Shapiro-Wilk W > 0.99). Standardized normal probability plots of the two pairwise differences of interest (12:00–15:59 vs 16:00–19:59, and 08:00–11:59 vs 20:00–23:59), and accompanying histograms of those differences, confirmed this visually ( Fig. 1 , Fig. 2 ).
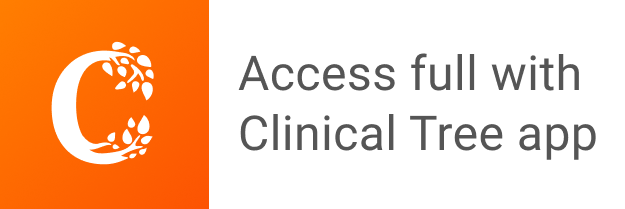