Fig. 5.1
Recovery as a continuous variable. X = individual patient change score, which is a measure of individual patient recovery as a continuous variable derived from summation of multiple domains
Defining recovery as a dichotomous variable enables analysis at the group and individual level, with some advantages. In dichotomous recovery, a patient is recovered if their postoperative score equates to or exceeds a threshold value (usually their pre-surgical value), either overall or in each domain. Recovery can be compared between groups (by comparison of the prevalence, and hence odds ratio, of recovery) or at the individual level. Importantly, it can assess recovery within differing domains. Unlike composite scores, which collapse all domains into one overall score, dichotomous scores have the ability to ‘drill down’ and identify in which (an how many) domains a patient failed to recover. It preserves the ability to assess recovery at four levels; overall recovery, within each domain to see where recovery has failed, within each subdomain to identify the particular problem (e.g. is the failure of functional recovery due to eating, dressing, standing or walking), and the severity of the problem indicated by the scores, or by the number of tests within each subdomain that fail to recover (Fig. 5.2), all of which are clinically relevant. This has implications in both the clinical and research setting, as it enables targeted clinical intervention or assessment of impact of intervention respectively.


Fig. 5.2
Recovery as a dichotomous score. This approach to domain analysis allows multiple levels of data interrogation to pinpoint the problem areas contributing to failed recovery, as well as allowing an indicator of severity of the problem.√ is recovered and X is not-recovered
Dichotomisation of a continuous variable must always be substantiated and none more so than with recovery. Recovery may be seen, as with many physiological variables, to be a continuum (i.e. 50 % recovered, 75 % recovered etc.). Thus, to maintain statistical rigor and minimise bias, it is argued that its data collection and statistical assessment should follow suit. In research, recovery data is often collected as a continuous variable which preserves data integrity and maximises internal validity and power with the lowest sample size [71]. Comparison of recovery between groups is by comparing the difference in mean change score between intervention and control groups in order to audit or research an intervention. However, the utility of recovery as a continuous variable for clinical decision-making is limited. Whilst recovery may be a continuum, the outcome of a clinical decision is binary – the patient has or has not recovered enough to finish the crossword or safely return to work (patient focus), or to be discharged (institution focus). A difference in mean change scores (derived from analysing continuous variables) does not directly indicate a tangible clinical effect, whilst recovery as a dichotomised outcome has direct clinical utility. When comparing recovery between two groups as a continuous variable, a difference in mean change scores may indicate a statistical difference in recovery between the two groups, but does not indicate whether this has clinical implications. By comparison, when comparing recovery between two groups as a dichotomous outcome, a difference in incidence or odds ratio of recovery has direct clinical consequences in terms of resource utilisation. In addition, the issue regarding the increased sample size resulting from dichotomisation is irrelevant in the clinical setting where the assessment is on an individual patient. This reinforces a limitation of translating research tools, with their definition of recovery as a continuous variable, into the clinical setting.
It is argued that by dichotomising a continuous variable, data richness is lost i.e. dichotomisation does not indicate the magnitude by which a patient may fail to recover but rather just that the postoperative threshold value for recovery has not been achieved. However, this limitation can be mitigated by the collection of raw data in its continuous form (where applicable) and subsequent secondary dichotomization for analysis. This enables analysis of a clinically relevant outcome (i.e. has this patient recovered or not), with secondary analysis of the raw data to determine the magnitude (i.e. by how much has the patient failed to recover?).
Dichotomisation of recovery requires a postoperative performance threshold to be defined, under which a patient is deemed to have failed to recover. The external validity of the recovery tool is thus limited by the duration in which this threshold is valid. However, by defining recovery as ‘a return to a patient’s own baseline or better’, this allows the recovery threshold definition to be independent upon future changes in nomenclature. This is because the patient’s postoperative recovery is derived using their own preoperative performance as a control for that specific postoperative event.
5.13.2 Objective vs. Subjective Testing
Although recovery has inherent implications to individual patients, it is essential that it be measured objectively to avoid undue influence of reduced postoperative patient insight [3, 38, 72, 73], impaired cognition or patient satisfaction. There is poor correlation between objective neurological impairment and self reported deficits [33, 64] and functional ability [63]. Although important in its own right, satisfaction is limited as a recovery assessment due to its lack of uniform definition, ease of measurement and rapid early recovery [74], with satisfaction rates of above 95 % [2] postoperatively reducing its discriminant utility. Satisfaction is also correlated more with patient expectation, patient experience and quality of therapeutic relationship [74] than with professional competence and anaesthetic outcome [74].
5.13.3 General Methodological Issues
The ideal recovery assessment tool is objective, provides both quantitative and qualitative data and is independent of bias. The introduction of baseline data and calculation of individual patient change scores addresses the initial differences between each group, thus reducing Type 1 error. A composite score allows quantification of the magnitude of recovery but collapses the multiple aspects of recovery into a single numerical value. By comparison, a dichotomised score allows assessment of recovery at the group, individual and sub domain level.
Both scoring types are susceptible to ceiling and floor effects. Ceiling bias results from an assessment question being answered uniformly independent upon actual recovery. Patient satisfaction and orientation rapidly uniformly recover [2, 38, 75] and thus are poor discriminators of recovery beyond the immediate postoperative recovery room. Ceiling bias may also result from inappropriate timing of testing. Floor bias occurred when patients respond poorly in baseline data such that it is mathematically impossible for them to achieve a ‘not recovered’ or ‘back to baseline’ score postoperatively. This occurs if the test is inappropriately difficult or administered prematurely in the recovery period.
Assessment of recovery over time requires tools to be resistant to learning effects, which can be addressed by subtracting the mean change score of a healthy population from that of the study population [64] or by introducing a tolerance factor [41].
There are specific methodological issues pertaining to assessment of cognitive recovery [57, 64, 70]. Measured cognitive performance is dependent upon the ability of the patient and the ease of the test. A patient with high cognitive ability may score above the population average on an easy test irrespective of whether there is any decline in cognitive function. Similarly, a patient with limited cognitive ability may fail to show an adequate performance (relative to a reference population) on a difficult test even when no cognitive decline is present. In addition, a tool that assesses cognitive recovery is biased when applied to a study population that has lower cognitive baseline scores than in the validation studies, as these patients are more likely to show increased recovery compared to the validation population. This exemplifies the importance of comparing a patient’s postoperative function with their individual preoperative baseline. Cognitive function scores were derived using age matched, healthy study populations without dementia. However, such study populations often exhibit a higher IQ, less cognitive deficits and a different learning effect and recovery time course than the general postoperative population [31, 64], thus reducing the external validity of the score [31].
5.14 The Future of Recovery and its Assessment: Patient Centred Care (PCC) and Real Time Recovery (RTR)
Patient Centred Care (PCC) has emphasised the importance of quantifying and optimising postoperative recovery in real time. PCC is defined as care that ‘is responsive to the preferences, needs and values of patients and consumers [76]. It is a concept that is foremost in healthcare worldwide, is advocated by the WHO and is associated with reduced mortality, length of stay, readmission and health-care cost. It is also associated with an improvement in functional status, patient satisfaction and real-time outcomes. PCC highlights the need for real time identification and management of patient’s with poor recovery and the corresponding need for routine qualitative and quantitative data collection and feedback.
Real-time recovery (RTR) is a concept ubiquitous in any arena that involves systems (i.e. business, computing) and is directly relevant to assessing a patient’s course of recovery. RTR is the ability of a system to recover from any error (human induced or infrastructure) in a time frame that has minimal impact. It thus requires first identification of a failure (i.e. poor recovery) and then secondarily implementation of an intervention known to counter this failure (i.e. implementation of an early intervention known to improve recovery). This highlights the difference between assessment of recovery in the research vs. clinical setting. Research recovery tools compare patient selection, anaesthetic and surgical technique and their impact on postoperative factors (i.e. pain management, function and discharge destination). It is retrospective and thus induces a time delay in the identification of poor recovery (and associated factors), which is prohibitive of early intervention for the original study patients. However, a recovery assessment tool that identifies RTR can serve both research and clinical functions. A RTR assessment tool analyses data as it is entered at clinically significant time-points and, through processes such as automated alerts, alerts the clinician at the time to patients that have sub-optimal recovery. Therapeutic interventions known to improve recovery can thus be implemented in a timely manner. Resources can thus be directed to those patients that would benefit most in a time frame that may have the most clinical impact, and in addition, resource use may be decreased in patients with good early recovery and facilitate fast-track care. RTR recovery tools also have research utility in that the databank can retrospectively assess recovery at the group level with respect to pre, intra and postoperative variables.
Integral to RTR assessment is the assumption that a patient’s recovery can be modified by early intervention. This requires not only identification of the presence of sub-optimal recovery but in which domain. Composite recovery scores are sensitive to the former, but not the latter. Multidimensional dichotomised assessment tools, however, allow a drill down of recovery assessment. They first identify poor recovery (a failure of performance in relation to a preoperative baseline) and then allow assessment of the domains involved and in which individual patients. This allows individual targeted intervention relevant to the clinical issue in an individual patient. For example, failure to recover due to persistent nausea requires different clinical interventions compared to cognitive decline. Postoperative recovery sub domains are interrelated (i.e. persistent pain may result in concomitant persistent nausea due to increased opiate use) but, by drilling down of recovery at the sub domain level, identification of the primary cause can target clinical intervention (i.e. use of non-opiate analgesia to reduce the persistent pain and subsequent nausea).
5.15 Conclusion
Defining the abstract ideals of quality and recovery is of paramount importance in perioperative medicine. Postoperative quality of recovery has developed from a unidimensional construct focused on restitution of physiological parameters, to one that is multidimensional and assesses recovery at both the individual patient and group level. Recovery can be assessed at clinically relevant postoperative time intervals, with early, intermediate and late recovery having both prognostic implications. The increasing awareness of the effects of surgical stress on the neurophysiology of an increasingly elderly ambulant surgical population highlights the need to include robust assessment of cognitive recovery. Recovery is ideally assessed as a dichotomous outcome overall and at a subdomain level, with real-time assessment at the individual and group level having both clinical and research utility.
References
1.
2.
3.
4.
5.
6.
Hogue SL, Reese PR, Colopy M, Fleisher LA, Tuman KJ, Twersky RS et al (2000) Assessing a tool to measure patient functional ability after outpatient surgery. Anesth Analg 91(1):97–106PubMed
7.
8.
Swan BA, Maislin G, Traber KB (1998) Symptom distress and functional status changes during the first seven days after ambulatory surgery. Anesth Analg 86(4):739–745PubMed
9.
Oakes CL, Ellington KJ, Oakes KJ, Olson RL, Neill KM, Vacchiano CA (2002) Assessment of postanesthesia short-term quality of life: a pilot study. AANA J 70(4):267–273PubMed
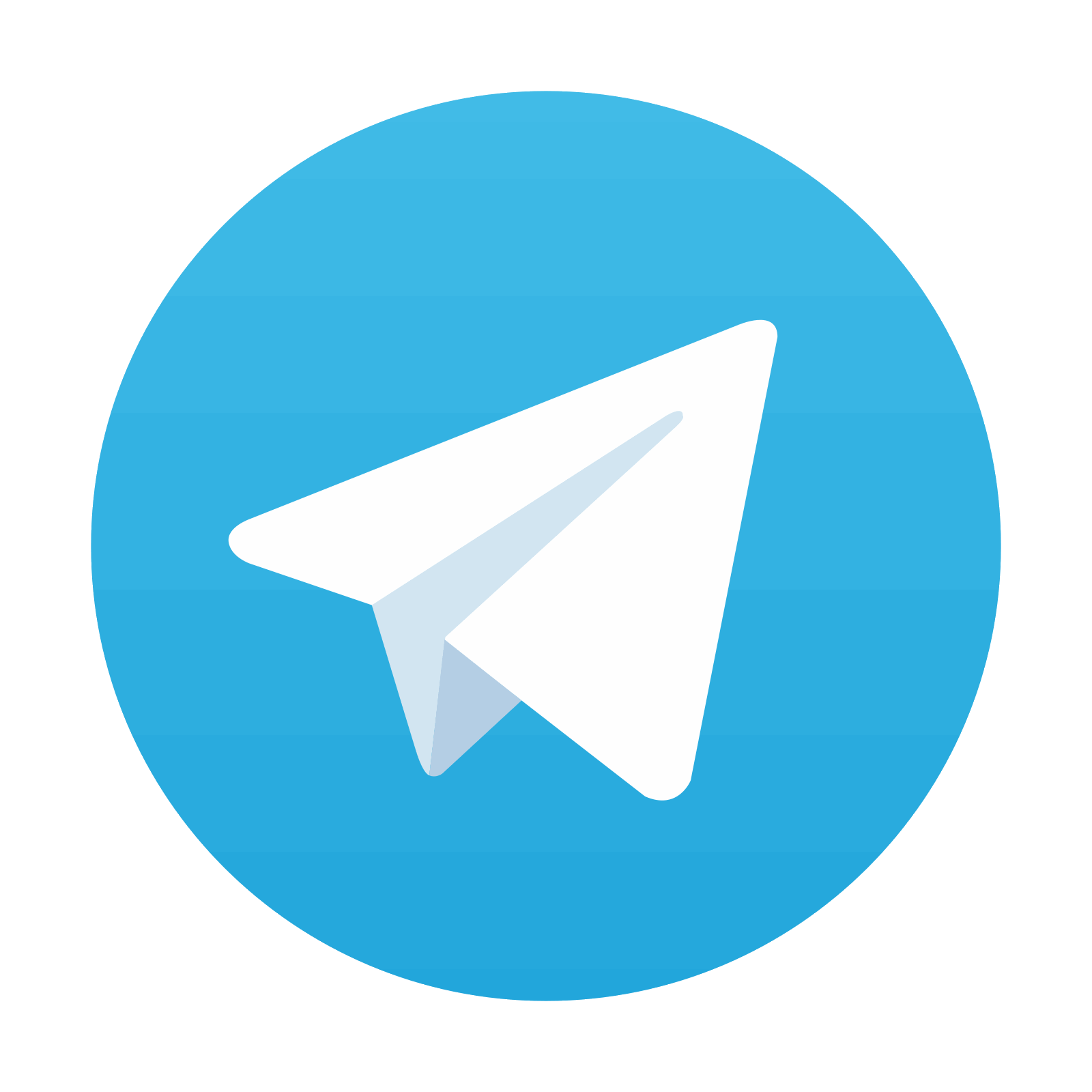
Stay updated, free articles. Join our Telegram channel

Full access? Get Clinical Tree
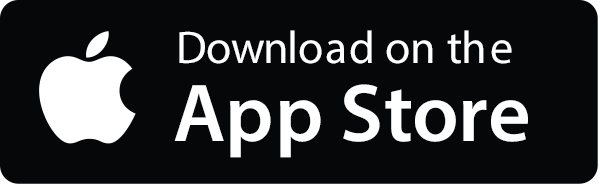
