Systems engineering embraces every scientific and technical concept known… . It is the job of integrating an entire problem to arrive at one overall answer, and the breaking down of this answer into defined units which are selected to function compatibly to achieve the specified objectives.
Introduction
Assuming the same introductory points as the previous chapter, where the most tested tools of Systems Engineering (SE) were presented, the aim of this chapter is to provide an overview of both methodological and practical aspects of the latest tools for SE in optimizing care processes, modeling and simulation, and radio frequency or image identification. Some possible uses will then be hypothesized in reference to geriatric surgery and finally, some keys will be offered to healthcare personnel for interacting with systems engineers, in the interest of both patients and society.
Systems Engineering: Substance and Methods
SE Definition: Historical and Philosophical Hints
SE is an interdisciplinary approach to process optimization that encompasses a wide spectrum of actions, from the correct formulation of problems to the development of adequate solutions and identification of the necessary resources. As a discipline, it is neither easy to date, nor well defined. A milestone was posed by a biologist (von Bertalanffy 1968), but the formal principles were founded, at the end of the nineteenth century, by mathematicians and physicists (Poincaré 1908, Boltzmann 1968), while conceptual foundations were expressed back in the times of Galileo and Descartes. Further developments were established in information theory (Wiener 1948, 1993) and other contributions date back to operational research used by English military planners during WW1. Modeling theory and practice completes the frame with user-friendly interfaces and methods (Gianni et al. 2014).
SE is a branch of organizational science that considers the roles played by the components of whatever organization (people, resources, space, money, environment, waste, etc.) in order to find the best way to deploy and manage them.
Healthcare professionals are not required to learn SE as a new specialty; they just need to know what tools are available, what they can obtain, including the scopes, by interacting with systems engineers.
How SE Operates: Methodological Keys
Process optimization is best achieved by designing a system with clear purposes and targets, ensuring that changes in any part of the organization will result in significant improvements in total system performance. SE is not one method per se, but rather a frame of mind, seeking specific pathways case by case, using specific tools, which will be sketched in the following, but using them in a framework of imagination, inspiration and vision. It is not by chance that NASA, the epitome of corporation technology, defines SE as the “art and science of developing systems etc.”, to emphasize its creative aspects (NASA 2006).
Operating tools
The number of tools pertaining to SE is huge. Beyond management systems (see previous chapter), other tools have been adapted to applications in healthcare delivery, to both improve individual organizations and to refine public systems: statistical process controls, queuing theory, failure-mode effects analysis, modeling and simulation, human-factors engineering, concurrent engineering, industrial engineering; while others that were already known, have also been applied (value engineering (Raftery and Powell 2013), total quality management (TQM; Andrew et al. 1995, Balasubramanian 2016), information management systems (IMS; Weaver et al. 2015), quality function deployment (QFD; Radharamanan and Godoy 1996, Chan and Wu 2001, Gremyr and Raharjo 2013), operation research (Sapula 2002, Malhotra and Zodpey 2011), cycle time analysis (Shortel 1998, Gilman et al. 2014), safety management (see Chapter 47), radio frequency identification (RFID; Yao et al. 2012, Kolokathi and Rallis 2013), and heat-tracing including asset location and management, etc.). Many of them, it should be observed, are parts of others or “variations on the theme”, overlapping each other, due to NIH (not invented here) syndrome. In the following paragraphs, two of the most advanced, original and promising tools are reported.
Modeling and simulation
Modeling and simulation (M&S) is the most advanced, sophisticated and powerful state-of-the-art tool, well-suited to provide professionals and top management with a way to evaluate their new proposed strategies and solutions, and experiment with them virtually before deciding on their implementation.
M&S is not new in healthcare: reviews of healthcare simulations can be found from the end of the seventies (England and Roberts 1978, Klein et al. 1993, Fone et al. 2003); approaches to relevant challenges (Jacobson et al. 2006, Brailsford et al. 2009), examples of applications in the USA (Kopach-Konrad et al. 2007) and case studies in Ireland, one of them specific to elderly care (Arisha et al. 2013) are found in more recent papers. Yih (2011) dedicated a whole chapter of her Handbook of Healthcare Delivery Systems to computer simulations. Actually, striving for a review of M&S in healthcare is “without doubt a Herculean task” (Brailsford et al. 2009).
Borrowed from other businesses, modeling is not specific to healthcare; however, models of care did indeed originate from modeling, even in its simplest and less technological forms (see Chapter 45), and the more complex the issue, as with geriatric surgery, the higher the benefits that are expected from M&S.
Modeling is performed by SE specialists, hence no specific ability is required from MDs in building and operating the models. To be “modern and up to date”, professionals are not called on to be engineering specialists. However, their contribution is essential to the goal: they are the “clients” of SE, and their role is to:
ask the relevant questions
sketch the basic organizational structure of new solutions they devise
procure and provide data to be fed into models
evaluate results of simulations (virtual computer-processed experiments, not to be confused with medical training simulations)
address the emerging issues
organize and implement the most convincing scenario into reality, establishing contingency plans for undetected failures
check and monitor chosen solutions.
With modeling and simulation, professionals will be able to “view” the bottlenecks in clinical pathways – instead of imagining or guessing them – and “fix” the possible drawbacks in implementing suggested solutions. Some examples in the following paragraph will show the power of M&S in re-engineering a healthcare facility, and what results are obtainable (Costantini and Bettelli 2005).
Radio-frequency Identification and Tracking (RFID)
RFIDs as Stand-alone Tools
RFID systems use tags or labels (transponders) attached to persons or objects in order for them to be identified and positionally tracked. An RFID system may be used as a stand-alone tool, with its own benefits. For instance, LCD monitors properly located can show at a glance, in real-time, the state of a healthcare unit (or of a hospital), by tracking:
patients (position, standing- or fallen-to-the-ground)
MDs and nurses
visitors
maintenance personnel
stretchers, wheelchairs, walking frames, beds, food carts
mobile medical facilities.
This has great pragmatic value per se, for instance checking the effective strategic allocation of emergency toolkit carts, finding the position of Rx movable equipment, or pointing to the locations of out-of-bed patients. RFIDs are not yet fully deployed and new technologies create newer opportunities; while RFIDs are still unmatched for asset tracking, webcam normal and/or thermal imagery is less costly, may be more efficient and can track elderly patients’ position and movements, detecting assumed decubitus, falls, wandering, abnormal toilet time and runaways, while privacy can be granted, keeping the imagery cached into the system until a specific alarm situation occurs, brought to the attention of the personnel. Such technologies would have prevented the real-life case of a missing patient, a cognitively impaired subject who left the hospital building, was painstakingly sought for days, and was found weeks later frozen to death in an uninspected skylight shaft.
RFIDs and Heat/Image-Tracing as Integrated Tools
These in fact are part of the family of automatic identification and data collection (AIDC) systems; an everyday example is the “Telepass’”device in the transportation business, the basis of a system of motorway toll reporting, accounting and billing. Tracing systems are an even more decisive tool when integrated into M&S, used not just to give real-time information to staff, but to gather, record and feed data to other systems.
Continuously updated information on patient locations, the length of time in that location, their waiting status or other and, if required, the waiting time through the whole process – as patients are admitted, moved throughout the facility and discharged – are saved to a database. It is interesting to note that since the eighties, an American National Standard Institution (ANSI) standard (HL7, now in version 3) has been available for electronic data exchange between systems in the clinical domain, designed to support both central patient care systems and distributed departmental systems.
As an example, HL7 supports the generation of a sequence of messages for post-medication instructions, laboratory requests, transfer and transportation orders, etc. This helps to decisively record care plans for each patient, evolving with patient stay and with possible readmissions; changing the habit of registering the information in separate clinical records, or different staff memorizing part of it is important. Explicit care plans were established through HL7 in the case studies in Irish hospitals reported below, with the declared intention to use them for modeling hospital processes, workload planning and quality improvement.
Examples of Applications
In the literature, examples of not-so-recent ongoing applications of these concepts and tools are found in the fields of therapeutic optimization (hemodialysis, radiation therapy) and patient flow modeling (Kopach-Konrad et al. 2007). Of these, flow modeling is clearly of specific interest for geriatric surgery.
Paradigmatically, the Irish case study of elderly patients (Arisha et al. 2013) aimed to optimize just the patient flow and find its criticalities; based on a user-friendly interface built through an Excel worksheet, the model was first validated, confirming proper compliance with the actual flow. Then, the impact of uncertainty on available capacity and on methods of LOS reduction for elderly patients in acute beds was investigated through the simulation of different solutions. In its conclusions, the study showed that by introducing intermediate postacute care beds, the system’s performance might be enhanced significantly, reducing delays and costs of hospital stays by almost 50%, and improving the discharge phase and related workflows. Situations of this kind happen frequently in hospitals, even more so in geriatric surgery. In general, today, operators navigate by sight to solve the issue, and they would be keen to see systematic criticalities removed.
The study also used the model of a cost/benefit analysis in implementing the proposed solution, and used statistical methods to detect the patient flow deadlock in the rehabilitation stage. Valid as this conclusion might also be for geriatric surgery units by logical inference, providing ready-made solutions is not the aim of this chapter. On the contrary, its gist is to stress the importance of building, developing and running specific models specialty by specialty, and then case by case, to give due regard to the specific conditions of structures for each country or region, such as:
the organization’s starting point
the micro- and macro-economic situation
patient type and status evolution
healthcare state of the art.
The interdisciplinary contribution of Arisha and his colleagues to geriatric medicine, and other studies applied to an emergency department in an adult teaching hospital in Ireland (Abo-Hamad and Arisha 2013) are evidence of how powerful and altogether feasible M&S are, in critical activities in healthcare.
Barriers to SE in Healthcare and Ways to Overcome Difficulties
Reviewing the already wide literature on SE in healthcare, and pondering the analyses by Reid et al. (2005), a few hindrances are easily spotted:
some (many?) staff are reluctant to explore new paths, particularly when this leads to significant changes in traditional relationships, overcoming a culture of functional compartmentalization and of a somewhat rigid division of tasks tasks (silo mentality, see Chapter 34), introducing new competences and work patterns transcending functional areas (Scott et al. 2015)
reimbursement practices and regulations provide small incentive for investing in quality and innovative systems, conversely favoring the status quo
decisive support of the top management can be hence difficult to obtain for systems improvement
engineering professionals often have little, if any, education in healthcare delivery, and seem not to contemplate the progressive aging of the population in terms of design
competition among healthcare facilities is not necessarily favorable, as the already excellent institutions try to stay as such, possibly lobbying against national initiatives and plans to generalize a quality increase in healthcare.
A quick empirical research on the internet shows that many MDs in management positions in the USA acquired an MBA at a certain point of their professional life (mostly later in their career), while in Europe this combined education is very seldom encountered for the same responsibility level. The scope to generate profit seems to be the divide in this subsidiary culture, in spite of the fact that delivering the best care at the lowest cost is the common goal of both profit and non-profit healthcare organizations.
On the other hand, other hindrances are objective: the case studies and examples considered in this chapter, beyond the advantages, explicitly state that the main challenges were:
irrelevant or insufficient data
data accuracy
lack of some types of data
consequent best-guess assumptions made by healthcare providers.
This should encourage systematic data recording and collection in the field of geriatric surgery, desegregated in a systemic view, as recommended in other parts of this book, possibly and hopefully organized into databases; model accuracy relies on solid, accurate and detailed input data.
Such difficulties in data gathering and validation are costly, of course, and are justified by the results obtained. Above we considered the usefulness of RFIDs integrated into SE as powerful “data seekers” and “data miners,” but – separately considered with no attention to global costs – it is itself a costly solution.
So, a peculiar paradox – a sort of “Catch 22” – may be observed: for SE to be used, there needs to be evidence of its advantages, but there can be no evidence unless SE is used. On the other hand, an important position paper mentions the “hypercomplexity” of healthcare systems, which “might have inhibited the use of modeling within the domain” (Klein and Young 2015).
The observation of a few current realities might hopefully overcome the obstacles and the paradox:
the perspectives shown in the previous chapter about a team approach, quality measures and assessment, and a systemic vision are starting to become part of the healthcare culture
the pressure of economic constraints and of damage litigations might trigger a more quality-related search for efficiency and efficacy, because knowledge costs less than inaction
the examples reported, and others that can today and will tomorrow be found in the literature might convince healthcare organizations and their professionals to welcome this upcoming trend more than incentives or recommendations.
References



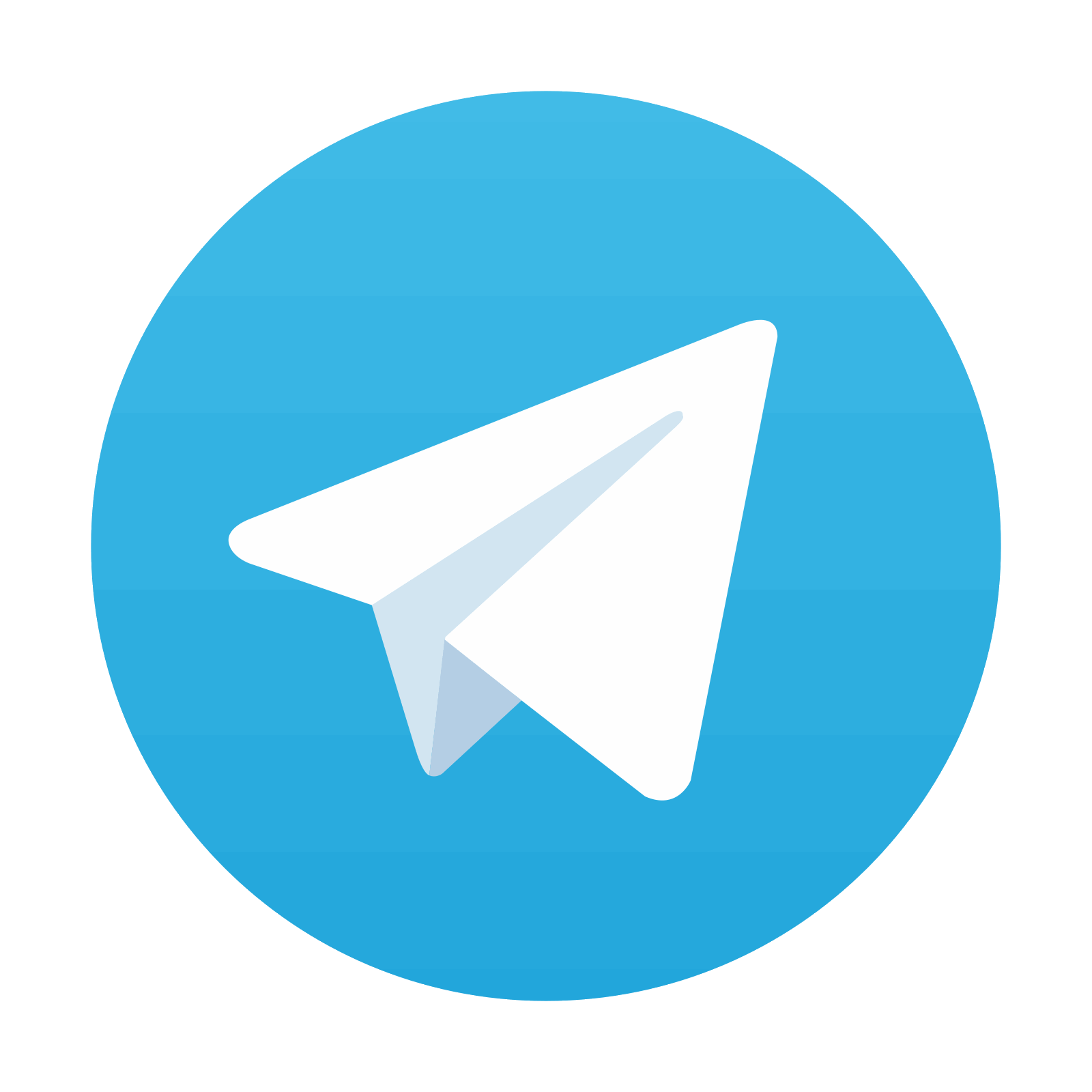
Stay updated, free articles. Join our Telegram channel

Full access? Get Clinical Tree
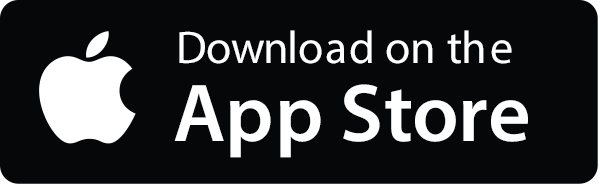

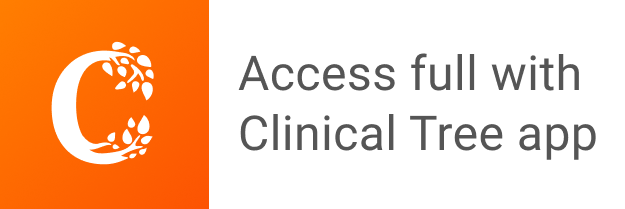