Abstract
This chapter provides a brief introduction about the basic principles that underlie study design, sample size, basic concepts of biostatistics, and data analysis for clinicians. The basic concepts of biostatistics are largely forgotten by clinicians by the time it is needed, and keeping this in mind, briefly, definition of biostatistics, introduction about the scales of measurements, principles of statistical inference, and need of probability distributions are given here. Study design and the sample size for each of the study designs are also explained. The appropriateness of statistical methods to be chosen for the analysis is eased with flowcharts.
Keywords
Biostatistics, Case-control study, Cohort study, Cross-sectional study, Data analysis, Designing studies, Interim analysis, Observational studies, Probability distributions, Randomized controlled trials, Sample size, Statistical inference
Outline
Introduction to Biostatistics 976
Definition of Statistics 976
Biostatistics and Its Applications 976
Uses of Statistical Methods in Medical Sciences 976
Some Basic Statistical Concepts 976
Population and Sample 977
Scale of Measurements 977
Constant 977
Variables 977
Parameter and Statistic 978
Ratio, Proportion, and Rate 978
Statistical Inference 979
Estimation 979
Hypothesis Testing 979
Steps in Hypothesis Testing or Testing the Statistical Significance 979
Defining the Null and Alternative Hypotheses 980
Calculating the Test Statistic 980
Obtaining, Using, and Interpreting the p -Value 980
Errors in Hypothesis Testing 980
The Possible Mistakes We Can Make 980
Other Important Concepts That Are Essential in Statistical Inference 981
Parametric and Nonparametric Statistical Methods 981
Basic Principles of Statistics 981
Probability Distributions 982
Study Design 982
Sample Size 985
Sample Size in Clinical Trials 985
Interim Analyses 985
Sample Size in Observational Studies 987
Cross-Sectional Studies 987
Case-Control Studies 987
Data Collection and Preparing Data for Analysis 987
Analysis and Presentation of Data 989
Summarizing Data 989
Comparing Groups: Continuous Data 989
Comparing Groups: Categorical Data 991
Comparing Groups: Time to Event Data 991
Relation Between Two Continuous Variables 992
Multivariable Analysis 994
Conclusion 995
References 995
Introduction to Biostatistics
Most of the researchers of medical sciences feel that mathematics in general and statistics in particular are an excessive and difficult task. In biomedical research, knowledge of statistics is mandatory and is an integral part of the research. In the era of evidence-based medicine, the practice of designing and conducting biomedical observations and experiments, presenting the data accruing therefrom, and interpreting the results, would be impossible without applying statistics.
Definition of Statistics
The word “statistics” is devised from the Greek words “status” meaning “state” or “position.” The Oxford dictionary defines statistics as “the study of the collection, analysis, interpretation, presentation, and organization of data.” Statistics can be defined both in plural and singular sense. In plural sense, it means “facts, expressed numerically or in figure, collected in a systematic way with a definite purpose in any field of study,” for example, according to the 2011 census, the population of India is 1.21 billion. In singular sense, it deals with the scientific treatment of data derived from individual subjects. The word statistics is used as the plural of the word “statistic,” which means a quantitative value like mean, median, standard deviation (SD), etc., derived from sample of subjects. For example, we select 15 individuals from a class of 100 patients, measure their body mass index (BMI), and find the average BMI. This average, a single numerical value, would be a statistic.
Biostatistics and Its Applications
The word “biostatistics” is combination of two words and two fields of study. The bio part contains biology and the study of living things, and the statistic part contains collection, analysis, and application of data. The use of statistical methods in analyzing data derived from medicine, biology, and public health is termed as “biostatistics.” The other popular names of this branch are biometry, medical statistics, and health statistics, and one can differentiate between them in the following manners:
Biometry : The analysis of biological data using statistical and mathematical procedures.
Medical statistics : Statistics/statistical methods related to clinical and laboratory parameters, their relationship, prediction after diagnosis/treatment, clinical trials, diagnostic analysis, etc.
Health statistics : Statistics/statistical methods related to the health of people in the community; epidemiology of disease, the association of demographic and socioeconomic variables, behavioral variables, environmental and nutritional factors with the occurrence of various disease, measurements of health indicators for a community, etc.
We researchers use statistics the way a drunkard uses a lamp post, more for support than illumination. Winifred Castle, a British statistician.
Biostatistics and biostatisticians are important partners and collaborators in health sciences. They have to be consulted right from the formulation of research question till submitting manuscript for publication.
Uses of Statistical Methods in Medical Sciences
Apart from collecting data scientifically and summarizing the collected data, biostatistics is used to test the hypotheses of research questions derived from all observational and experimental studies. Scientists or researchers combine biostatistics and probability theory for a given set of data to determine the likelihood of a disease to hit the target population. Therefore, statistical methods are as good at predicting the future as they are in analyzing the past.
Population and Sample
A population is a group of people for which we would like to investigate or make inferences, but it is simply not possible to study everyone with a specific medical condition of interest. The only choice is to select a sample, i.e., to study a subset of people selecting them at random from the population; for example, if we wish to investigate maternal weight gain in pregnancy and baby’s birth weight, we must study a sample of pregnant women. If the selected sample is random and large enough, we can make an inference about population without any snag.
Variables
In contrast, variables are properties or characteristics of study subjects that vary in quality or magnitude from person to person. To be a variable, a variable must vary (e.g., not be a constant), that is, it must take on different values, levels, intensities, or states, for example, age, sex, height, weight, blood pressure, cholesterol level, severity of injury, etc. The types of variables are given in Fig. 63.1 .

An understanding of variables is important to summarize them and to choose appropriate statistical methods to analyze them. Generally, there are two types of variables: categorical or qualitative and quantitative or measurable .
Categorical or qualitative variable : If the individual belongs to a particular group, class, or category, it is called a categorical variable, for example, sex (male, female), severity of disease (no disease, mild, moderate, and severe), etc. There are two types of categorical variables: ordinal and nominal . For ordinal variables the categories or groups are ordered in some way, for example, socioeconomic class (rich, middle, poor), degree of pain (no pain, mild, moderate, severe), etc. Nominal variables are those in which there is no possibility of ordering in classification, for example, sex (male and female), blood group (A, B, AB, and O), mortality (no, yes), etc.
A categorical variable is binary or dichotomous if there are only two categories, for example, yes/no, dead/alive.
Quantitative or measurable : A variable that takes some numerical value is called a quantitative or measurable variable. For example, age, height, weight, etc.
There are two types of quantitative variables: discrete and continuous . Discrete variable has distinct numerical value or certain whole numerical value. The number of patient visits during a particular out patient department or the number of children in a household are examples of discrete variables. Continuous variable has no limitation on the values that a variable can take; such variable can have decimal point values, e.g., weight or height.
Furthermore, there are two types of continuous variables. If there is no true zero point, then it is called “interval scale.” In this scale, the zero or starting point is arbitrary. Temperature is an example of interval type of continuous variable. In the case of “ratio scale,” the variable has a true zero point independent of the unit of measurement, e.g., weight or height.
These variables can be called in the following manner in epidemiological point of view while analyzing the data: outcome , exposure / risk factor , other factors (confounder, effect modifier, and intermediate variable). Outcome variable : a variable in which investigator is actually interested. It is also known as dependent, effect, or response variable. Exposure variable : a variable that is manipulated either by the researcher or by nature or circumstance. These variables are also called as stimulus, independent, covariate, factor, or predictor variables. Other factor ( s ): any factor(s) or variable(s) that has potential to influence the relationship between an outcome and exposure.
Parameter and Statistic
The value of a parameter is the function of population values, and it is related to the population. Statistic is the function of sample values, and it is related to the sample. For example, if the mean diastolic blood pressure (DBP) of the male population is 80 mmHg, it is the parametric value ( μ ), and if the DBP of a sample of males selected randomly from the population is 84 mmHg, it is the statistic value ( ˉx
x ¯
). We use statistic value to estimate the unknown population parameter. As sample size increase, the statistic value—obtained from the sample values—will be as close as possible to the unknown population parameter value.
Ratio, Proportion, and Rate
Ratio is obtained simply by dividing one value by another. In ratio, numerator is not a part of denominator. Examples are male/female or sex ratio, student/teacher ratio, and patient/doctor ratio.
Proportion is a type of ratio in which the numerator is a part of denominator, i.e., numerator is included in the denominator. For example, if there are 400 males and 600 females, then the proportion of males in the population is 40%. This is usually expressed in terms of percentage or in multiple of 10s such as 1000, 10,000 depending upon the number in the numerator with respect to the denominator.
Rate is a measure of the frequency with which an event occurs in a defined population in a defined time. In rate, a measure of time factor is an essential part of the denominator, whereas in a proportion it is does not, for example, number of deaths per 100,000 Asians in 1 year, number of perinatal deaths per 1000 births, etc.
Statistical analysis methods are of two types: descriptive method and inferential method, given in Fig. 63.2 .

Descriptive statistical methods are used to summarize the collected data using tables, diagrams, graphs, and certain statistics or summary measures such as averages (like mean, median etc.), and variation (SD, interquartile range, etc.).
Inferential statistical methods are used to make inferences about the population from which the sample was drawn. In this branch, the unknown population parameter(s) is (are) estimated using sample statistics (called estimates). Inferential statistics can further be divided into two subsections: estimation and hypothesis testing .
Statistical Inference
The main objective of the statistics, in this way or that way, is to study the population. Population in statistics is defined as aggregate of the objects having certain characteristics. The exact value of any characteristics of any population can be known when each and every member of the population is measured. However, as the population is very large, it is practically impossible to make measure on each and every member of the population. So, we draw a random sample from the given population, and as samples are comparatively very small in size, we can make measurements on each and every member of the sample. On the basis of these measurements, we estimate the value of the population characteristics. This is the main objective of the inferential statistics method. There are two types of inferential methods: estimation ( point estimate , interval estimate ) and hypothesis testing .
Estimation
It is the process of providing a numerical value for an unknown population parameter on the basis of information collected from a sample. Any statistics, for example, values of mean, proportion, correlation coefficient, computed from the sample for estimating the unknown population parameters is considered as point estimate, as this is a single (point) value/figure and no confidence of any kind can be associated with this value. The larger the sample size, nearer will be this estimated value to the unknown population parameter. On the other hand, interval estimate or confidence interval give us an interval (a lower limit, an upper limit) in which we believe the true parameter value lies, together with an associated probability. The objectives of interval estimation are to find narrow intervals with high reliability. Generally, the confidence probability is fixed as 0.95, 0.99, or 0.999 depending upon the requirement.
The lower limit and upper limit estimates for the statistic are given as
where, C = confidence coefficient, SE = {SD/√ n )}, and n = sample size.
Hypothesis Testing
Research questions, always for some target population, help the researcher to reach a valid conclusion using statistical method. This research question is made in terms of statistical hypothesis, which is nothing but a declarative statement about population parameters or distribution. For our hypothesis, we collect data, and based on this, a hypothesis is confirmed or rejected. In other words, Hypothesis testing permits us to determine whether enough statistical evidence exists to conclude that a belief (i.e., hypothesis ) about a population parameter is supported by the data.
Steps in Hypothesis Testing or Testing the Statistical Significance
- •
Define the null and alternative hypothesis
- •
Collect relevant data from a sample of individuals
- •
Calculate the value of test statistics specified under H 0
- •
Compare the value of this test statistics to value from a known probability distribution
- •
Interpret the p -value and results
Defining the Null and Alternative Hypotheses
We always test null hypothesis ( H 0 ), which assumes no difference or no effect in the population. It assumes that result observed in sample is purely due to chance. For example, if we are interested in recovery rates due to drug A and drug B in the population, the null hypothesis would be:
H 0 : recovery rates are the same due to both the drugs in the population.
We then define the alternative hypothesis ( H 1 ), which holds if the null hypothesis is not true.
Example: There is no difference in mean cholesterol level in obese and nonobese subjects. This is also known as researcher’s hypothesis.
H 1 : recovery rates are different for both the drugs in the population.
We have not directed for the differences in mortality rates, i.e., we have not stated whether drug A has higher or lower recovery rates than drug B. This leads to a two – tailed test , and it is recommended in most of situations, as we are rarely certain about the direction of any differences in advance, if this difference exists. In few situations, one may carry out a one – tailed test in which the direction of effect is specified in advance in H 1 . For example, we are considering a disease in which all untreated patients die.
Calculating the Test Statistic
After collecting data, values from our sample are substituted into a formula specific to the statistical test we are using to calculate a value for the test statistic . Usually, the larger the value of test statistic, ignoring its sign, greater is the evidence against the null hypothesis.
Obtaining, Using, and Interpreting the p -Value
All test statistics follow some known probability distribution. To obtain the p -value, we relate the value of the test statistic obtained from the sample to the known distribution. Most statistical software provides the two-tailed (sometimes one-tailed also) p -value automatically. The p -value is probability of getting a result as extreme as or more extreme than the one observed when the null hypothesis is true. It is also called observed level of significance or the probability of chance if the null hypothesis is true. Smaller the p-value , the greater the evidence against null hypothesis . When our study results in a probability of 0.02, we say that the likelihood of getting the difference we found by chance would be 2 in a 100 times. Conventionally, we consider that if the p -value is less than 0.05, we reject the null hypothesis and say that the result is significant at 5% level of significance. These cutoffs are arbitrary and have no specific importance.
Errors in Hypothesis Testing
As all result are based on sample, nothing can be said with 100% certainty in hypothesis testing procedure of inferential methods. So error always exists in acceptance or rejection of null hypothesis and it can be easily explained by using Table 63.1 .
The Possible Mistakes We Can Make
The possible mistakes we can make are of two types: Type I and Type II errors.
Type I error : It is defined as the probability of rejecting the null hypothesis when null hypothesis is actually true. In other words, probability of finding the effect of the treatment when effect of the treatment actually does not exists. It is also called as significance level ( α ) and generally fixed at 0.05, 0.01, and 0.001.
Level of confidence ( 1 − α ): It is defined as the probability of not finding the effect of the treatment when effect of the treatment actually does not exist.
Type II error : It is defined as probability of accepting the null hypothesis when null hypothesis is actually not true. In other words, probability of not finding the effect of the treatment when effect of the treatment actually exists. The chance of making this error is expressed by β . Conventional maximum allowable error is 20%.
Power of the test ( 1 − β ): It is defined as the probability of rejecting the null hypothesis when it is false or the probability of finding the effect of the treatment when effect of the treatment actually exists. Conventionally it is 80% or 90%.
Other Important Concepts That are Essential in Statistical Inference
Sampling distribution : The probability distribution of a statistic calculated from a random sample of a particular size of n or the distribution of the statistic (sample means, sample proportions) computed after taking repeated sample of a fixed size from the population.
Sampling error : Sampling error is the difference that occurs purely by chance between the sample statistic and the corresponding population characteristic that is being estimated. In practice, the sampling error can rarely be determined because the population parameter cannot be totally eliminated and can be reduced by increasing sample size.
Standard error ( SE ): The SE of a sample statistics is the SD of its sampling distribution. Many times, students are confused between the two terms: SE and SD. Both terms measure the variability; SD measures the variability among the observations of a variable in a sample, whereas SE measures the variability among the “statistic” values from different samples. SE = (SD/√ n ).
Parametric and Nonparametric Statistical Methods
Hypothesis tests that are based on the knowledge of the form of the frequency function of the parent population are known as parametric tests , and these tests deal with the parameters of the population. For example: student’s t -test, one-way ANOVA.
Nonparametric tests are the hypothesis tests in which assumptions about the shape of the distribution of the parent population are not made, for example, Mann–Whitney U test, Kruskal–Wallis test, etc.
Basic Principles of Statistics
The concept of probability and its understanding is very important while dealing with the application of statistical methods, which is commonly applied in the discipline of general anesthesia. For example, what is the probability of subtenon block being successful for perioperative analgesia in pediatric cataract surgery? What is the probability that patient with a pain will respond to intravenous fentanyl? Statistical methods can answer many questions like these, provided appropriate data are given. The probability can be outlined as expected occurrence of defined outcome of interest out of all possible outcomes (under repeated circumstances of the experiment). In other word, it is as the ratio of the number of outcomes that possess a specific characteristic “ r ” out of “ N ” number of trials (empirical probability).
where, N is large.
The value of probability, by definition, lies between 0 and 1. The probability of an event that cannot happen is 0 and that of an event certain to happen is 1. For example, suppose 10,000 patients are reported to have severe stomach pain in a tertiary care hospital in a year. After endoscopy, it has been found that 1000 patients had ulcer. What is the probability that a patient with severe pain in his or her stomach has an ulcer? This probability can be calculated by dividing 1000 patients (had ulcer) by 10,000 patients (who were reported to have severe pain in their stomach), which is equal to 0.1.
Probability Distributions
The principle of statistical inference is to collect data on sample of individuals and use the information derived from the sample to make inference about the population from where the sample was drawn. There is uncertainty in the relation between sample and population, and it is being handled with the ideas of probability and its distributions. The basic assumption of many statistical methods is that the observed data are a sample from the population with a distribution that has a known theoretical form. The choice of statistical methods is decided based on this distributional form, i.e., the statistical methods that make distributional assumptions are called parametric methods and those that make no assumptions about distributions are called distribution – free or nonparametric methods . The most important distribution is normal distribution, which is for continuous variables, and the other commonly used probability distributions in medical science are binomial (for binary variables) and Poisson (for discrete variables) distribution.
Study Design
After finalizing the research question, the next step is to transform the research question into a feasible and valid study plan by selecting an appropriate study design. Study designs encompass the structure and approach of a research in finding relevant answer to the research question. It describes the combination of ways in which the study group is formed and the order in which the exposure and outcome variables are measured.
Each of the study designs simply represents the different way of harvesting data to answer the research question appropriately and vary in planning, conduct, data analysis, and data interpretation. The selection of one study design over another depends on the research question, available resources, feasibility, and concerns about validity and ethical considerations. No amount of statistical adjustment can compensate for poor design of the study. Thus selection of appropriate study design forms the basis for good-quality research especially in clinical fields. Study designs are broadly classified into observational studies and experimental (trials) based on the assignment of exposure/intervention.
Observational study : In observational studies, the investigator does not assigns any intervention/exposure, allows the nature to take its course, and assumes a passive role in observing the events taking place in the study subjects. Even though randomized controlled trials (RCTs) are considered gold standard, there are many situations for which observational studies are better choice or the only feasible options. For example, assigning smoking and alcohol to the study subjects will be unethical where observational methods are the only choice. The observational studies take advantage of the natural/unintentional exposure of people to harmful/healthy substances. So they do not suffer from ethical and feasibility issues of experimental studies. The observational studies are further classified into descriptive and analytical studies based on the presence of comparison/control group as shown in Fig. 63.3 .

Case report : It is a descriptive study of a single case. It generally reports a new or unique finding like previous undescribed disease, its diagnosis and prognosis, unexpected link between diseases, new therapeutic effect, adverse events, etc. It is the least publishable unit in the medical literature and provides very weak empirical evidence. As such, it does not require any statistical consideration, e.g., Case Report on Apnea During Awake Epilepsy Surgery: an Unusual Cause for a Rare Complication.
Case series : It is a descriptive study on aggregates of individual cases in one report. It is composed of several similar cases that appeared in a short period. It informs about a very rare disease with few established risk factors. Case series can constitute the case group for a case-control study. As such, it may require only descriptive statistics for analysis.
Cross-sectional studies : In this study, the investigator measures both exposure and outcome variables on a single occasion in a defined population at a single point in time with no follow-up period. This makes them fast and inexpensive. They are well suited to estimate the prevalence (burden) and distribution pattern of disease/risk factors either in the community or hospital setting. This can be a descriptive study (without comparison group) or an analytical study (with comparison group). As the exposure and outcome variables are measured simultaneously, temporal sequence (exposure preceded the outcome) and incidence cannot be assessed thus limiting it to provide information on disease causation and prognosis.
Longitudinal study : It is a descriptive study (without comparison group) where a cohort (defined population) is followed over specific time period. It is prospective and measures incidence and prognosis of a disease. For example, in a longitudinal study of 71 consecutive patients with acute injury to the spinal cord, Lehmann and colleagues demonstrated persistent bradycardia among 31 patients with severe cervical cord injury.
Case-control studies : It is an analytical study and looks backward from outcome to exposure (retrospective). Typically such studies examine multiple exposures in relation to a disease. Subjects are defined as cases and controls and the exposure histories are compared. This design is useful for rare diseases, diseases with long induction/latent period, and for difficult/expensive exposure data. Controls should be sample of population that gave rise to cases, which may be from general population, hospital, clinic roster, friends, and relatives. Selection of controls is an important and critical activity in case-control study. Control-to-case ratio of more than 4 is not considered worthwhile. To minimize confounding, cases are matched with controls for known confounders (age, sex). Matching can be done through paired matching or frequency matching. Such matched case-control study requires matched analysis. Only odds ratio can be calculated from case-control study (neither prevalence nor incidence). Henzler and colleagues used a case-control study design to identify potentially modifiable contributors to secondary brain injury.
Nested case-control study : It is a case-control study nested in cohort design. The investigator identifies a cohort with banked specimens/information, identifies cases during the follow-up, and selects a sample of controls from rest of the cohort at the time each case develops. It avoids potential biases of conventional cases control study, reduces the cost by avoiding expensive measures on the entire cohort, and allows temporality between exposure and outcome.
Nested case-cohort study : Here the investigator identifies a cohort with banked specimens/information, selects a sample of controls from the cohort at beginning of the study, and identifies cases during the follow-up.
Cohort study : It is analytical study and looks forward from exposure to outcome (prospective). It typically examines multiple outcomes of an exposure. Subjects are defined as exposed and nonexposed and followed for disease occurrence. This is used for studying causation, incidence, and prognosis of the disease. Cohort study provides stronger evidence than the case-control study. In historic/retrospective cohort, both exposure and outcome have occurred before the start of the study. In concurrent/prospective cohort, only exposure has occurred before the start of the study. Mixed/ambidirectional cohort has both retrospective and prospective components. Cohort studies are inefficient for rare outcomes and diseases with long induction/latent period. They are expensive, time-consuming, and require large sample size. Incidence and relative risk can be calculated from cohort study. A population-based, historical cohort study was conducted in Olmsted County, Minnesota, by Flaada and colleagues to assess the difference in observed and expected mortality by age among traumatic brain injury (TBI) cases.
Studies of medical tests : Most of them are observational designs that assess reliability, accuracy, feasibility, costs, and risk of the medical test. Among these, diagnostic accuracy tests are conducted more frequently in clinical settings where the new test is compared with gold standard test to assess how well the new test correctly identifies or rules out disease. The results of this study are usually summarized using sensitivity, specificity, receiver operating characteristic curves, and likelihood ratios. In a study by Tsivgoulis and colleagues that used angiography as gold standard, transcranial Doppler was found to have a sensitivity of 88% and specificity of 89% in detecting complete recanalization during intra-arterial procedures for acute ischemic stroke when compared with the gold standard angiography.
Experimental studies : The assignment of intervention/exposure to the study subjects actively by the investigator is the hallmark of experimental studies that distinguishes it from observational studies. Experimental studies can be classified in several ways, depending on their design and purpose. Based on comparison group into uncontrolled / open trials (without comparison group) and controlled trials (with comparison group), based on the unit of assignment into individual (treatment is allocated to individuals) and community / cluster (treatment is allocated to entire community/cluster) trials, based on the purpose into preventive / prophylactic trials (prophylactic agent is given to healthy/high-risk individuals to prevent disease occurrence) and therapeutic / clinical trials (treatment is given to diseased individuals to reduce the risk of recurrence, improve survival/quality of life), based on the method of treatment administration into parallel (individuals in each group concurrently receive only one treatment) and cross – over trials (individuals in each group receive both treatments one after another and only the order of treatment differs; each person may serve as his or her own control; washout period may intervene between treatments), based on the number of treatment being tested into simple (experimental group gets only one treatment) and factorial trials (two or more treatments are combined and allows the investigator to test the separate and combined effects of several agents), and based on randomization into randomized (random assignment of intervention) and quasiexperimental (no random assignment of intervention).
Therapeutic / clinical trials have well-established sequence for assessing therapeutic effect of new drugs. Phase I is out carried on healthy volunteers (20–80) to assess safety, pharmacodynamics, pharmacokinetics, and maximum tolerated dose for a drug. Phase II is carried out on patients (100–200) to assess efficacy, dose–response, and side effects. Phase III is carried out on larger samples of patients to show the effectiveness of new drug over the standard one. Phase IV trial (post marketing surveillance) is carried out to assess long-term safety and efficacy of drug.
RCT : It is a planned experiment designed to assess the efficacy/effectiveness of an intervention in human beings by comparing the intervention to a control condition, using randomization. Randomization means random assignment of intervention to the study subjects. It does not refer to random selection of study subjects from the source population. Usual methods of randomization are: simple Randomization, stratified randomization, block randomization, and minimization. A well-conducted RCT produces more scientifically rigorous results and is considered as gold standard of study designs because the potential for bias (selection into treatment groups) is avoided. The group that has received intervention is called experimental group and the comparison group is called control group, which may receive placebo/alternate therapy/no therapy. Bulger and colleagues conducted a randomized controlled trial to determine the effectiveness of out-of-hospital administration of hypertonic fluids in improving neurologic outcome following severe TBI.
Nonrandomized / quasiexperimental studies : The study subjects are allocated without randomization to experimental and control groups. There are many types of quasiexperimental studies. The most common quasiexperimental design is the comparison group pretest/posttest design. This design is the same as the classic controlled experimental design except that the subjects cannot be randomly assigned to either the experimental or the control group.
The classic analytic approach for an experimental study is known as intention-to-treat (ITT) analysis. In this analysis, all individuals who were randomly allocated to treatment initially are analyzed, regardless of whether they completed/received the treatment. The usual measures of effect used in experimental studies are risk difference, number needed to treat, relative risk, or odds ratio.
Finally, comparison of various attributes of all possible study designs is given in Table 63.2 .
Attribute | Cross-Sectional | Case-Control | Cohort | Experimental |
---|---|---|---|---|
Assignment of intervention/exposure | No | No | No | Yes |
Directionality | Outcome and exposure measured simultaneously | From exposure to outcome | From outcome to exposure | From outcome to exposure |
Study group | Exposed or diseased | Cases (diseased) | Exposed | Experimental group (received intervention) |
Comparison group | Nonexposed or nondiseased | Controls (nondiseased) | Nonexposed | Control group (not received intervention) |
Temporal sequence | Hard to establish | Hard to establish | Easy to establish | Easy to establish |
Multiple associations | Can study multiple exposures and outcome | Often one outcome with multiple exposures | Often one exposure with multiple outcomes | Can study multiple interventions and outcomes |
Time and money | Less expensive | Least expensive | Expensive | Most expensive |
Sample size | Large or small | Relatively small | Relatively large | Large |
Measures of effect and association | Prevalence Prevalence odds ratio | Mainly odds ratio only | incidence relative risk Risk difference Odds ratio Hazard ratio Survival analysis | Incidence relative risk Risk difference Odds ratio Hazard ratio Survival analysis |
Best when | Onset of disease is prolonged | Outcomes are rare, attrition is problem | Exposures are rare, selective survival is problem, all factors are not known | Assessing therapeutic/preventive effect of drug/intervention |
Evidence of causality | Only suggestive | Needs more careful analysis | Strong | Strongest |
Biases | Difficult to mange | Needs more effort to mange | Easy to manage | Easy to mange |
Other issues | Cannot measure incidence of disease | Selection of appropriate control often difficult | Directly measures risks | Ethical issues are critical Directly measures risks |
Sample Size
Sample Size in Clinical Trials
Sample size plays vital role in RCTs. The optimum sample size should be estimated to have a desired power for detecting a clinically meaningful difference. At the same time, it is also important to balance between cost-effectiveness and power due to rare condition of the disease and limited budget. The sample size of the study is calculated in the planning stage of the study, and it depends on clinical as well as statistical inputs. The clinical inputs include primary objective, study design, and type of primary outcome and the statistical inputs include hypothesis being tested, statistical analysis being used, type I error (alpha, α ), and type II error (beta, β ).
In clinical trials, sample size is derived by method of treatment administration and hypothesis tested. For parallel treatment administration, the hypotheses that may be tested include equality, superiority, and noninferiority hypotheses and the details of sample size for all these three hypotheses are given in Tables 63.3A–C .
Inputs for Sample Size | Example 1 | Example 2 |
---|---|---|
Objective | Comparison of test and placebo drug for reducing cholesterol for treatment of patients with CHD | Comparison of test and placebo drug of daily dose for treatment of skin infection |
Design | Parallel | Parallel |
Primary outcome | Percent change in LDL cholesterol | Cure |
Type of primary outcome | Continuous outcome | Binary outcome |
Hypothesis being tested | Test for equality H 0 : μ T = μ P vs. H 0 : μ T ≠ μ P (Two-sided) | Test for noninferiority H 0 : P T = P P vs. H 1 : P T ≠ P P (Two-sided) |
Level of significance | 5% | 5% |
Power | 80% or 90% | 80% or 90% |
Sample size formula | n=2(z1−α/2+z1−β)σ2(μT−μP)2 n = 2 ( z 1 − α / 2 + z 1 − β ) σ 2 ( μ T − μ P ) 2 | n=(z1−α/2+z1−β)2[pT(1−pT)+pP(1−pP)](pT−pP)2 n = ( z 1 − α / 2 + z 1 − β ) 2 [ p T ( 1 − p T ) + p P ( 1 − p P ) ] ( p T − p P ) 2 |
Inputs for Sample Size | Example 1 | Example 2 |
---|---|---|
Objective | Comparison of two-cholesterol lowering agents for treatment of patients with CHD | Comparison of two microbial agents of daily dose for treatment of skin infection |
Design | Parallel | Parallel |
Primary outcome | Percent change in LDL cholesterol | Cure |
Type of primary outcome | Continuous outcome | Binary outcome |
Hypothesis being tested | Test for noninferiority H 0 : μ T − μ S ≥ Δ I vs. H 1 : μ T − μ S < Δ I (One-sided) | Test for noninferiority H 0 : P S − P T ≥ Δ I vs. H 1 : P S − P T <Δ I (One-sided) |
Level of significance | 5% | 5% |
Power | 80% or 90% | 80% or 90% |
Sample size formula | n=2(z1−α+z1−β)σ2[(μT−μS)−ΔI]2 n = 2 ( z 1 − α + z 1 − β ) σ 2 [ ( μ T − μ S ) − Δ I ] 2 | n=(z1−α/2+z1−β)2[pT(1−pT)+pS(1−pS)][(pT−pS)−ΔI]2 n = ( z 1 − α / 2 + z 1 − β ) 2 [ p T ( 1 − p T ) + p S ( 1 − p S ) ] [ ( p T − p S ) − Δ I ] 2 |
Inputs for Sample Size | Example 1 | Example 2 |
---|---|---|
Objective | Comparison of two-cholesterol lowering agents for treatment of patients with CHD | Comparison of two microbial agents of daily dose for treatment of skin infection |
Design | Parallel | Parallel |
Primary outcome | Percent change in LDL cholesterol | Cure |
Type of primary outcome | Continuous outcome | Binary outcome |
Hypothesis being tested | Test for superiority H 0 : μ T − μ S ≤ Δ vs. H 1 : μ T − μ S > Δ (One-sided) | Test for superiority H 0 : P S − P T ≤ Δ vs. H 1 : P S − P T > Δ (One-sided) |
Level of significance | 5% | 5% |
Power | 80% or 90% | 80% or 90% |
Sample size formula | n=2(z1−α+z1−β)σ2[(μT−μS)−Δ]2 n = 2 ( z 1 − α + z 1 − β ) σ 2 [ ( μ T − μ S ) − Δ ] 2 | n=(z1−α/2+z1−β)2[pT(1−pT)+pS(1−pS)][(pT−pS)−Δ]2 n = ( z 1 − α / 2 + z 1 − β ) 2 [ p T ( 1 − p T ) + p S ( 1 − p S ) ] [ ( p T − p S ) − Δ ] 2 |
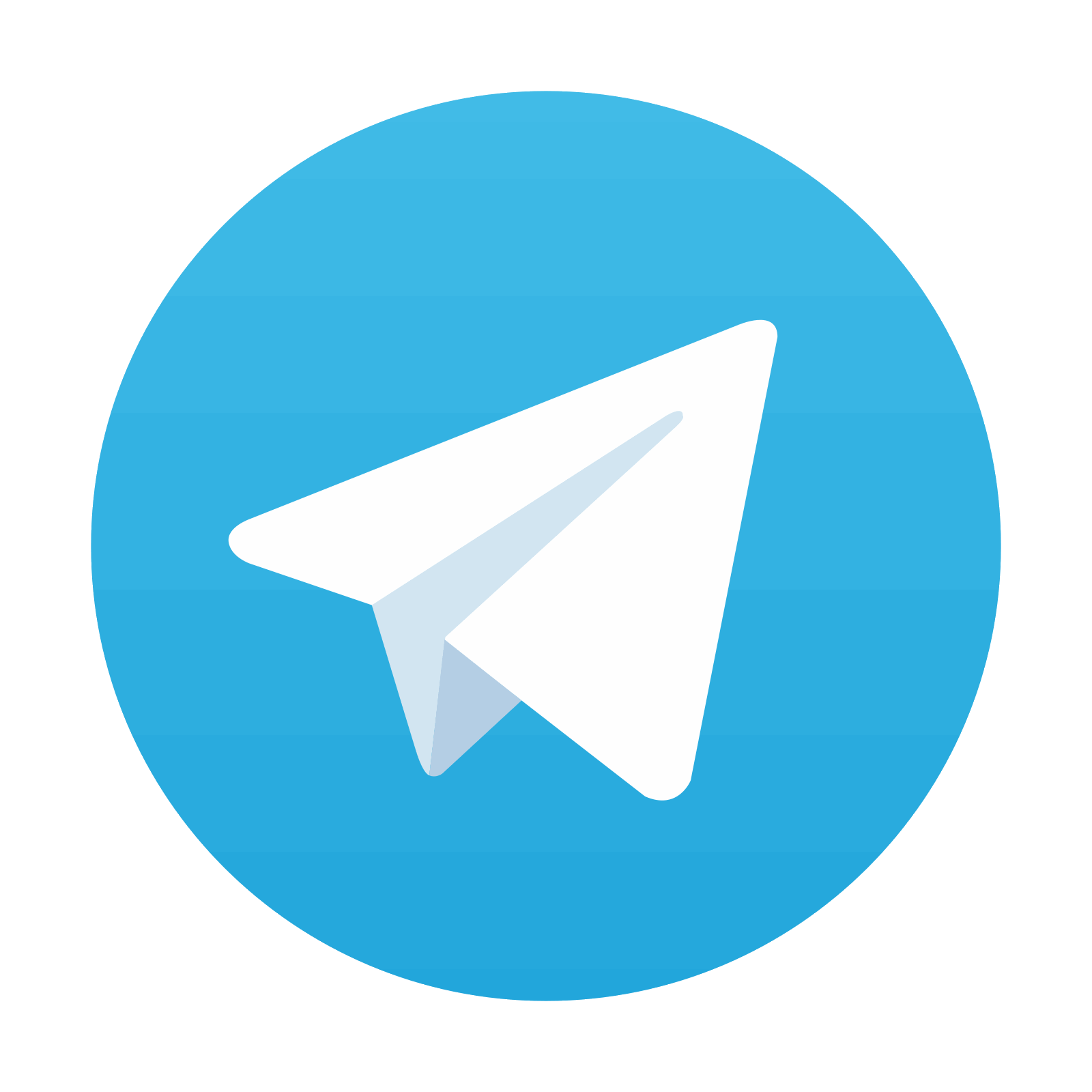
Stay updated, free articles. Join our Telegram channel

Full access? Get Clinical Tree
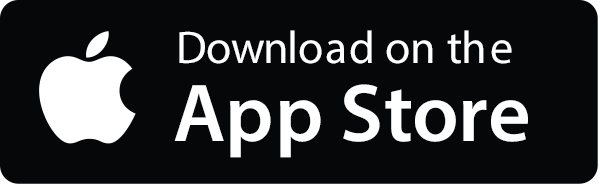

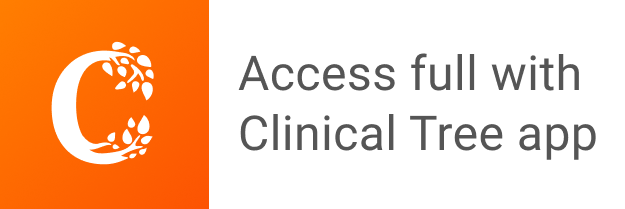