Assessing the Value and Impact of Critical Care in an Era of Limited Resources: Outcomes Research in the Intensive Care Unit
Andrew F. Shorr
William L. Jackson Jr
Derek C. Angus
During the last three decades, critical care has matured to a distinct medical specialty. Sepsis, respiratory failure, and the care of the complicated postoperative patient are now perceived as the purview of the intensivist. Concomitant with this evolution in critical care medicine has been a growing focus on health care outcomes. This emphasis on the end points and effects of medical care generally and critical care specifically reflects the realization that critically ill subjects face a high risk of death and that many interventions applied in the intensive care unit (ICU) are expensive. Some older studies estimate that nearly 1% of the gross national product of the United States is consumed in the ICU and, relative to days spent on hospital wards, others suggest that ICU costs are nearly three times greater [1,2]. Whether it is mechanical ventilation (MV), extensive nursing care, or acute dialysis, many of the technologies and medications used in the ICU are associated with substantial economic costs. In addition, many often perceive that ICU interventions only delay mortality rather than prevent mortality, or that mortality reduction in the ICU comes only at the price of significant morbidity. Thus, there is increasing pressure to carefully evaluate and to understand the results of ICU care. This pressure becomes even more evident when one considers that ICU outcomes must be evaluated from both patient and societal perspectives. In other words, the emphasis on outcomes in the ICU reflects an underlying question about value.
Outcomes research reflects a systematic effort to address these issues and concerns. According to a recent position statement on outcomes research in critical care, “Outcomes research is employed to formulate clinical practice guidelines, to evaluate the quality of care, and to inform health policy decisions” [3]. Like clinical critical care, outcomes research draws on many different tools and expertise in multiple disciplines. More than only an issue of economics, outcomes research requires expertise in psychology and anthropology (to understand patient and physician behavior), epidemiology (to identify disease patterns and burdens), and health services research (to appreciate process) [3]. Use of a term like outcomes, though, presupposes a question: Outcomes for whom? At the bedside, the clinician or the investigator focuses on pathophysiology of a sole patient. Outcomes research addresses broader issues. Rather than being either centered on a particular disease or a physiologic measure, outcomes research deals with the overall results of care for the patient, for the family, and for society. Also in distinction to traditional clinical research, outcomes research has clear policy aspects as well; it attempts to facilitate debates about competing plans for resource allocation, research priorities, and national health policy. As an example, a randomized clinical trial deals with issues of efficacy (Does intervention “x” in a controlled environment have an independent impact?) and outcomes research is more concerned with effectiveness (What are the implications of intervention “x” applied outside a controlled setting and in the “real world” for the patient and society?). Traditional clinical research, moreover, often employs experimental approaches, and observational methods are routinely used in outcomes research. In short, outcomes research attempts to use methods from the social sciences to augment the understanding of health care as opposed to using only methods from conventional “hard” sciences. As a recent summary regarding outcomes research in sepsis indicated, the outcomes researcher seeks to answer a question separate from traditional research [4]. The clinical investigator essentially asks, “Does this work?” and outcomes researchers deal with the concern, “Does it help?” [4].
Readers should note that outcomes research is now a key component of the biomedical enterprise. It is no longer seen as an option or an add-on. It fits with mechanistic and clinical work in building the triumvirate of information needed to translate research findings into clinical practice. The absence of outcomes studies can lead to the failure to adopt what otherwise might be useful interventions.
Methods in Outcomes Research
Outcomes research relies on multiple methods for exploring patient-centered concerns. Generally, researchers employ both qualitative and quantitative methods [3]. Qualitative approaches are only occasionally used but can offer insight into complex processes that do not easily lend themselves to standard hypothesis testing. As such, qualitative work often results in the generation of important hypotheses for more formal testing. Quantitative methods are more standard in outcomes research in critical care and have two general aspects. First, they use some tool to measure a particular outcome (e.g., mortality, quality of life, functional status, cost). Second, quantitative techniques then seek to compare the outcome of interest between at least two alternatives. Unlike the controlled environment of the bench laboratory or even the randomized controlled trial (RCT), outcomes research is necessarily exposed to multiple potential confounders that can and do affect the primary measure of interest. Because critical care outcomes research remains patient-centered, it is important to acknowledge that these subjects bring with them complexities that may alter their mortality, quality of life, and function. Moreover, the impact of these preceding factors may affect a researcher’s end point of interest in ways that have little to do with the
intervention under study. Similarly, after any intervention in the ICU, many post-ICU variables come into play that might affect the results of an outcomes study.
intervention under study. Similarly, after any intervention in the ICU, many post-ICU variables come into play that might affect the results of an outcomes study.
To address these complexities requires adoption of various techniques, all of which must be rigorous and reproducible. Therefore, outcomes research relies on more than simply RCTs. RCTs are well suited for deciding if specific interventions or agents can alter an easily ascertainable end point such as mortality. For example, use of large sample sizes combined with both block randomization techniques and protocols for patient care help to ensure that the potential confounders previously noted are minimized and, in turn, allows one to explore questions such as how low tidal volume MV affects mortality at day 28. But if the policy or research query deals with the functional status or total cost of care for survivors of acute respiratory distress syndrome (ARDS) more than a year after their hospital discharge, one may require additional approaches other than an RCT. In any event, critical care outcomes research begins by defining a particular question. The investigator can subsequently determine which approach is most appropriate.
In fact, sometimes outcomes research requires entirely separate study designs and major modifications to traditional models of clinical research. In other cases, more traditional models of investigation can be expanded to incorporate outcomes measures. This generally requires building these measures into the trial during the study inception phase. Therefore, outcomes research can be seen as an extension and complement to standard research practices. In other areas of medicine, such as rheumatology, patient-centered measures such as quality of life have come to serve as the primary end point in clinical studies.
Observational Studies
Of the various types of observational studies (e.g., case series, case-control, cross-sectional, and cohort), two are particularly important in critical care outcomes. A cross-sectional design has the advantage of looking at one precise time or over a short period of time at a specific disease or practice. This snapshot-in-time approach can provide important insight into both epidemiology and health services research. For example, a recent 1-day international survey of respiratory failure in the ICU demonstrated the burden of this disease relative to other diseases treated in the ICU and also documented the wide range in practice style with respect to the use of MV [5]. The Sepsis Occurrence in Acutely Ill Patients (SOAP) study, a European sepsis registry using an essentially cross-sectional design (it covered a set 2-week period) confirmed the burden of sepsis in the ICU and underscored the variability in the use of various medical therapies in the care of these patients [6]. Hence, these cross-sectional analyses generated important information about the current state of affairs and therefore provided a potential benchmark for use in future comparisons.
In addition, cohort studies are valuable components in outcomes research. With this strategy, subjects are selected based on some common characteristic (e.g., a diagnosis, a risk factor) and then observed [7]. Thus, cohort analyses have the advantage of being prospective. Cohort studies also specify a set starting time for the observation (e.g., time zero) from which observations proceed forward. Researchers can then look at the interplay of certain predefined risk factors or interventions and the characteristics that defined the cohorts to see how these affect the outcome. Often a cohort design is used to either describe the natural history of a disease or to assess quality of life. Although theoretically straightforward, cohort studies pose important challenges to the researcher. Selection bias and the inherent heterogeneity of critically ill patients can confound efforts to create a homogeneous cohort. Similarly, one needs to ensure means for capturing multiple potential exposure variables and acknowledge that the interaction between risk factors, exposures, and time is complex.
As Needham et al. [7] and Dowdy et al. [8] indicate in a recent review of methodologic issues associated with cohort studies, this study design has three key components: subjects, outcomes and exposures, and time. Subjects must be carefully identified, but the cohort study gives the researcher flexibility to define the population as sharing particular characteristics, such as common diagnoses, or risk factors. Alternatively, cohorts can be developed such that two groups emerge: individuals exposed to a particular event or variable and those not exposed. As a result, one can, using this technique, begin to draw conclusions about causal relationships. Generally, because the cohort shares some common time of designation (e.g., time zero) by observing the population one can evaluate the strength of the relationship between the given exposure and the outcome. Unlike the rigidity of an RCT, in which randomization works to ensure study groups are similar except for the intervention in question, a cohort design provides the researcher the chance to explore multiple exposures simultaneously, and how they interact with each other. To the point, in an RCT of a novel treatment for sepsis, any differences seen in outcomes should be a function of the particular intervention experimentally introduced. The ICU organization, pre-ICU care, and posthospital events should not affect the outcome because randomization should ensure that the impact of these variables is equalized between the active and comparator groups.
The purpose of a cohort study is to enhance the RCT by providing information that cannot, by definition, be gleaned from the RCT. Expanded adoption of cohort studies can also facilitate better understanding of natural history by shifting the focus back to a time prior to ICU admission. Without some initial work with adopting a cohort approach, we cannot hope to address significant questions relating to what determines which patients get admitted to the ICU, who most likely benefits from ICU care, and the outcomes for those never admitted to the ICU.
Interventions and End Points in Critical Care Outcomes Research
Unlike traditional biomedical research, which looks at either novel technologic interventions (new drugs, new devices) or perhaps management strategies, the interventions studied in outcomes research are more diverse. Certain clinical measures have significant outcomes implications for the patient and society. However, managerial and organizational changes may be equally important. The issue of management and organization of critical care services is particularly acute at present, given current (and conflicting) data suggesting that the model of ICU administration affects both mortality and cost [9,10]. The question of organization and management is broader than simply whether one uses a closed, full-time intensivist model or a more traditional open ICU model. Under the rubric of organization and management are questions of nurse-to-patient ratios, the role for respiratory therapy, and the value of a dedicated critical care pharmacy group. Measuring how these types of potential features of the ICU work and whether they help patients and society is perhaps as important a question as if a new molecule for sepsis alters mortality. Issues of management and organization can provide feedback to affect the conduct of traditional research. Whether it is studies of resuscitation strategies or rapid response teams, these types of interventions
include service, delivery, and organizational aspects. If any one of these components of the trial collapses, the entire venture may be jeopardized.
include service, delivery, and organizational aspects. If any one of these components of the trial collapses, the entire venture may be jeopardized.
Mortality
With respect to end points, mortality remains the center of investigative efforts because it has tangible meaning to the patient, to health care institutions, and to society. When outcomes research addresses mortality, it tries to do it in an appropriate context. In other words, the question of mortality begs the question of when? Is the appropriate timeframe survival to ICU discharge or to hospital discharge? Are these time points too myopic? Altering long-term mortality (e.g., 2 years after ICU admission) would be an admirable goal. Historically, 28-day all-cause mortality has served as the primary end point for trials in critical care. However, after some period of time it seems reasonable to postulate that occurrences and interventions in the ICU diminish in their impact while the patient’s age [11] and health state prior to his or her ICU admission [3] become the main drivers of outcomes. Thus, the issue revolves around the timeframe chosen for measurement and its likely mechanistic link to the intervention under evaluation [12].
It is important to be cautious, though, since one can artificially alter ICU mortality by early use of certain interventions (e.g., tracheotomy in order to facilitate transfer to a chronic ventilator care facility). Likewise, decisions about when to suggest withdrawal of care can alter the apparent timing of death in the ICU. The central limitation is that with all time-dependent end points, there can be confounding by multiple factors. As the recent American Thoracic Society position statement on outcomes research appropriately observes, “The ‘correct’ mortality endpoint depends on the specific research question, the mechanisms and timing of the disease and/or treatment under study, and the study design” [3]. In addition, if a disease state is not associated with significant mortality, use of this measure may simply fail to capture the value of a particular intervention. Finally, mortality as the sole end point of any research ignores the entire concern about morbidity and the tradeoff between mortality and morbidity. Similarly, it fails to address the quality of life of the survivor.
Mortality, moreover, has limitations as a tool for comparing outcomes across different ICUs. Although recorded and tracked nearly uniformly in ICUs throughout the world, ICU mortality is a relatively uninformative measure of ICU performance. Extensive variability exists in not only the types of patients admitted to different ICUs but also in admission and discharge policies [12]. Some ICUs serve as major referral centers for and receive multiple transfers from other hospitals. These patients tend to be sicker or in need of specialized care. Hence, the mortality rates of the ICUs that send these persons elsewhere may be artificially low compared to the ICUs that accept such high-risk cases. Similarly, ICUs with intermediate-care facilities can transition individuals out of the ICU at different rates than ICUs lacking access to these resources. This fact can alter apparent ICU mortality rates because one might essentially be able to transition patients receiving comfort care only out of the ICU so that when they die the death is not captured as an ICU-related event.
One could correct for these possible variables by employing a definition of ICU mortality (for benchmarking performance) that removed transfers from both the numerator and denominator of the crude mortality rate. Adjusting for differences for availability of “stepdown” wards can be made by limiting comparisons to like-sized hospitals. However, even these efforts would be insufficient for purposes of performance and quality assessments because issues of case-mix remain unaddressed. Case-mix as a concept tries to capture that different ICUs admit different types of patients with differing severity of illnesses. It is important to note that case-mix as a concept describes more than differences in disease severity [13]. Case-mix adjusting tries to balance issues with underlying diagnosis, comorbidity, age, and severity of illness [13]. To illustrate the breadth of the aspects related to case-mix one need only consider an ICU that cared for only postoperative cardiothoracic patients should report low mortality rates and an ICU that admitted mainly immunocompromised persons would certainly describe different outcomes, even after one adjusted for severity of illness. As a corollary, comparing mortality between similar types of ICUs that admit similar types of patients, after controlling for disease severity, can prove helpful [13].
Severity of Illness Tools
To address disease severity, multiple tools exist. They differ with respect to the variables they measure, when they measure these variables, and if they try to describe ICU mortality or hospital mortality. The Acute Physiology and Chronic Health Evaluation (APACHE) score is commonly used in the United States and the Simplified Acute Physiology Score (SAPS) system is more regularly employed in Europe [14,15,16]. Severity of illness scores have been developed for application in specific types of patients (e.g., pediatrics, trauma) and others try to deal with a broader range of subjects. Other modeling systems include the Sequential Organ Failure Assessment (SOFA) score and the Multiple Organ Dysfunction Score [17,18]. A major limitation of all scoring systems is that they are developed and validated on large patient populations. Therefore, predicted mortality estimates for individual patients cannot and should not be translated into decisions at the bedside as to whether, based solely on predicted mortality, one should withhold or offer aggressive care.
Another concern with severity of illness tools as they relate to mortality is that some were initially created many years ago. Over time, new interventions and technologies have altered patient care and morality. Hence, older iterations of certain models may not longer apply and no longer have adequate calibration to be informative. Like many scales, they require recalibration. As an example, the APACHE system is now on its fourth revision, and with APACHE II versus APACHE IV, there are significant differences in terms of the explanatory power [19]. Nonetheless, in critical care research many have adopted the APACHE II and III approach as its equations are published. Researchers and administrators need therefore be cautious when assuming that similar scores computed by an older rubric necessarily translate into similar predicted mortalities among populations or across ICUs. APACHE generally functions by exploring historical cohorts of patients and creating prediction scores based on this “control” population. Alternatively one can also use the acuity measures used in these instruments to derive from predictions that are specific for the population of interest or under study.
Calculations of the actual scores for patients can also be prone to error. Several studies document significant interobserver variability among even trained researchers as to the calculation of severity of illness scores [20]. With APACHE II, one project revealed that the interrater agreement was strikingly poor (κ = 0.20) [21]. The main sources of variability appeared to be in assessment of the Glasgow Coma Score but variability was evident even in the determination of the blood pressure. Changes in practice can also have unpredicted effects on severity of illness scores. Nearly all scoring systems rest on measurement of physiologic parameters such as blood pressure, platelet count, and hemoglobin. The more extreme the actual value from the “normal” range, the greater the negative impact of this factor on the individual’s composite
severity of illness score. As an example, a low hemoglobin is associated with more APACHE II points than a normal hemoglobin. Clinicians, though, may now be more tolerant of lower hemoglobins than they were when APACHE II was created. In fact, a restrictive transfusion strategy that necessarily allows the hemoglobin to drift lower may improve outcomes [22]. Consequently, APACHE II scores may be rising in ICU patients over time, reflecting this change in practice because physicians are not transfusing as frequently. This increase in APACHE II-predicted mortality when actual mortality might improve because of a change in clinical practice based on a large randomized trial underscores a significant assumption and limitation of severity of illness scoring classifications.
severity of illness score. As an example, a low hemoglobin is associated with more APACHE II points than a normal hemoglobin. Clinicians, though, may now be more tolerant of lower hemoglobins than they were when APACHE II was created. In fact, a restrictive transfusion strategy that necessarily allows the hemoglobin to drift lower may improve outcomes [22]. Consequently, APACHE II scores may be rising in ICU patients over time, reflecting this change in practice because physicians are not transfusing as frequently. This increase in APACHE II-predicted mortality when actual mortality might improve because of a change in clinical practice based on a large randomized trial underscores a significant assumption and limitation of severity of illness scoring classifications.
Severity of Illness and Performance Assessment
Mortality prediction equations can also result in calculation of a standardized mortality ratio (SMR) [13]. This ratio compares observed mortality to predicted mortality. Conceptually, the SMR can be calculated irrespective of the severity of illness system used to determine the predicted mortality. Ratios greater than 1 suggest excess mortality and those less than 1 imply enhanced survivorship. Implicitly, an SMR greater than 1 indicates an ICU with inferior outcomes after adjusting for severity of illness case mix. Alternatively, though, differences in SMR can reflect more than quality. First, scoring systems may be generally imprecise (see previous discussion) and may not capture some aspects of disease severity or other case-mix issues. Second, the SMR can be affected by the quality of data collection and by the sample size. There is also discordance in the published literature exploring if and how well the SMR correlates with other markers of ICU quality. Some investigators suggest the SMR sufficiently captures aspects of quality and others conclude that the relationship between other markers of quality and the SMR is less clear [13]. It is likely that no one SMR calculation method accurately reflects quality. Therefore, as policy makers, third-party payers, and patients demand simple report cards that allegedly capture quality, it is important that the intensivist resist the urge to simply publish SMRs without references to case-mix. Some more recent scoring systems address this (i.e., APACHE IV) but still may be imprecise as they derive from historical cohorts. We need to encourage the use of multiple measures beyond the SMR to describe qualitative differences in ICUs.
Nevertheless, the SMR can be used over time to assess interventions within an ICU or group of relatively homogenous ICUs [13]. Although one may not be able to conclude that SMR differences across institutions reflect true differences in quality and performance, when used as a benchmarking tool the SMR can be insightful. If one ICU has historical data about its case-mix and performance, it can then track over time how the SMR varies in response to interventions. Conversely, an increasing SMR can suggest the presence of some change in practice or structure that is adversely affecting mortality. By identifying these trends and investigating them, ICU staff can elucidate potentially harmful changes that have transpired and attempt to address them.
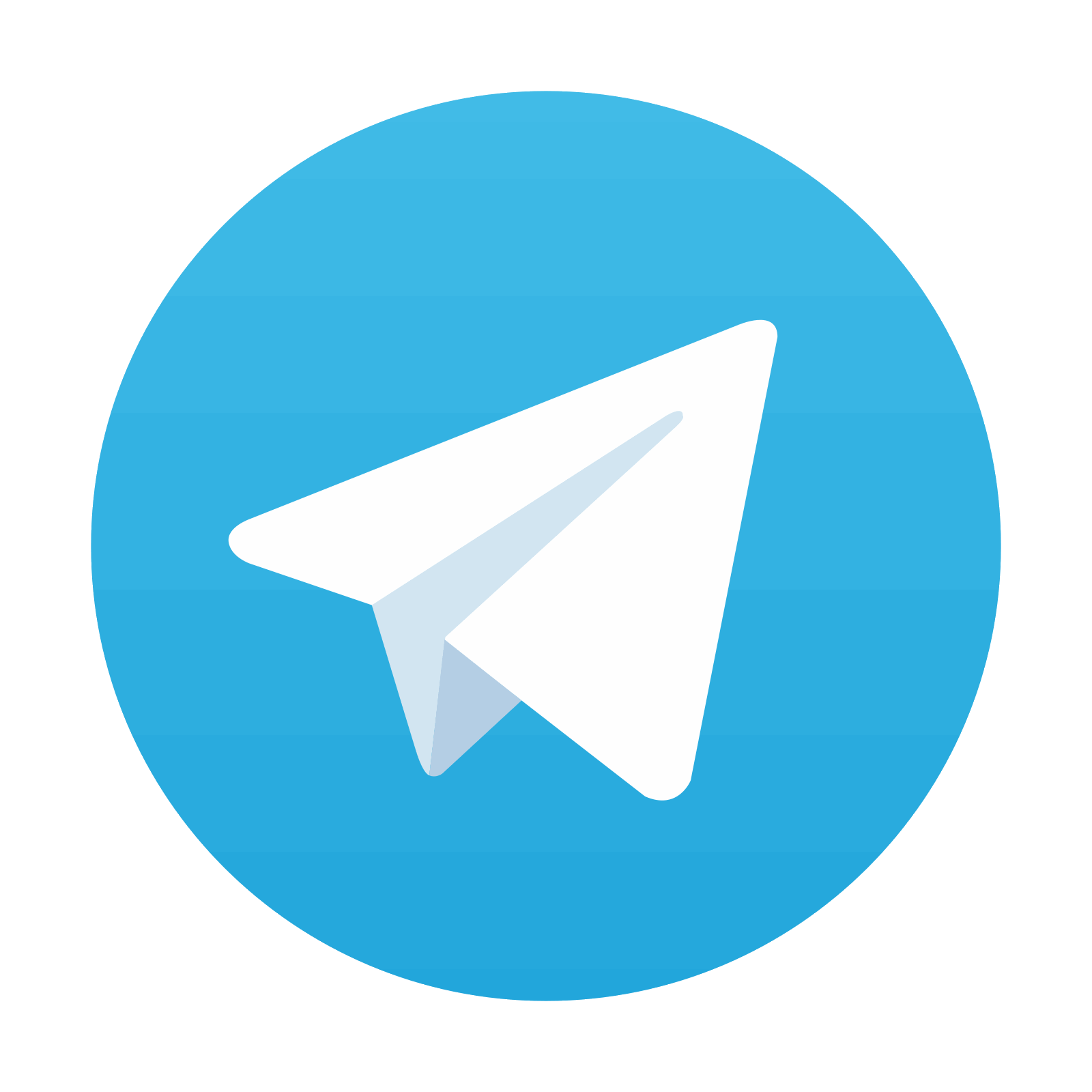
Stay updated, free articles. Join our Telegram channel

Full access? Get Clinical Tree
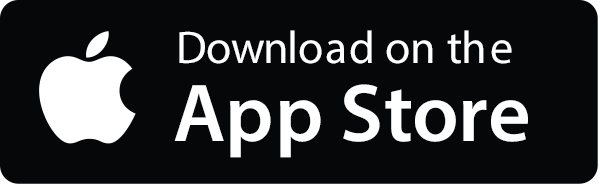
