Abstract
The goal of pharmacological treatment is a desired response, known as the target effect (e.g. bispectral index of 50). An understanding of the concentration–response relationship (i.e. pharmacodynamics (PD)) can be used to predict the target concentration (e.g. propofol 4 mg/L) required to achieve this target effect in a typical individual [1]. Pharmacokinetic (PK) knowledge (e.g. clearance, volume) then determines the dose that will achieve the target concentration. Each individual, however, is somewhat different and there is variability associated with all parameters used in PK and PD equations (known as models). Covariate information (e.g. weight, age, pathology, drug interactions, pharmacogenomics) can be used to help predict the dose in a specific patient. The Holy Grail of clinical pharmacology is prediction of drug PK and PD in the individual patient (Fig. 4.1) and this requires knowledge of the covariates that contribute to variability [2].
Introduction
The goal of pharmacological treatment is a desired response, known as the target effect (e.g. bispectral index of 50). An understanding of the concentration–response relationship (i.e. pharmacodynamics (PD)) can be used to predict the target concentration (e.g. propofol 4 mg/L) required to achieve this target effect in a typical individual [1]. Pharmacokinetic (PK) knowledge (e.g. clearance, volume) then determines the dose that will achieve the target concentration. Each individual, however, is somewhat different and there is variability associated with all parameters used in PK and PD equations (known as models). Covariate information (e.g. weight, age, pathology, drug interactions, pharmacogenomics) can be used to help predict the dose in a specific patient. The Holy Grail of clinical pharmacology is prediction of drug PK and PD in the individual patient (Fig. 4.1) and this requires knowledge of the covariates that contribute to variability [2]. Adverse effects will also impact on dose.
Fig. 4.1 The complex interaction between pharmacokinetics (PK) and pharmacodynamics (PD). Administration of a dose must undergo multiple steps before effect is achieved. Each step in this pathway is associated with variability. In addition, each step experiences either positive or negative feedback from subsequent steps. For example, the drug may impact on the disease, but changes in the disease have multiple effects on PK, PD and the biophase. The use of concentration to describe effect removes variability associated with PK aspects.
Quantifying Covariate Effect
Parameter variability has two contributors: between subject variability (BSV; also known as inter-individual variability, IIV) and between occasion variability (BOV, also known as intra-individual variability). The difference between BSV without covariates and with covariates is a measure of the predictable decrease in BSV due to covariates. The variance (ω2) estimates for the different components contributing to variability of vancomycin pharmacokinetics are shown in Table 4.1 [3]. The ratio of the between-subject variability predictable from covariates (BSVP2) to the total population parameter variability obtained without covariate analysis (PPVt2) gives an indication of how important covariate information is. For example, the ratio of 0.823 achieved for clearance in a study of vancomycin in neonates indicates that 82.3% of the overall variability in clearance is predictable from covariate information. Weight explained 49%, postmenstrual age (PMA) 18% and renal function 34.1% [3].
Table 4.1. Effect of covariate analysis on variance of clearance (ω2) for vancomycin. The impact of each covariate when added sequentially to the model is demonstrated. (Adapted from Anderson BJ et al: Br.J.Clin.Pharmacol. 2007; 63: 75–84.)
Individual covariate alone | PPVt2 | BSVR2 | BOV2 | PPVP2 | PPVP2/PPVt2 | OBJ |
---|---|---|---|---|---|---|
No covariates | 0.279 | 0.279 | 0 | 0 | 0 | 2835.261 |
Allometric scaling | 0.279* | 0.0538 | 0.0862 | 0.139 | 0.498 | 2632.123 |
Postmenstrual age | 0.279* | 0.0452 | 0.0450 | 0.189 | 0.677 | 2514.865 |
Renal function | 0.279* | 0.0362 | 0.0137 | 0.230 | 0.821 | 2418.198 |
Ventilation scaling factor | 0.279* | 0.0356 | 0.0137 | 0.230 | 0.823 | 2413.725 |
*=assumed from no covariate model estimate
PPVt2 is the total population parameter variance estimated without covariate analysis, which is the sum of PPVP2 (the population parameter variance predictable from covariates), BSVR2 (the random BSV2 estimated on a parameter when covariate analysis is included) and BOV2 (the between occasion variance), or mathematically expressed: PPVt2=PPVP2+BSVR2+BOV2. The ratio of the between subject variance predictable from covariates (PPVP2) to the total population parameter variance obtained without covariate analysis (PPVt2, PPVP2/PPVt2) indicates the fraction of the total variability in the parameter that is predictable from covariates. OBJ is the objective function value, a parameter that measures the goodness of fit.
Major Covariates
Covariate effects will differ depending on the population studied. For example age may have a large effect in those aged less than 1 year or those aged more than 60 years, but minimal effect in young adults. The variability observed for any parameter (e.g. clearance) is contributed to by multiple covariate influences (Fig. 4.2). Covariate effects can be related to known biology (mechanistic), be based on theory or may be related to an observed variable that may or may not reflect physiology to some extent. The latter models are known as empirical. The American Association of Anesthesiologists (ASA) classification, for example, may be used to describe altered propofol disposition in patients when compared to healthy volunteers; this is an empirical model. Allometry (see below), in contrast, could be considered a theoretical model. There is no ‘best model’ to describe covariates. Theoretical models may fail if assumptions for the theory are not met.
Fig. 4.2 Schematic representation of factors contributing to clearance variability in children. Neonates have reduced clearance (e.g. morphine), pharmacogenomic influences may cause increased clearance (e.g. CYP2D6 fast metabolizers of codeine), renal failure may reduce clearance (e.g. aminoglycosides), other drugs may increase clearance (e.g. smoking and theophylline).
Major Paediatric Pharmacokinetic Covariates
Growth and development are two major aspects of children not readily apparent in adults. How these factors interact is not necessarily easy to determine from observations because they are quite highly correlated. Drug elimination clearance, for example, may increase with weight, height, age, body surface area and creatinine clearance. One approach is to standardize for size before incorporating a factor for maturation [4].
Size
Size, frequently expressed as weight, is the most common covariate used to determine dose in children [5]. The normal variation of weight with age (from 3rd to 97th percentile) is considerable, being least at 1 year (+25% to –20% at 10 kg) and reaching a maximum at about 13 years (+45% to –26% at 40 kg) [6, 7]. Although total body weight (TBW) is used commonly, it is now more widely recognized that there is a non-linear relationship between weight and drug elimination [8, 9]. Non-linear scaling models include body surface area [10], lean body weight [11], fat free mass [12], normal fat mass [13] and allometric scaling (defined below) [14].
Clearance in children 1–2 years of age, expressed as L/h/kg (the linear per kilogramme model), is frequently greater than that observed in older children and adolescents. This is a size effect and is not due to bigger livers or increased hepatic blood flow in preschool children. This ‘artefact of size’ disappears when allometric scaling is used to replot the same data (Fig. 4.3). Allometry is a term used to describe the non-linear relationship between size and function. This non-linear relationship is expressed as:
where y is the variable of interest (e.g. basal metabolic rate), a is the allometric coefficient and PWR is the allometric exponent. The value of PWR has been the subject of much debate. Basal metabolic rate (BMR) is the commonest variable investigated and camps advocating for a PWR value of 2/3 (i.e. body surface area) are at odds with those advocating a value of ¾.
Fig. 4.3 Paracetamol clearance maturation shown as the linear per kilogramme model (upper panel) and using the allometric ¾ power model (lower panel). Data are the same for each graph but clearance units differ. The upper panel using the linear per kilogramme model shows how clearance increases in the first few years of life and then decreases to reach adult rates in teenage years. The lower panel using allometric scaling shows that once clearance maturation matures over the first few years of life, the clearance is constant at all ages until elderly.
Support for a value of ¾ comes from investigations that show that the log of BMR plotted against the log of body weight produces a straight line with a slope of ¾ in all species studied, including humans. Fractal geometry is used to mathematically explain this phenomenon. The ¾ power law for metabolic rates was derived from a general model that describes how essential materials are transported through space-filled fractal networks of branching tubes [15]. A great many physiological, structural and time related variables scale predictably within and between species with weight (W) exponents (PWR) of ¾, 1 and ¼ respectively [16].
These exponents have applicability to PK parameters such as clearance (CL exponent of ¾), volume (V exponent of 1) and half-time (T1/2 exponent of ¼) [16]. The factor for size (Fsize) for total drug clearance may be expressed as:

Clearance is a metabolic function, and while the process for drug clearance may differ between animals, the clearance of tramadol plotted against the log of body weight produces a straight line with a slope of ¾ in all species studied (Fig. 4.4) [17].
Fig. 4.4 Weight-predicted tramadol total clearance (CLP total) compared to human allometric prediction using a ¾ power exponent (solid line). Clearance can be scaled using allometric theory over four orders of magnitude.
Maintenance dose is determined by clearance. The difference in drug clearance between an adult and a child is predictable from weight using theory-based allometry:

Allometric theory predicts maintenance dose per kg is higher in children. For example, remifentanil clearance is increased in neonates, infants and children when expressed per kilogramme [18]. However, remifentanil clearance in children aged 1 month–9 years is similar to adult rates when scaled using an allometric exponent of 3/4 [19]. Non-specific blood esterases that metabolize remifentanil are mature at birth [20], and that is when clearance (expressed as per kilogramme) is highest (see Fig. 4.5).
Fig. 4.5 The effect of age on the dose of remifentanil (x) tolerated during spontaneous ventilation under anaesthesia in children undergoing strabismus surgery. Superimposed on this plot is estimated remifentanil clearance (solid curved line) determined using an allometric model. There is a mismatch between clearance and infusion rate for those individuals still in infancy. The higher infusion rates recorded in those infants can be attributed to greater suppression of respiratory drive in this age group than the older children during the study; a respiratory rate of ten breaths per minute in an infant is disproportionately slow compared to the same rate in a 7-year-old child, suggesting excessive dose.
Population analyses of drugs in children using allometric models for size report that total body weight contributes more than 50% of clearance variability, e.g. for dexmedetomidine 86% [21], acetaminophen 77% [22], ketamine 54% [23] and levobupivacaine 62% [24]. The contribution that size makes is dependent on the subgroup of the paediatric population studied. For example, weight only contributed 57% of acetaminophen clearance variability in a neonatal population. The contribution of size to clearance variability is even less for other drugs in the neonatal period, e.g. tramadol 38% [25], aminoglycosides 47% [26]. The weight range in the neonatal population is smaller (e.g. 0.5–5 kg) than in the entire paediatric population (e.g. 0.5–100 kg) and we might anticipate age to have greater additional impact in the first few years of life because it reflects maturation.
Maturation
Unlike for remifentanil clearance, allometry alone is insufficient to predict clearance in neonates and infants from adult estimates for most drugs [27, 28]. The addition of a model describing maturation is required [29]. The sigmoid hyperbolic or Hill model [30] has been found useful for describing this maturation factor (MF):

The TM50 describes the maturation half-time, while the Hill coefficient relates to the slope of this maturation profile. Maturation of clearance begins before birth, suggesting that PMA would be a better predictor of drug elimination than postnatal age (PNA) [16]. Figure 4.6 shows the maturation profile for dexmedetomidine expressed as both the standard per kilogramme model and using allometry. Clearance is immature in infancy. Clearance is maximal at 2 years of age when expressed using the linear per kilogramme model, decreasing subsequently with age. This ‘artefact of size’ disappears with use of the allometric model.
Fig. 4.6 The clearance maturation profile of dexmedetomidine expressed using the per kilogramme model and the allometric ¾-power model. The profiles are similar to those observed for paracetamol (Fig. 4.3). These maturation patterns are typical of many drugs cleared by the liver or kidneys.Clearance is highest in infants when expressed as the linear per kilogramme model. However, use of allometric theory reveals that clearance matures sometime in the first year or so of life and then plateaus.
The impact of PMA will depend on the speed of maturation and the subpopulation studied; age will contribute more to variability of drugs given to neonates where clearance pathways mature rapidly in the first months of life (e.g. fentanyl CYP3A4) [31] or tramadol (CYP2D6, CYP3A) [25] than those that mature more slowly (acetaminophen, UGT1A6) [9]. PMA only contributed 12% to clearance variability in an acetaminophen neonatal study [32], but 27% of tramadol clearance variability in neonates [25]. Consequently, size, described using weight, is the single most important covariate for determining acetaminophen clearance in neonates during the initial slow maturation phase (<44 weeks PMA). Age has a greater contribution after this phase so that both age and size contribute 91% of variance of clearance throughout the paediatric age span [33].
Organ Function
Changes associated with normal growth and development can be distinguished from pathological changes describing organ function (OF) [4]. Morphine clearance is reduced in neonates because of immature glucuronide conjugation, but clearance was lower in critically ill neonates than healthier cohorts [34–36], possibly attributable to reduced hepatic function. The impact of organ function alteration may be concealed by another covariate. For example, positive pressure ventilation may be associated with reduced clearance for perfusion limited drugs (e.g. propofol, morphine); reduced hepatic blood flow due to artificial ventilation may be the cause of reduced clearance rather than being critically ill.
Creatinine clearance is commonly used as a measure of renal function and dictates dose of those drugs cleared by that organ. Difficulties arise determining renal function in children. Neonatal creatinine reflects that of maternal function in the first few days of life; creatinine production is reduced because of less muscle bulk. Classic methods of creatinine clearance estimation require, in addition to toilet training, complete bladder emptying. Radioisotopes are not ideal in children for repeat studies [37]. A number of formulae have been published that allow estimation of glomerular filtration rate (GFR) from clinical characteristics [38]. These formulae use simple markers such as height, creatinine concentration in plasma and body surface area (BSA). Estimation of GFR is acceptable in adults, but prediction is poor in children with a GFR value less than 40 mL/min [39]. We might expect the maturation of creatinine clearance, a marker for GFR, to reflect the influences of size, maturation and organ function. Estimation methods such as those of Schwartz incorporate a size factor (body length or height) and a scaling factor (k) that is age dependent as follows: k=0.33 in premature neonates; k=0.45 in term infants 0–1 y; k=0.45 in 1–12-y-old; and k=0.7 in 13–21-y-old adolescent males [40–42]. This variable is entered in the following formula:

Creatinine clearance estimation over-predicts GFR in children, possibly because of tubular secretion. In premature neonates tubular reabsorption may also create inaccuracies. Dose of renally cleared drugs in premature neonates should be based on size and maturation-based predictions of GFR alone and not use serum creatinine until creatinine production rate predictions in this age group are better established. A description of GFR maturation from data that ranged from premature neonates (22 weeks PMA) to adulthood (31 years) using an Emax model has been published [13].
Modelling Major Pharmacokinetic Covariates in Children
Pharmacokinetic parameters (P) can be described in an individual as the product of size (Fsize), maturation (MF) and organ function (OF) influences where Pstd is the value in a standard size adult without pathological changes in organ function [4]:
This methodology is increasingly used to describe clearance changes with age [29, 43]. An understanding of these principles can be used to predict dose in children using target concentration methodology [5].
Major Adult Pharmacokinetic Covariates
Weight and age are the most relevant covariates used to adjust anaesthetic doses in adult patients (e.g. [44, 45]). Per-kilogramme dosing guidelines are commonly appropriate for normal weight adult subjects. In the obese, however, linear per-kilogramme schemes using total body weight derived from lean patients can lead to overdose and adverse effects [46–48]. Obesity is highly prevalent in adult patients and will be treated separately in a later section of this chapter. Age, organ function, sex, ethnicity and comorbidity also influence the PK and PD of commonly used anaesthetic drugs.
Age
The average age of the world’s population is increasing rapidly and a higher proportion of patients presenting for surgery in the future will be older [49]. Ageing processes are linked mechanistically to body composition changes, decreases in physiological reserve and altered PD responses. These changes are progressive and might affect organ and tissue function. However, much of the age-related decline in OF is secondary to disease rather than normal ageing, since an increased likelihood of underlying comorbidities and polypharmacy are strongly associated with ageing. These characteristics make older patients a very heterogeneous group and a challenging population. In general, drug requirements decrease with ageing due to PK and PD changes.
Ageing is associated with an increase in fat mass (20–40%) and a decrease in body water (10–15%) that may cause an increased plasma concentration of water-soluble drugs and a longer elimination half-life for lipid-soluble drugs. Changes in protein binding with age (e.g. a decrease in albumin and an increase in alpha-1 glycoprotein) may lead to an altered unbound fraction of drugs in the elderly with possible changes in their volumes of distribution and clearances.
Ageing is also commonly associated with a reduction in clearance of drugs. Hepatic clearance is influenced primarily by hepatic blood flow, intrinsic clearance (enzyme activity and liver mass) and protein binding [50]. Liver mass and blood flow decrease by approximately 30–40% in elderly subjects [50, 51]. Similarly, reduced metabolism of drugs can also be associated with ageing. Renal function is progressively reduced in older people. Chronic diseases such as hypertension and diabetes, which are highly prevalent in older subjects, contribute to renal failure.
Ageing is associated with changes that lead to a decrease in cardiovascular reserve. Some of these changes include: conduction system fibrosis, loss of atrial node cells, increased stiffening of connective tissues, myocardial hypertrophy, stiff arteries and veins, decreased response to beta-receptor stimulation and an increase in sympathetic tone at rest. Older people are more susceptible to adverse drug effects and cardiovascular instability [50].
In adult patients, age has also been shown to be an important covariate for anaesthetic drugs. Although it is recognized that biological age is a better covariate than chronological age, most use chronological age as a covariate. For example, age effect in remifentanil pharmacokinetics described by a three-compartment model was represented by a decrease in V1 by approximately 25% and CL1 by 33% from the ages of 20 to 85 years [52]. Propofol elimination clearance decreased linearly in patients older than 60 years; as did V1 similarly [53]. A propofol analysis found that all the model parameters, with the exception of V3, appear to decrease with increasing age [54]. Similarly, paracetamol clearance decreases with increasing age, with changes most marked in frail older patients.
Age as a covariate has been investigated as either a discrete or continuous variable. The simplest method is to simply add an additional factor (FAGE) to the mathematical formula for those patients older than a certain age (e.g. 70 years), but a continuous variable may be more appropriate because changes rarely suddenly begin at a specified age:
Where SLOPE is a parameter describing changes about a median age (e.g. 50 years). The use of an exponential function avoids a negative FAGE:
This FAGE can then be used as a covariate for the estimation of the PK parameter (P, e.g. CL or V) as in Equation 6.
Organ Dysfunction
Organ dysfunction is an important covariate in adults. Renal, hepatic and cardiac function can all impact on drug clearance. Collinearity between organ function and age or disease is common and so reduced function may be marked by a surrogate e.g. age. While hepatic and renal disease and their impact on clearance are well documented, cardiac function may have more subtle effects, impacting on initial volume of distribution [55] or plasma to effect-site equilibration rate (T1/2keo) [55–57] and clearances (particularly for those drugs that are perfusion limited) [54, 57]. For example, a reduction in mean arterial blood pressure is associated with propofol PK alterations that increase the blood propofol concentration [58].
Liver Function
The liver is the most important organ involved in biotransformation and elimination of drugs [59]. Liver failure may alter absorption, distribution, metabolization and elimination (ADME) processes. A sensitive marker of liver function that could be used to characterize PK effects is not available. Hepatic dysfunction using clinical scoring systems (e.g. Child–Pugh or MELD classification) may be hard to quantify and relate to altered drug disposition because the impact of dysfunction is multipronged: alteration in hepatic blood flow, reduced hepatocellular mass, reduced synthetic ability, reduced plasma protein concentrations and binding, and reduced clearance for some pathways. Each patient may have an individual pattern of dysfunction and different drugs are affected differently depending on their clearance mechanism [60]. Clearance of dexmedetomidine, a highly selective α-2 adrenergic agonist that is extensively metabolized in the liver [61, 62], decreased by 33% with obstructive jaundice [63]. In contrast, cirrhotic patients had a similar propofol clearance but a greater volume of distribution than healthy patients [64].
Hepatic elimination of drugs (CLH) depends on liver blood flow (QH), intrinsic clearance (CLint) and the fraction of unbound drugs in the blood (fu) [59]:

The efficiency of the liver to eliminate a drug from the circulation is expressed by the hepatic extraction ratio (EH) as high (EH>0.7), intermediate (0.3<EH<0.7) or low (EH<0.3). Hepatic elimination of highly extracted drugs (perfusion limited clearance) is affected by changes in liver blood flow, and is relatively insensitive to changes in plasma protein binding or enzymatic activity, whereas poorly extracted drugs are mostly influenced by plasma protein binding and enzyme activity (capacity limited clearance). Drugs with high capacity clearance pathways (e.g. glucuronidation) are less affected by hepatic dysfunction than those with capacity limited clearance. Clearance estimation of drugs that are highly bound to albumin or alpha1-acid glycoprotein in patients with severe liver failure should always account for the potential increase in the unbound fraction (fu) to correctly interpret clearance estimations, since a decrease in plasma protein binding is frequently observed in chronic hepatic disease. A potential decrease in the liver metabolic capacity might be masked by an increase in fu if only total plasma clearance is considered [59].
Renal Function
Renal dysfunction impacts on both those drugs dependent on the kidney for clearance and unmeta-bolites that may be cleared by the renal system [65]. Morphine, for example, is metabolized predominantly by hepatic glucuronide conjugation, but the water soluble active metabolites (morphine 3-glucuronide, morphine 6-glucuronide) are cleared by the kidneys [66]. Accumulation of the active metabolite, morphine-3-gluronide, in patients with renal failure can cause respiratory depression [67].
Renal clearance of drugs is determined by glomerular filtration, tubular secretion and tubular reabsorption. GFR has been shown to be a sensitive marker to assess kidney function and is widely used to adjust dose in patients with renal compromise. There are several methods to measure or estimate GFR [68]. The Cockroft–Gault equation can be used to estimate creatinine clearance (CrCL) and to guide dose in adult patients:

The constant is 1.23 for males and 1.04 for females. Additional constants can be added to account for race. Such adjustments simply allow for differences in creatinine production rate (CPR) between races and sexes. The standard adult CPR is 0.516 mmol/h in a 70 kg, 40-year-old male [49]. The increase of CPR with age in adolescents is assumed to be a consequence of increasing muscle bulk as opposed to the decrease in muscle bulk that occurs with older age in adults.
The volume of distribution of many drugs is increased in end stage renal disease due to fluid overload, decreased protein binding or altered tissue binding [68]. An increase in volume of distribution normally requires a larger loading dose to reach the target concentration more rapidly.
Drug Interactions
Drug interactions may involve either PK interactions, PD interactions or even both [69]. These are discussed in Chapter 3.
Some Additional Covariates
Disease Progression
Pathological states may resolve, worsen or remain static and the interpretation of the impact of medication on these changes can be complex [70]. Pain, for example, is not constant over time. It may wax and wane. A cyclic function has been used for interpreting fever response to ibuprofen in children [71], but not yet for pain response. Pain scores in children after tonsillectomy decrease after only three days, but are often worse on the second or third day [72–74]. Subsequent pain resolves over two weeks, and a variant of the Hill equation (Equation 4) has been used to model this resolution [75].
Assessment of the impact of any analgesic intervention in children should take into account changes with pain over time. Functions describing these changes have been incorporated into disease progression models. Disease status (or pain) usually has a baseline (S0) from which it decreases over time. A simple model to describe the natural history of pain could be a linear decrease with time, where α describes the slope of pain decrease, e.g.
Response to treatment is then a sum of the natural history of the pain resolution and the drug effect:
Placebo and Nocebo
Placebo and nocebo effects may be transient yet substantial, and few reports of pharmacological effect acknowledge their impact. An attempt to determine paracetamol analgesic effect in children after tonsillectomy, in which placebo was unaccounted for, overestimated the drug’s analgesic effect [76]. An additional function describing placebo effects was required in order to define more clearly the analgesic effect from medication [75]. The placebo effect can be difficult to quantify in a clinical study. It may be present in paediatric studies in almost all children studied, but quantification of this effect is greatly improved if some children are given a placebo drug [75]. Use of placebo in children can be difficult to justify ethically [77].
Placebo mechanisms can affect therapeutic outcomes and potentially be exploited clinically to improve clinical outcomes in paediatric populations [78]. Placebo effect is rarely considered in paediatric analgesic studies [79], even though placebo responses might be more pronounced in children than in adults [80]. Certainly, the placebo effect accounted for a mean pain reduction of 5.6 units (VAS, 0–10) at three hours in a study investigating paracetamol analgesia after tonsillectomy [75]. This placebo effect is greater than that described in adults given naproxen analgesia following wisdom tooth extraction. Those adults only reported a maximum reduction in baseline pain attributable to placebo of 20% [81].
The placebo response in the paediatric study reflected a combination of placebo effect, natural pain resolution, behavioural pain coping mechanisms and our inability to discriminate pain from emergence phenomena in the early postoperative period [82]. Following emergence from anaesthesia, children were reunited with family, given access to movies and allowed flavoured ice. These are all important contributors to the relief of pain that was described as the placebo response. This response can be augmented by verbally induced expectations, conditioning and learning mechanisms, and the child–parent–physician interactions [78].
The same factors that may be used to augment the placebo response can also induce a nocebo response. The nocebo effect is the development of aversive effects also following any treatment or situation that normally has no therapeutic effect for the treated condition [83]. Virtually all treatment, pharmacological or not, will carry a placebo or nocebo effect related to the conditioned response to a similar situation and the expectation of the patient [84]. There are currently few studies in children that monitor nocebo effect, although the nocebo effect in epilepsy treatment in children [85] and in adult patients suffering undergoing interventional pain management has been reported [86]. Differentiating this placebo or nocebo response from drug effect can be difficult. Ethical considerations often preclude a group of children randomized to receive a placebo drug [77]. The continuing use of placebo controls has been strongly argued against in the literature [77, 87].
Functions that have been used to quantify the placebo effect include:
where t is time, PMAX is the maximum placebo effect and kpl is the rate constant for onset of placebo effect.
where PMAX is the maximum placebo effect and kon and koff are the rate constants for onset and offset of placebo effect, respectively.

where PMAX is the maximum placebo effect and λ and k are the scale and shape parameters of the Weibull function.
The shape of the curve described by these functions depends on the variables estimated for each function. Some typical examples of such curves for placebo effects are shown in Fig. 4.7. Nocebo effects could be described by the inverse of these curves.
Fig. 4.7 Examples of three functions (exponential decay, Bateman and Weibull) that could be used to describe placebo effect for pain relief. The y-axis uses a visual analogue scale (VAS) where a score of 10 is the worst pain imaginable. The Bateman function demonstrates a placebo effect that wears off with time. Nocebo effects can be imagined as the inverse of these curves.
Consequently, the observed effect is further complicated by placebo (or nocebo):
Drop Outs
Patients who receive rescue medication and are withdrawn from a study may introduce bias because the remaining study patients are those who do not have such severe pain; the reason for their reduced analgesia may not be solely pharmaceutical [88]. Failure to account for drop out in pain studies means that we consider all study attrition to be random, when in fact attrition usually occurs because of some factor (such as inadequate pain control or unpleasant drug effects) and may not be equally distributed among study groups. The rate of loss of study subjects (i.e. drop outs) can be explained as a hazard (h), with contributing factors explaining attrition included. For example, the time to request of rescue medication can be described using a time-to-event model where the hazard of requesting rescue medication is modelled as:
where h(t) is the hazard at time t, h0 is the hazard without influence of covariates, and COV is the influence of covariates on the hazard. The hazard without influence of covariates (h0) can be described using different hazard distributions such as the exponential, Weibull and Gompertz functions [89].
Introduction of a hazard model in an investigation of combined paracetamol and diclofenac analgesia after tonsillectomy helped to correct bias in model predictions of pain score introduced by study drop out. Those patients with severe pain, for example, were more likely to drop out of the study [90].
Coexisting Morbidity
Patients commonly present for anaesthesia with conditions that require investigation or surgical intervention; they also present with diseases unrelated to the need for surgery. The impact of disease on therapy (and vice versa) has been known for centuries. Pythagoras (510 BC) observed haemolytic anaemia after fava bean ingestion and this observation was later attributed to glucose-6-phosphate dehydrogenase (G-6-PD) deficiency only last century.
Disorders affect all ages. In the very young, congenital malformations (e.g. duodenal atresia) or disease characteristics (e.g. necrotizing enterocolitis) contribute to absorption variability. Perinatal circulatory changes (e.g. ductus venosus, ductus arteriosus) alter drug disposition [91]. Induction of anaesthesia may be slowed by right-to-left shunting of blood in neonates suffering cyanotic congenital cardiac disease or intrapulmonary conditions.
The environment in which children live can have subtle effects. Opioid administration was reduced in otherwise healthy children with altitude-induced chronic hypoxia when compared to non-hypoxic children undergoing similar operations under similar anaesthetic regimens [92]. Similar findings have been reported in obese children suffering obstructive sleep apnoea who have increased sensitivity to opioids [93]. Opioid sensitivity may be affected in disease states because there are specific transport systems that mediate active transport. Pathological central nervous system (CNS) conditions can cause blood–brain barrier (BBB) breakdown and alter functioning of its transport systems. Fentanyl is actively transported across the BBB by a saturable ATP-dependent process, while ATP-binding cassette proteins such as P-glycoprotein actively pump out opioids such as fentanyl and morphine [94]. P-glycoprotein modulation significantly influences opioid brain distribution and onset time, magnitude and duration of analgesic response [95]. Modulation may occur due to disease processes, fever or other substances (e.g. verapamil, magnesium) [94].
The influence of coexisting morbidity on the PK effect of anaesthetic drugs in adult patients is well recognized. For example ASA III patients had a smaller central volume of distribution and a reduced clearance for propofol compared to ASA I patients [96]. Similarly, in a pooled analysis of 21 propofol datasets, patients were found to have decreased propofol elimination and distribution clearances (CL, Q2, Q3) using a three compartment model, and slow peripheral volume of distribution (V3) compared with healthy volunteers [54]. The use of such empirical models describes an observed difference between patients and healthy volunteers; the cause is uncertain and might be related to concomitant medication, comorbidity or the anaesthetic technique applied. Another study in critically ill patients showed that fentanyl PK was strongly influenced by severe liver disease and congestive heart failure [97]. In that same study severity of illness as a covariate did not improve the model fit after liver and heart diseases were included. The expected PK and PD alterations of coexisting morbidity should consider not only the severity of the disease and its pathophysiological implications, but the possible effect of drug interactions from concomitant medications used.
Circadian Rhythms
Drugs can show time-of-day variation in their effect, and some are dosed according to a circadian schedule [98]. This effect can be due to either PK or PD changes over the day since the circadian system influences multiple biochemical and physiological variables which include changes in body temperature, hormone secretion, metabolism and our immune systems [99]. Local anaesthetic action is longest during the afternoon [100–102] and neuromuscular blockade by rocuronium lasts one-third longer in the morning compared to the afternoon [103]. Chronotherapeutic dosing of 5-fluorouracil, with peak delivery at 0400 h, is more effective and less toxic than when administered as a constant infusion [104]. Both absorption and bioavailability differences at different times of the day have been described for paracetamol [105], indomethacin [106, 107], diclofenac [108] and ketoprofen [109] in adults.
The Burgeoning Obesity Problem
Volume (determining loading dose) and clearance (determining maintenance dose) of some drugs are known to be changed in obesity [110]. Although body fat has minimal metabolic activity, fat mass contributes to overall body size and may have an indirect influence on both metabolic and renal clearance from the secondary increase in lean tissues needed to support the increase in fat weight. In addition, an increase in abdominal visceral fat can produce an excessive secretion of hormones and inflammatory bio-active peptides, with a variety of potential effects in organ systems and circulation. On the other hand, the volume of distribution of a drug depends on its physicochemical properties [111]. There are drugs whose apparent volume of distribution may be independent of fat mass (e.g. digoxin) or be extensively determined by it (e.g. diazepam).
A number of size descriptors (Fig. 4.8) have been put forward for use in the obese patient e.g. total body weight (TBW), lean body weight (LBW), ideal body weight (IBW), body mass index (BMI), fat free mass (FFM) and normal fat mass (NFM). These size descriptors invariably demonstrate nonlinear relationships between weight and clearance. The best size descriptor accounting for obesity remains unknown [112]. LBW is often advocated for use in the obese, but that descriptor may not apply for all drugs [113, 114]. For example, for propofol, commonly used to maintain anaesthesia in the paediatric patient, the infusion rate is dependent on clearance. An incorrect estimate of clearance may lead to inadequate anaesthesia, and awareness. Propofol clearance in obese children [115] and adults, [54, 116] and in non-obese adults and children [53, 117], is best predicted using TBW as the size descriptor with theory-based allometry. But for remifentanil, another commonly used drug, lean body weight appears to be the better size descriptor [45, 48].
Fig. 4.8 Body size metrics used to describe clearance changes with weight for individuals of average height for weight. The clearance scale factor shows how clearance would differ with weight. A nonlinear relationship exists between weight and clearance using theory-based allometry. The per kg method increasingly overestimates clearance in adults and underestimates clearance in children. The BSA method overestimates clearance in children compared to theory-based allometry. Scaling with fat free mass (FFM) lies between the per kg method and theory-based allometry. An additional function is required to describe maturation (not shown).
The use of NFM [118] with allometric scaling as a size descriptor may prove versatile [13, 119–121]. That size descriptor uses the idea of FFM (which is similar to LBW but excludes lipids in cell membranes, CNS and bone marrow) plus a ‘bit more’. The ‘bit more’ will differ for each drug and the maximum ‘bit more’ added to fat free mass would equal TBW.

Where WHSmax is the maximum FFM for any given height (HT, m) and WHS50 is the TBW value when FFM is half of WHSmax. For men, WHSmax is 42.92 kg/m2 and WHS50 is 30.93 kg/m2 and for women WHSmax is 37.99 kg/m2 and WHS50 is 35.98 kg/m2 [12].
NFM is then defined as:
The parameter Ffat is estimated and accounts for different contributions of fat mass. If Ffat is estimated to be zero, then FFM alone predicts size, while if Ffat is 1, then size is predicted by TBW. This parameter is drug specific and also specific to the PK parameter such as clearance or volume of distribution. Ffat has a value of 0.211 for GFR which implies that 21% of fat mass is a size driver for kidney function in addition to FFM [13]. Size based on NFM assumes that FFM is the primary determinant of size with an extra Ffat factor (which may be positive or negative) that determines how fat mass contributes to size. A negative value for Ffat might suggest organ dysfunction, which is not an uncommon scenario in the morbidly obese. A negative Ffat has been described for the drug dexmedetomidine [122].
Pharmacodynamic Variability
Pharmacodynamic (concentration–effect) variability also exists. Receptor sensitivity variability (5–50%) and efficacy variability (30%) exist. Variability also exists due to distribution from the blood to the site of action and depends largely on changes in perfusion of target tissue (5–60%), the link between PK and PD. However, the observed response may not be a direct consequence of drug–receptor binding itself, but it may rather have been mediated through intermediate physiological mechanisms (e.g. antipyretics, angiotensin converting enzyme inhibitors). A typical value for this variability is 30% [123].
Age may also be a marker for PD changes. Age was a significant covariate of remifentanil EC50 and keo, with both decreasing with increasing age by approximately 50% over the age range studied [52]. Similar results are reported for propofol in the elderly patient. The EC50 values for loss of consciousness were 2.35, 1.8 and 1.25 μg/mL in volunteers of 25, 50 and 75 years old, respectively [124].
The minimum alveolar concentration (MAC) is commonly used to express anaesthetic vapour potency. The MAC values for most vapours in neonates are less than those in infants [125]. MAC increases with age in preschool children, and thereafter decreases again through childhood [126, 127]. The MAC of isoflurane in preterm neonates < 32 weeks’ gestation was 1.28% (SD 0.17), and in preterm neonates 32–37 weeks’ gestation was 1.41% (SD 0.18) which in turn is less than in full-term neonates [126]. Similar data are reported for halothane (Fig. 4.9) and sevoflurane [127–129].
Fig. 4.9 Halothane minimum alveolar concentration (MAC) changes with age. MAC is greatest in infancy.
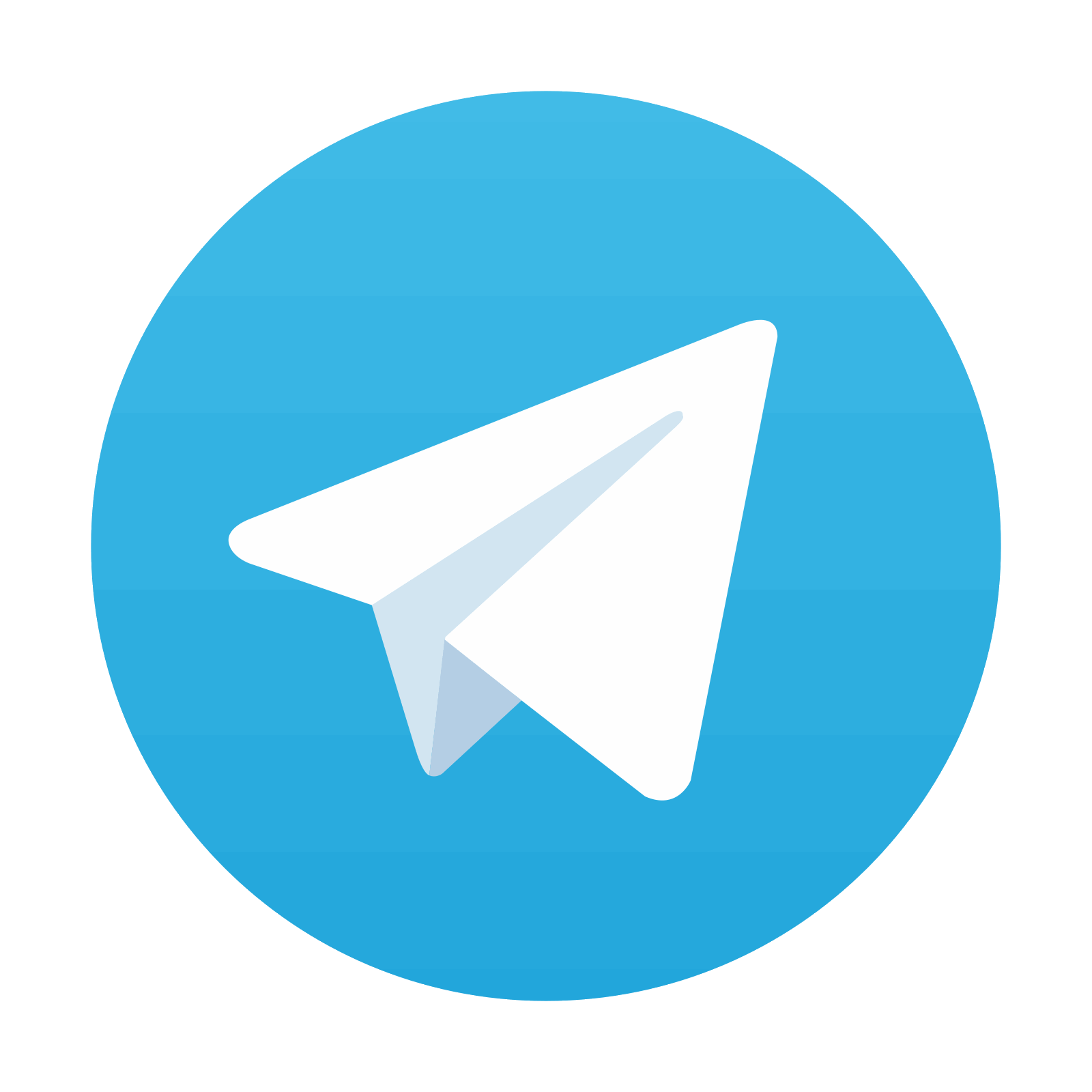
Stay updated, free articles. Join our Telegram channel

Full access? Get Clinical Tree
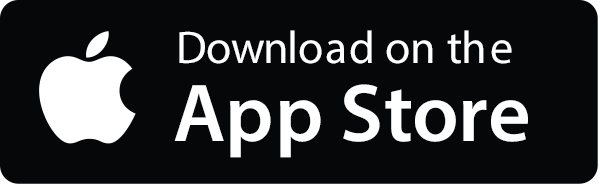

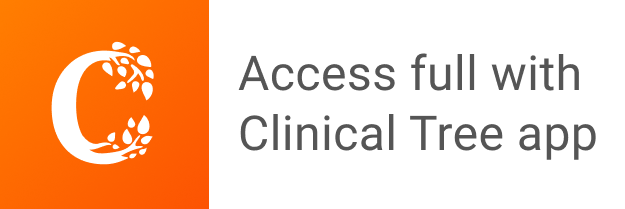